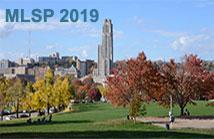
The 29th MLSP workshop in the series of workshops organized by the IEEE Signal Processing Society MLSP Technical Committee will take place at the University of Pittsburgh Campus, Pittsburgh, PA, USA and present the most recent and exciting advances in machine learning for signal processing through keynote talks, tutorials, special and regular single-track sessions as well as matchmaking events.
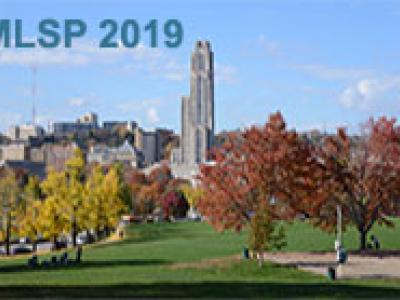
- Read more about Deep Learning for MRI Reconstruction Using a Novel Projection Based Cascaded Network
- Log in to post comments
After their triumph in various classification, recognition and segmentation problems, deep learning and convolutional networks are now making great strides in different inverse problems of imaging. Magnetic resonance image (MRI) reconstruction is an important imaging inverse problem, where deep learning methodologies are starting to make impact. In this work we will develop a new Convolutional Neural Network (CNN) based variant for MRI reconstruction. The developed algorithm is based on the recently proposed deep cascaded CNN (DC-CNN) structure.
- Categories:
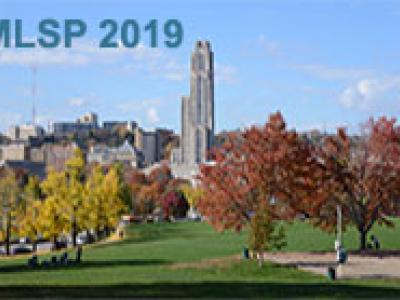
- Read more about Regularized state estimation and parameter learning via augmented Lagrangian Kalman smoother method
- Log in to post comments
In this article, we address the problem of estimating the state and learning of the parameters in a linear dynamic system with generalized $L_1$-regularization. Assuming a sparsity prior on the state, the joint state estimation and parameter learning problem is cast as an unconstrained optimization problem. However, when the dimensionality of state or parameters is large, memory requirements and computation of learning algorithms are generally prohibitive.
- Categories:
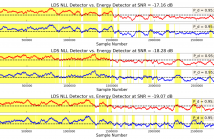
A machine learning approach to detecting unknown signals in time-correlated noise is presented. In the proposed approach, a linear dynamical system (LDS) model is trained to represent the background noise via expectation-maximization (EM). The negative log-likelihood (NLL) of test data under the learned background noise LDS is computed via the Kalman filter recursions, and an unknown signal is detected if the NLL exceeds a threshold. The proposed detection scheme is derived as a generalized likelihood ratio test (GLRT) for an unknown deterministic signal in LDS noise.
poster_mlsp.pdf

- Categories:
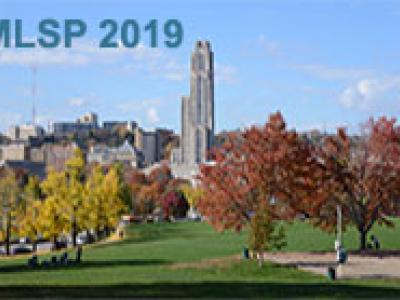
- Read more about Improving Neural Non-Maximum Suppression For Object Detection By Exploiting Interest-Point Detector
- Log in to post comments
Non-maximum suppression (NMS) is a post-processing step in almost every visual object detector. Its goal is to drastically prune the number of overlapping detected candidate regions-of-interest (ROIs) and replace them with a single, more spatially accurate detection. The default algorithm (Greedy NMS) is fairly simple and suffers from drawbacks, due to its need for manual tuning. Recently, NMS has been improved using deep neural networks that learn how to solve a spatial overlap-based detections rescoring task in a supervised manner, where only ROI coordinates are exploited as input.
- Categories: