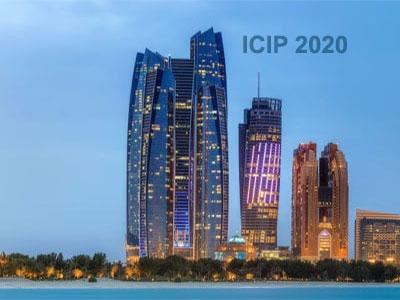
- Read more about Automatic Region Selection For Objective Sharpness Assessment of Mobile Device Photos
- 1 comment
- Log in to post comments
Mobile devices are the source of a vast majority of digital photos today. Photos taken by mobile devices generally have fairly good visual quality. When evaluating high-quality mobile device photos, people have to manually zoom in to local regions to discern the subtle difference. Understandably, a global objective quality assessment method cannot perform well on such task. Therefore, local region selection is widely recognized as a prerequisite for the following quality evaluation.
ICIPnow.pdf

- Categories:
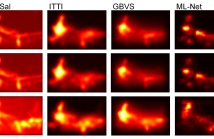
- Read more about DEEP LEARNING VS. TRADITIONAL ALGORITHMS FOR SALIENCY PREDICTION OF DISTORTED IMAGES
- Log in to post comments
Saliency has been widely studied in relation to image quality assessment (IQA). The optimal use of saliency in IQA metrics, however, is nontrivial and largely depends on whether saliency can be accurately predicted for images containing various distortions. Although tremendous progress has been made in saliency modelling, very little is known about whether and to what extent state-of-the-art methods are beneficial for saliency prediction of distorted images.
- Categories:
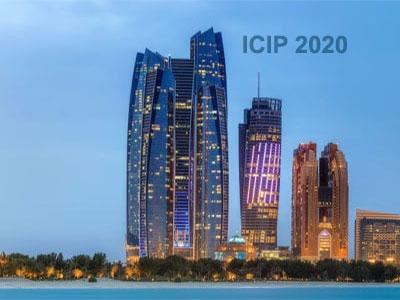
- Read more about Joint Demosaicking / Rectification of Fisheye Camera Images using Multi-color Graph Laplacian Regularization
- Log in to post comments
- Categories:
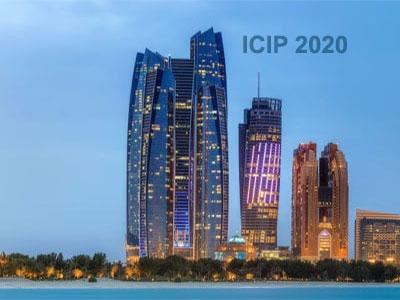
- Read more about Open-Set Metric Learning for Person Re-identification in The Wild
- Log in to post comments
Person re-identification in the wild needs to simultaneously (frame-wise) detect and re-identify persons and has wide utility in practical scenarios. However, such tasks come with an additional open-set re-ID challenge as all probe persons may not necessarily be present in the (frame-wise) dynamic gallery. Traditional or close-set re-ID systems are not equipped to handle such cases and raise several false alarms as a result. To cope with such challenges open-set metric learning (OSML), based on the concept of Large margin nearest neighbor (LMNN) approach, is proposed.
- Categories:
- Read more about DEPTH MAPS FAST SCALABLE COMPRESSION BASED ON CODING UNIT DEPTH
- Log in to post comments
- Categories:
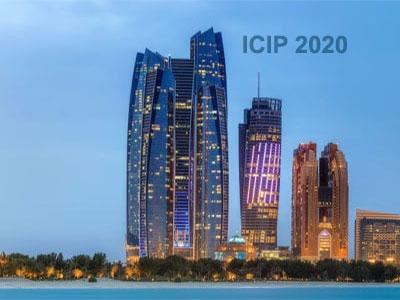
- Read more about 3D Point Cloud Enhancement using Graph-Modelled Multiview Depth Measurements
- Log in to post comments
A 3D point cloud is often synthesized from depth measurements collected by sensors at different viewpoints. The acquired measurements are typically both coarse in precision and corrupted by noise. To improve quality, previous works denoise a synthesized 3D point cloud a posteriori, after projecting the imperfect depth data onto the 3D space. Instead, we enhance depth measurements on the sensed images a priori, exploiting inherent 3D geometric correlation across views, before synthesizing a 3D point cloud from the improved measurements.
- Categories:
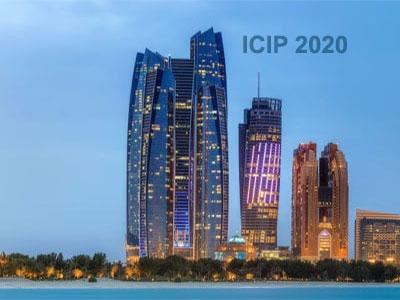
The multi-modality sensor fusion technique is an active research
area in scene understating. In this work, we explore
the RGB image and semantic-map fusion methods for depth
estimation. The LiDARs, Kinect, and TOF depth sensors are
unable to predict the depth-map at illuminate and monotonous
pattern surface. In this paper, we propose a semantic-to-depth
generative adversarial network (S2D-GAN) for depth estimation
from RGB image and its semantic-map. In the first stage,
the proposed S2D-GAN estimates the coarse level depthmap
- Categories:
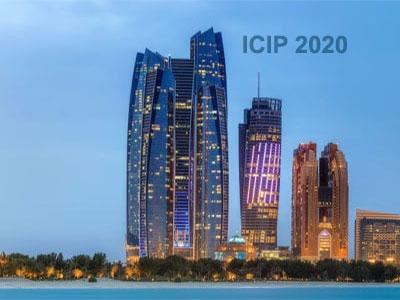
- Read more about Egok360: A 360 Egocentric Kinetic Human Activity Video Dataset
- Log in to post comments
Recently, there has been a growing interest in wearable sensors which provides new research perspectives for 360 ° video analysis. However, the lack of 360 ° datasets in literature hinders the research in this field. To bridge this gap, in this paper we propose a novel Egocentric (first-person) 360° Kinetic human activity video dataset (EgoK360). The EgoK360 dataset contains annotations of human activity with different sub-actions, e.g., activity Ping-Pong with four sub-actions which are pickup-ball, hit, bounce-ball and serve.
- Categories: