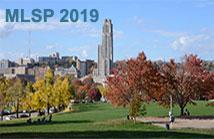
The 29th MLSP workshop in the series of workshops organized by the IEEE Signal Processing Society MLSP Technical Committee will take place at the University of Pittsburgh Campus, Pittsburgh, PA, USA and present the most recent and exciting advances in machine learning for signal processing through keynote talks, tutorials, special and regular single-track sessions as well as matchmaking events.
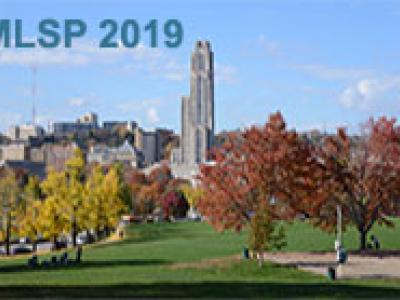
- Read more about Efficient Parameter Estimation for Semi-Continuous Data: An Application to Independent Component Analysis
- Log in to post comments
Semi-continuous data have a point mass at zero and are continuous with positive support. Such data arise naturally in several real-life situations like signals in a blind source separation problem, daily rainfall at a location, sales of durable goods among many others. Therefore, efficient estimation of the underlying probability density function is of significant interest.
MLSP_2019.pdf

- Categories:
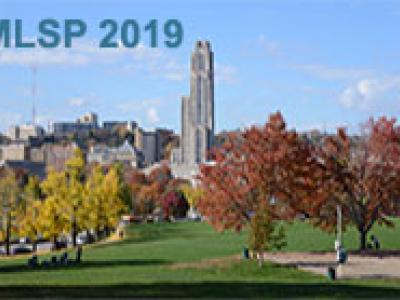
- Read more about Incorporating Intra-Spectral Dependencies With A Recurrent Output Layer For Improved Speech Enhancement
- Log in to post comments
Deep-learning based speech enhancement systems have offered tremendous gains, where the best performing approaches use long short-term memory (LSTM) recurrent neural networks (RNNs) to model temporal speech correlations. These models, however, do not consider the frequency-level correlations within a single time frame, as spectral dependencies along the frequency axis are often ignored. This results in inaccurate frequency responses that negatively affect perceptual quality and intelligibility. We propose a deep-learning approach that considers temporal and frequency-level dependencies.
- Categories:
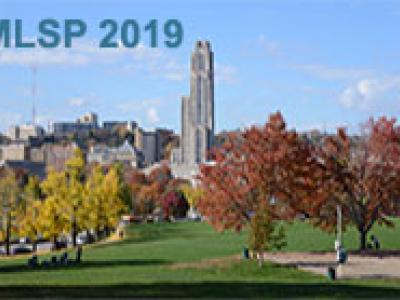
- Read more about COLLABORATIVE INFERENCE OF MISSING SMART ELECTRIC METER DATA FOR A BUILDING
- Log in to post comments
This paper proposes a novel approach to infer missing electricity meter data of a building using a seasonal autoregressive integrated moving average model with exogenous variables (SARIMAX). A cross-correlation function is utilized to identify buildings that have electricity usage patterns similar to the building with missing meter data.
- Categories:
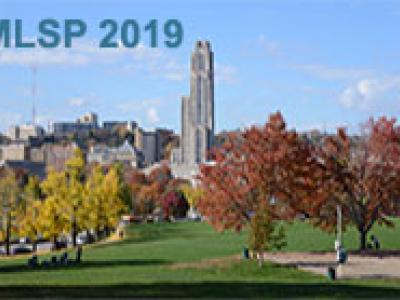
- Read more about HACD: Hierarchical Agglomerative Community Detection in Social Networks
- Log in to post comments
Communities (also referred to as clusters) are essential building blocks of all networks. Hierarchical clustering methods are common graph-based approaches for graph clustering. Traditional hierarchical clustering algorithms proceed in a bottom-up or top-down fashion to encode global information in the graph and cluster according to the global modularity of the graph.
- Categories:
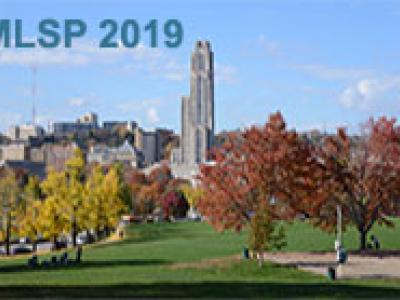
- Read more about VISUALIZING HIGH DIMENSIONAL DYNAMICAL PROCESSES
- Log in to post comments
- Categories:
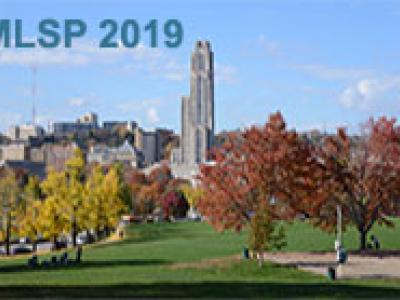
- Read more about Deep Clustering based on a Mixture of Autoencoders
- Log in to post comments
In this paper we propose a Deep Autoencoder Mixture Clustering(DAMIC) algorithm based on a mixture of deep autoencoders whereeach cluster is represented by an autoencoder. A clustering networktransforms the data into another space and then selects one of theclusters. Next, the autoencoder associated with this cluster is usedto reconstruct the data-point. The clustering algorithm jointly learnsthe nonlinear data representation and the set of autoencoders. Theoptimal clustering is found by minimizing the reconstruction loss ofthe mixture of autoencoder network.
- Categories:
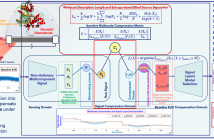
- Read more about Unsupervised Drift Compensation Based on Information Theory for Single-Molecule Sensors
- Log in to post comments
Single-molecule sensors based on carbon nanotubes transducer, enable to probe stochastic molecular dynamics thanks to long acquisition periods and high throughput measurements. With such sampling conditions, the sensor baseline may drift significantly and induce fake states and transitions in the recorded signal, leading to wrong kinetic estimates from the inferred state model.
We present MDL-AdaCHIP a multiscale signal compression technique based on the Minimum Description Length (MDL) principle, combined with an Adaptive piecewise Cubic Hermite Interpolation (AdaCHIP), both implemented into a blind source separation framework to compensate the parasitic baseline drift in single-molecule biosensors
- Categories:
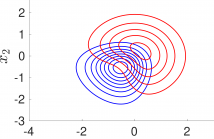
- Read more about Robust importance-weighted cross-validation under sample selection bias
- Log in to post comments
Cross-validation under sample selection bias can, in principle, be done by importance-weighting the empirical risk. However, the importance-weighted risk estimator produces sub-optimal hyperparameter estimates in problem settings where large weights arise with high probability. We study its sampling variance as a function of the training data distribution and introduce a control variate to increase its robustness to problematically large weights.
- Categories:
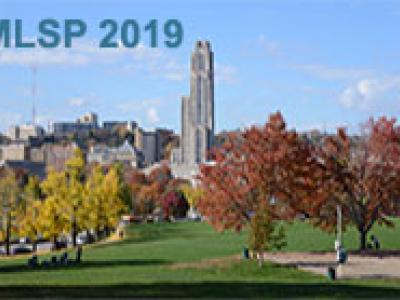
- Read more about VAE/WGAN-BASED IMAGE REPRESENTATION LEARNING FOR POSE-PRESERVING SEAMLESS IDENTITY REPLACEMENT IN FACIAL IMAGES
- Log in to post comments
We present a novel variational generative adversarial network (VGAN) based on Wasserstein loss to learn a latent representation
from a face image that is invariant to identity but preserves head-pose information. This facilitates synthesis of a realistic face
image with the same head pose as a given input image, but with a different identity. One application of this network is in
privacy-sensitive scenarios; after identity replacement in an image, utility, such as head pose, can still
- Categories:
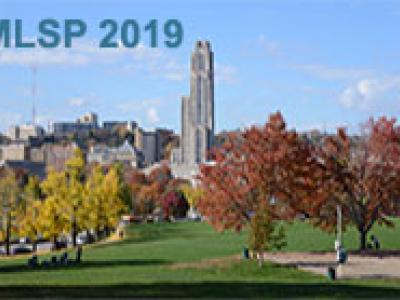
- Read more about A Benchmark Study of Backdoor Data Poisoning Defenses for Deep Neural Network Classifiers and A Novel Defense
- Log in to post comments
While data poisoning attacks on classifiers were originally proposed to degrade a classifier's usability, there has been strong recent interest in backdoor data poisoning attacks, where the classifier learns to classify to a target class whenever a backdoor pattern ({\it e.g.}, a watermark or innocuous pattern) is added to an example from some class other than the target class.
- Categories: