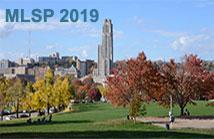
The 29th MLSP workshop in the series of workshops organized by the IEEE Signal Processing Society MLSP Technical Committee will take place at the University of Pittsburgh Campus, Pittsburgh, PA, USA and present the most recent and exciting advances in machine learning for signal processing through keynote talks, tutorials, special and regular single-track sessions as well as matchmaking events.
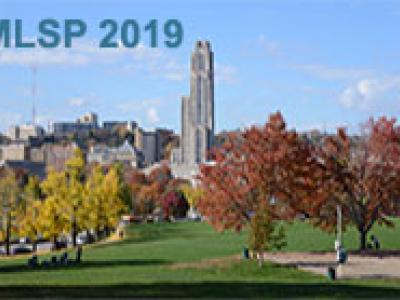
- Read more about EEG SIGNAL DIMENSIONALITY REDUCTION AND CLASSIFICATION USING TENSOR DECOMPOSITION AND DEEP CONVOLUTIONAL NEURAL NETWORKS
- Log in to post comments
- Categories:
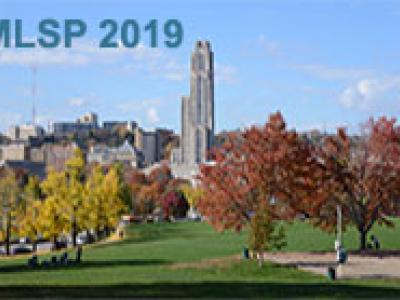
- Read more about Minimax Active Learning via Minimal Model Capacity
- Log in to post comments
Active learning is a form of machine learning which combines supervised learning and feedback to minimize the training set size, subject to low generalization errors. Since direct optimization of the generalization error is difficult, many heuristics have been developed which lack a firm theoretical foundation. In this paper, a new information theoretic criterion is proposed based on a minimax log-loss regret formulation of the active learning problem. In the first part of this paper, a Redundancy Capacity theorem for active learning is derived along with an optimal learner.
- Categories:
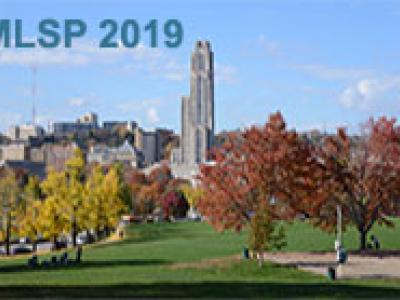
- Read more about Deep Reinforcement Learning Based Energy Beamforming for Powering Sensor Networks
- 1 comment
- Log in to post comments
We focus on a wireless sensor network powered with an energy beacon, where sensors send their measurements to the sink using the harvested energy. The aim of the system is to estimate an unknown signal over the area of interest as accurately as possible. We investigate optimal energy beamforming at the energy beacon and optimal transmit power allocation at the sensors under non-linear energy harvesting models. We use a deep reinforcement learning (RL) based approach where multi-layer neural networks are utilized.
- Categories:
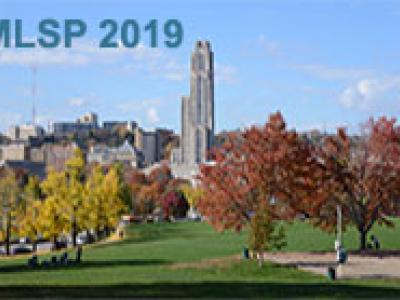
- Read more about AUDIO-VISUAL FUSION AND CONDITIONING WITH NEURAL NETWORKS FOR EVENT RECOGNITION
- Log in to post comments
Video event recognition based on audio and visual modalities is an open research problem. The mainstream literature on video event recognition focuses on the visual modality and does not take into account the relevant information present in the audio modality. We propose to study several fusion architectures for the audio-visual recognition task of video events. We first build classical fusion architectures using concatenation, addition or Multimodal Compact Bilinear pooling (MCB).
- Categories:
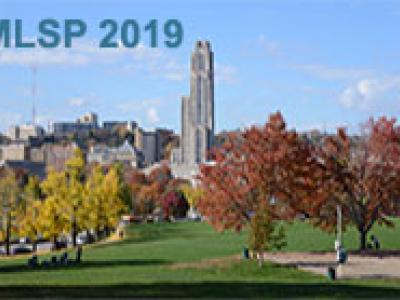
- Read more about Online Learning for Indoor Asset Detection
- Log in to post comments
Building floor plans with locations of safety, security, and energy assets such as IoT sensors, fire alarms, etc. are vital for climate control, emergency response, safety, and maintenance of building infrastructure. Existing approaches to building survey are tedious, error prone, and involve an operator with a clipboard and pen, enumerating and localizing assets in each room. We propose an interactive method for a human operator to use an app on a smartphone, which can detect, classify, and localize assets of interest, to expedite such a task.
- Categories:
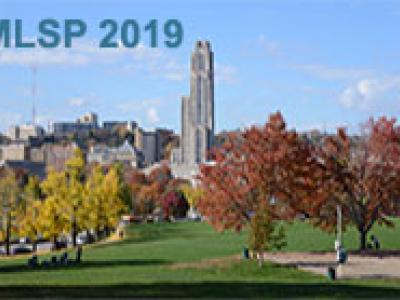
- Read more about ONLINE ANOMALY DETECTION IN MULTIVARIATE SETTINGS
- Log in to post comments
- Categories:
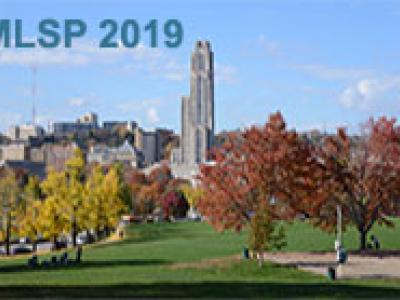
- Read more about DYNAMIC SYSTEM IDENTIFICATION FOR GUIDANCE OF STIMULATION PARAMETERS IN HAPTIC SIMULATION ENVIRONMENTS
- Log in to post comments
- Categories:
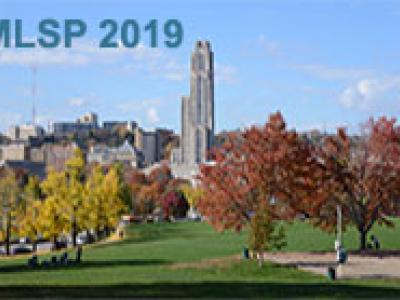
- Read more about ACCELERATING NONNEGATIVE MATRIX FACTORIZATION OVER POLYNOMIAL SIGNALS WITH FASTER PROJECTIONS
- Log in to post comments
- Categories:
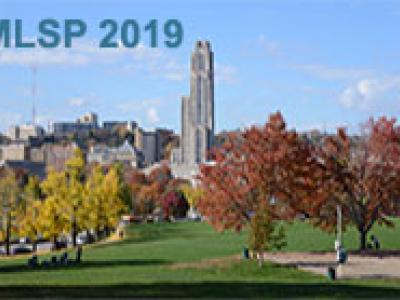
- Read more about Self-supervised representation learning from electroencephalography signals
- Log in to post comments
The supervised learning paradigm is limited by the cost - and sometimes the impracticality - of data collection and labeling in multiple domains. Self-supervised learning, a paradigm which exploits the structure of unlabeled data to create learning problems that can be solved with standard supervised approaches, has shown great promise as a pretraining or feature learning approach in fields like computer vision and time series processing. In this work, we present self-supervision strategies that can be used to learn informative representations from multivariate time series.
- Categories: