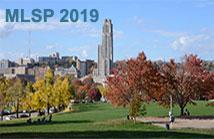
The 29th MLSP workshop in the series of workshops organized by the IEEE Signal Processing Society MLSP Technical Committee will take place at the University of Pittsburgh Campus, Pittsburgh, PA, USA and present the most recent and exciting advances in machine learning for signal processing through keynote talks, tutorials, special and regular single-track sessions as well as matchmaking events.
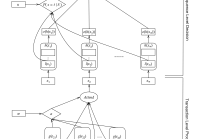
- Read more about Interpretable Online Banking Fraud Detection based on Hierarchical Attention Mechanism
- Log in to post comments
Online banking activities are constantly growing and are likely to become even more common as digital banking platforms evolve. One side effect of this trend is the rise in attempted fraud. However, there is very little work in the literature on online banking fraud detection. We propose an attention based architecture for classifying online banking transactions as either fraudulent or genuine. The proposed method allows transparency to its decision by identifying the most important transactions in the sequence and the most informative features in each transaction.
- Categories:
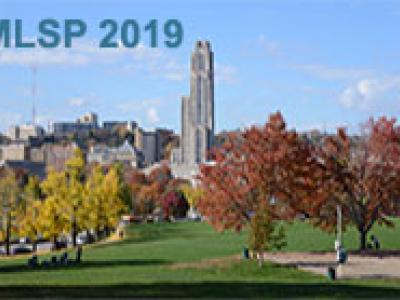
- Read more about INCREMENTAL LABEL PROPAGATION ON FACIAL IMAGES
- Log in to post comments
The increasing computational complexity of label propagation-based facial image annotation when applied on multimedia data whose cardinality increases over the time (e.g., when analyzing video or movie content on-line), can be reduced by using an incremental approach. In this paper, a method for incremental label propagation on facial images is described. The similarity matrix is incrementally constructed by employing the kd-tree nearest neighbor algorithm.
- Categories:
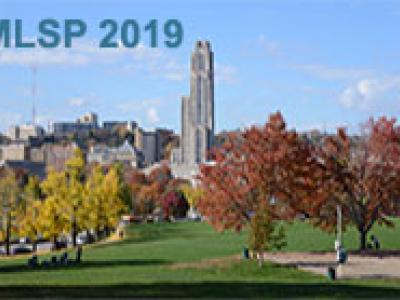
- Read more about AUTOMATIC SCREENING OF CHILDREN WITH SPEECH SOUND DISORDERS USING PARALINGUISTIC FEATURES
- Log in to post comments
MLSP (3).pdf

- Categories:
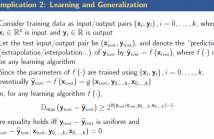
- Read more about Generic Bounds on the Maximum Deviations in Sequential/Sequence Prediction (and the Implications in Recursive Algorithms and Learning/Generalization)
- Log in to post comments
In this paper, we derive generic bounds on the maximum deviations in prediction errors for sequential prediction via an information-theoretic approach. The fundamental bounds are shown to depend only on the conditional entropy of the data point to be predicted given the previous data points. In the asymptotic case, the bounds are achieved if and only if the prediction error is white and uniformly distributed.
- Categories:
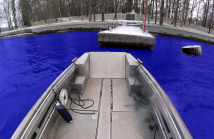
- Read more about High Resolution Water Segmentation for Autonomous Unmanned Surface Vehicles: A Novel Dataset and Evaluation
- Log in to post comments
Even though Unmanned Surface Vehicles (USVs) are increasingly used to perform various laborious and expensive off-shore tasks, they still require an extensive dedicated crew supporting and ensuring the safety of their operations. The recent developments in computer vision and robotics further fueled the interest on developing \textit{autonomous} USVs that will overcome the aforementioned limitations, unleashing their full potential. One of the most vital and fundamental tasks in order to automate and ensure the safety of USV operations is to perform water segmentation.
- Categories:
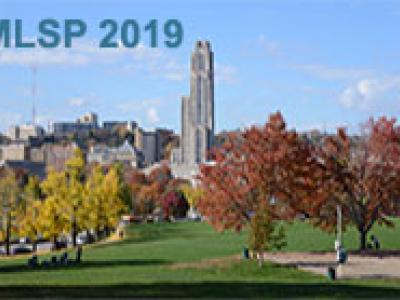
- Read more about Single-Pixel Camera Sensing Matrix Design For Hierarchical Compressed Spectral Clustering
- 1 comment
- Log in to post comments
Compressive spectral imaging (CSI) acquires random projections of a spectral scene. Typically, before applying any post-processing task, e.g. clustering, it is required a computationally expensive reconstruction of the underlying 3D scene. Therefore, several works focus on improving the reconstruction quality by adaptively designing the sensing matrix aiming at better post-processing results. Instead, this paper proposes a hierarchical adaptive approach to design a sensing matrix of the single-pixel camera, such that pixel clustering can be performed in the compressed domain.
- Categories:
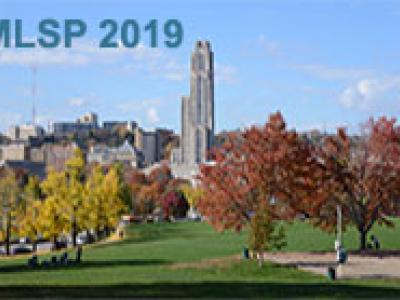
- Read more about EXTENDED VARIATIONAL INFERENCE FOR PROPAGATING UNCERTAINTY IN CONVOLUTIONAL NEURAL NETWORKS
- Log in to post comments
Model confidence or uncertainty is critical in autonomous systems as they directly tie to the safety and trustworthiness of
the system. The quantification of uncertainty in the output decisions of deep neural networks (DNNs) is a challenging
problem. The Bayesian framework enables the estimation of the predictive uncertainty by introducing probability distributions
over the (unknown) network weights; however, the propagation of these high-dimensional distributions through
- Categories:
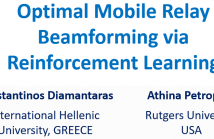
- Categories:
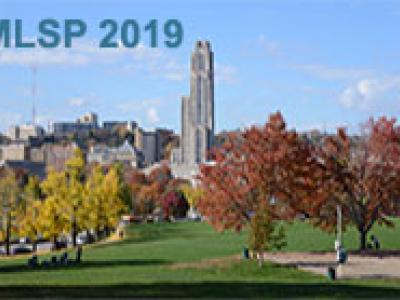
- Read more about A Bayesian Generative Model With Gaussian Process Priors For Thermomechanical Analysis Of Micro-Resonators
- Log in to post comments
Thermal analysis using resonating micro-electromechanical systems shows great promise in characterizing materials in the early stages of research. Through thermal cycles and actuation using a piezoelectric speaker, the resonant behaviour of a model drug, theophylline monohydrate, is measured across the surface whilst using a laser-Doppler vibrometer for readout. Acquired is a sequence of spectra that are strongly correlated in time, temperature and spatial location of the readout. Traditionally, each spectrum is analyzed individually to locate the resonance peak.
- Categories: