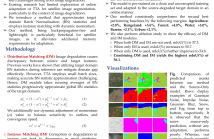
- Read more about Source-Free Online Domain Adaptive Semantic Segmentation of Satellite Images under Image Degradation
- Log in to post comments
Online adaptation to distribution shifts in satellite image segmentation stands as a crucial yet underexplored problem. In this paper, we address source-free and online domain adaptation, i.e., test-time adaptation (TTA), for satellite images, with the focus on mitigating distribution shifts caused by various forms of image degradation. Towards achieving this goal, we propose a novel TTA approach involving two effective strategies. First, we progressively estimate the global Batch Normalization (BN) statistics of the target distribution with incoming data stream.
- Categories:
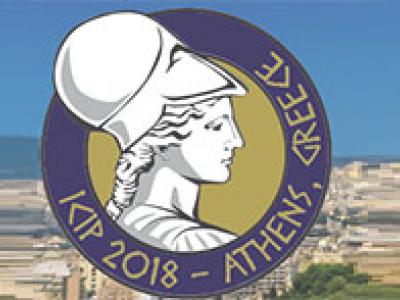
- Read more about Learning Semantics-Guided Visual Attention for Few-shot Image Classification
- Log in to post comments
We propose a deep learning framework for few-shot image classification, which exploits information across label semantics and image domains, so that regions of interest can be properly attended for improved classification. The proposed semantics-guided attention module is able to focus on most relevant regions in an image, while the attended image samples allow data augmentation and alleviate possible overfitting during FSL training. Promising performances are presented in our experiments, in which we consider both closed and open-world settings.
- Categories:
- Read more about Multi-Glimpse LSTM with Color-Depth Feature Fusion for Human Detection
- Log in to post comments
With the development of depth cameras such as Kinect and Intel Realsense, RGB-D based human detection receives continuous research attention due to its usage in a variety of applications. In this paper, we propose a new Multi-Glimpse LSTM (MG-LSTM) network, in which multi-scale contextual information is sequentially integrated to promote the human detection performance. Furthermore, we propose a feature fusion strategy based on our MG-LSTM network to better incorporate the RGB and depth information.
ICIP_ORAL.pdf

- Categories:
- Read more about DEEP LEARNING ARCHITECTURE FOR PEDESTRIAN 3-D LOCALIZATION AND TRACKING USING MULTIPLE CAMERAS
- Log in to post comments
- Categories:
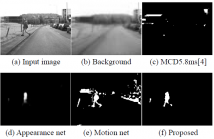
- Read more about Appearance and Motion based Deep Learning Architecture for Moving Object Detection in Moving Camera
- Log in to post comments
Background subtraction from the given image is a widely used method for moving object detection. However, this method is vulnerable to dynamic background in a moving camera video. In this paper, we propose a novel moving object detection approach using deep learning to achieve a robust performance even in a dynamic background. The proposed approach considers appearance features as well as motion features. To this end, we design a deep learning architecture composed of two networks: an appearance network and a motion network.
ICIP17_heo.pdf

- Categories:
- Read more about ICIP2017_Incremental zero-shot learning based on attributes for image classification
- Log in to post comments
Instead of assuming a closed-world environment comprising a fixed number of objects, modern pattern recognition systems need to recognize outliers, identify anomalies, or discover entirely new objects, which is known as zero-shot object recognition. However, many existing zero-shot learning methods are not efficient enough to incrementally update themselves with new samples mixed with known or novel class labels. In this paper, we propose an incremental zero-shot learning framework (IIAP/QR) based on indirect-attribute-prediction (IAP) model. Firstly, a fast incremental
- Categories: