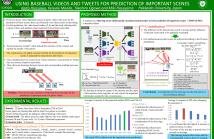
- Read more about TIME-LAG AWARE MULTI-MODAL VARIATIONAL AUTOENCODER USING BASEBALL VIDEOS AND TWEETS FOR PREDICTION OF IMPORTANT SCENES
- Log in to post comments
A novel method based on time-lag aware multi-modal variational autoencoder for prediction of important scenes (Tl-MVAE-PIS) using baseball videos and tweets posted on Twitter is presented in this paper. This paper has the following two technical contributions. First, to effectively use heterogeneous data for the prediction of important scenes, we transform textual, visual and audio features obtained from tweets and videos to the latent features. Then Tl-MVAE-PIS can flexibly express the relationships between them in the constructed latent space.
- Categories:
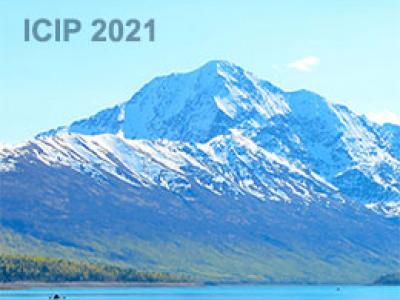
- Read more about TIME-LAG AWARE MULTI-MODAL VARIATIONAL AUTOENCODER USING BASEBALL VIDEOS AND TWEETS FOR PREDICTION OF IMPORTANT SCENES
- Log in to post comments
A novel method based on time-lag aware multi-modal variational autoencoder for prediction of important scenes (Tl-MVAE-PIS) using baseball videos and tweets posted on Twitter is presented in this paper. This paper has the following two technical contributions. First, to effectively use heterogeneous data for the prediction of important scenes, we transform textual, visual and audio features obtained from tweets and videos to the latent features. Then Tl-MVAE-PIS can flexibly express the relationships between them in the constructed latent space.
- Categories:
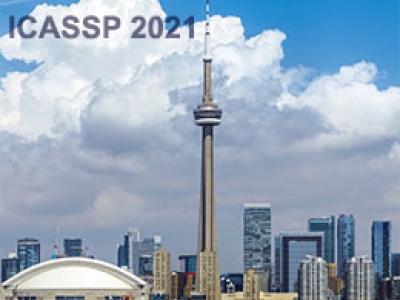
In this work, we present a hybrid CTC/Attention model based on a modified ResNet-18 and Convolution-augmented transformer (Conformer), that can be trained in an end-to-end manner. In particular, the audio and visual encoders learn to extract features directly from raw pixels and audio waveforms, respectively, which are then fed to conformers and then fusion takes place via a Multi-Layer Percep- tron (MLP). The model learns to recognise characters using a com- bination of CTC and an attention mechanism.
- Categories:
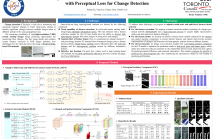
- Read more about An Adaptive Multi-Scale and Multi-Level Features Fusion Network with Perceptual Loss for Change Detection
- Log in to post comments
Change detection plays a vital role in monitoring and analyzing temporal changes in Earth observation tasks. This paper proposes a novel adaptive multi-scale and multi-level features fusion network for change detection in very-high-resolution bi-temporal remote sensing images. The proposed approach has three advantages. Firstly, it excels in abstracting high-level representations empowered by a highly effective feature extraction module.
MFPNet_poster.pdf

- Categories:
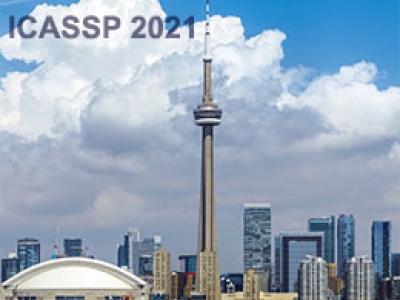
- Read more about ADAPTIVE RE-BALANCING NETWORK WITH GATE MECHANISM FOR LONG-TAILED VISUAL QUESTION ANSWERING
- Log in to post comments
- Categories:
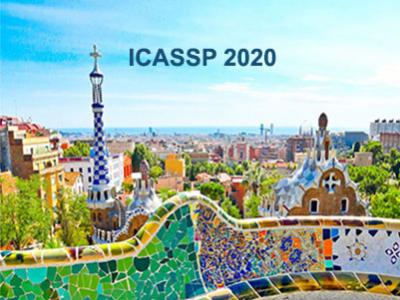
- Read more about Multi-Layer Content Interaction Through Quaternion Product for Visual Question Answering
- Log in to post comments
Multi-modality fusion technologies have greatly improved the performance of neural network-based Video Description/Caption, Visual Question Answering (VQA) and Audio Visual Scene-aware Di-alog (AVSD) over the recent years. Most previous approaches only explore the last layers of multiple layer feature fusion while omit-ting the importance of intermediate layers. To solve the issue for the intermediate layers, we propose an efficient Quaternion Block Net-work (QBN) to learn interaction not only for the last layer but also for all intermediate layers simultaneously.
- Categories:
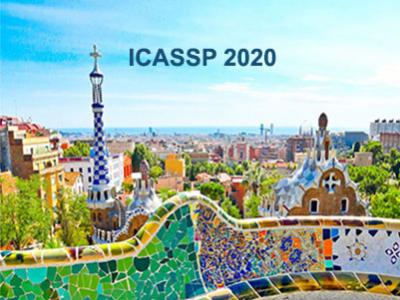
- Read more about WHAT MAKES THE SOUND?: A DUAL-MODALITY INTERACTING NETWORK FOR AUDIO-VISUAL EVENT LOCALIZATION
- Log in to post comments
The presence of auditory and visual senses enables humans to obtain a profound understanding of the real-world scenes. While audio and visual signals are capable of providing scene knowledge individually, the combination of both offers a better insight about the underlying event. In this paper, we address the problem of audio-visual event localization where the goal is to identify the presence of an event that is both audible and visible in a video, using fully or weakly supervised learning.
- Categories:
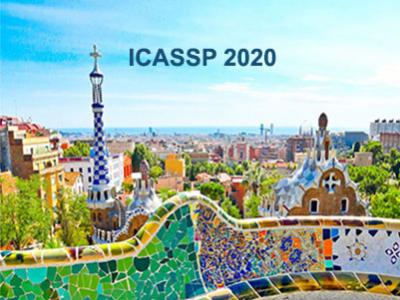
- Read more about Spectrogram Analysis Via Self-Attention for Realizing Cross-Model Visual-Audio Generation
- Log in to post comments
Human cognition is supported by the combination of multi- modal information from different sources of perception. The two most important modalities are visual and audio. Cross- modal visual-audio generation enables the synthesis of da- ta from one modality following the acquisition of data from another. This brings about the full experience that can only be achieved through the combination of the two. In this pa- per, the Self-Attention mechanism is applied to cross-modal visual-audio generation for the first time.
SA-CMGAN poster.pdf

- Categories:
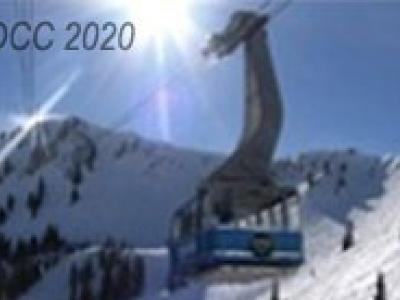
- Categories:
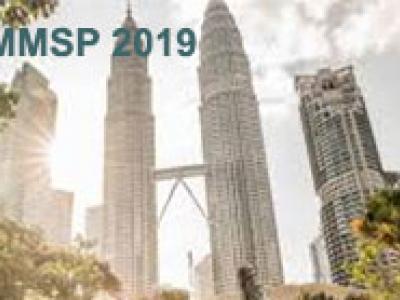
- Read more about Lightweight Deep Convolutional Neural Networks for Facial Epression Recognition
- Log in to post comments
- Categories: