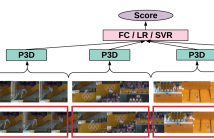
- Read more about S3D: Stacking Segmental P3D for Action Quality Assessment
- 1 comment
- Log in to post comments
Action quality assessment is crucial in areas of sports, surgery and assembly line where action skills can be evaluated. In this paper, we propose the Segment-based P3D-fused network S3D built-upon ED-TCN and push the performance on the UNLV-Dive dataset by a significant margin. We verify that segment-aware training performs better than full-video training which turns out to focus on the water spray. We show that temporal segmentation can be embedded with few efforts.
- Categories:
- Read more about VIDEO ENHANCEMENT WITH CONVEX OPTIMIZATION METHODS
- Log in to post comments
Video enhancement methods enable to optimize the viewing of video content at the end-user side. Most approaches do not consider the compressed nature of the available content. In the present work, we build upon a recently proposed video enhancement approach that explicitly models a compression stage. To apply the enhancement framework on compressed representations requires to extract specific syntax elements during their decoding. This additional information embeds the enhanced result in a domain that closely fits the observation.
- Categories: