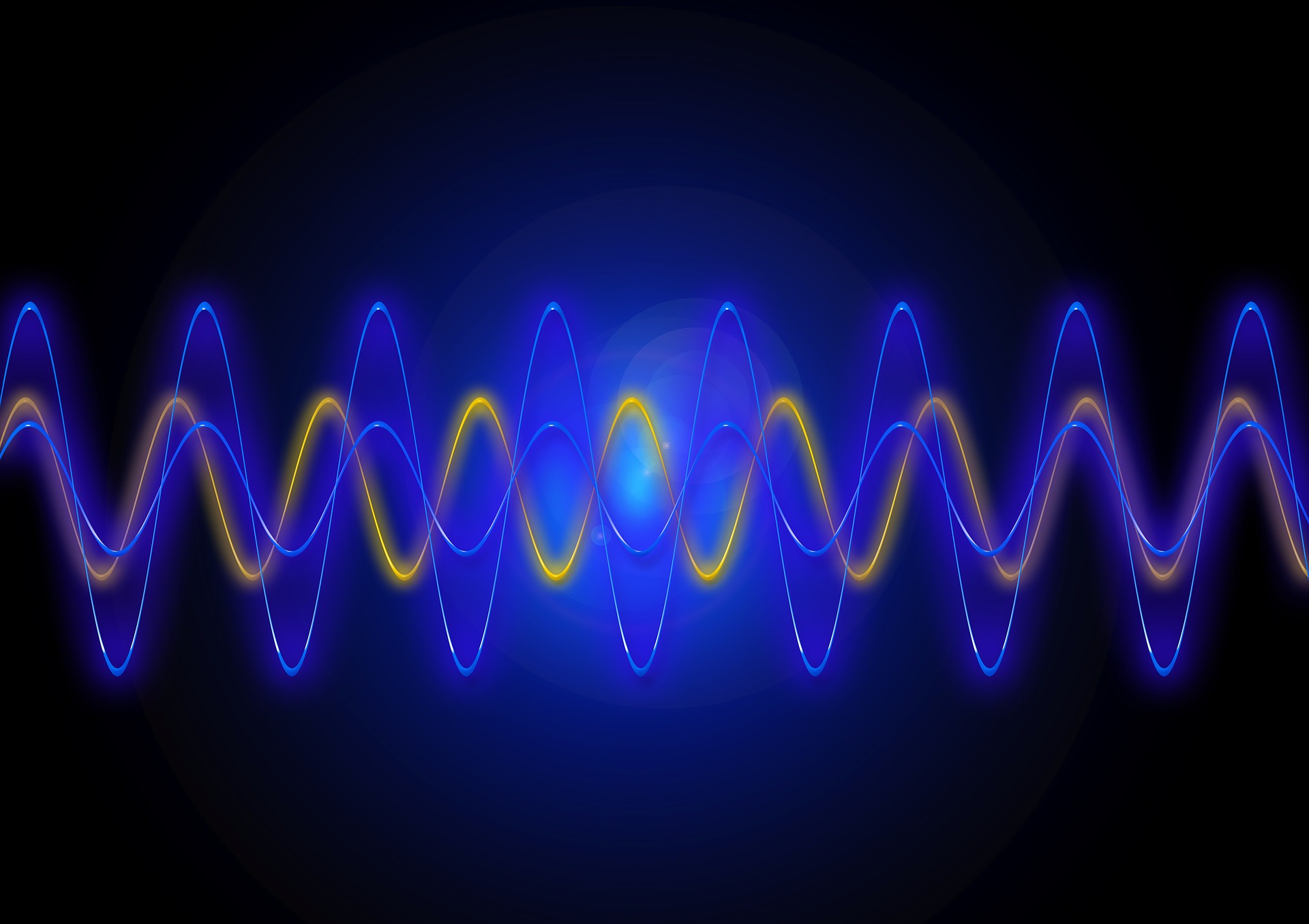
- Read more about GIVE: A Multi-Agent Framework for Generating Immersive Multi-Modal Virtual Environments for 3D Games - Supplementary Material
- Log in to post comments
In this work, we present a novel multi-agent framework for generating immersive 3D virtual environments from high-level semantic inputs, powered by large language and vision-language models (LLMs/VLMs). Unlike prior work that focuses primarily on visual output, data-intensive training pipelines, and code generation, our system coordinates a team of specialized agents, each assigned a role such as manager, planner, or expert in visual, audio, or spatial domains, to decompose and execute environment construction tasks within a game engine.
- Categories:
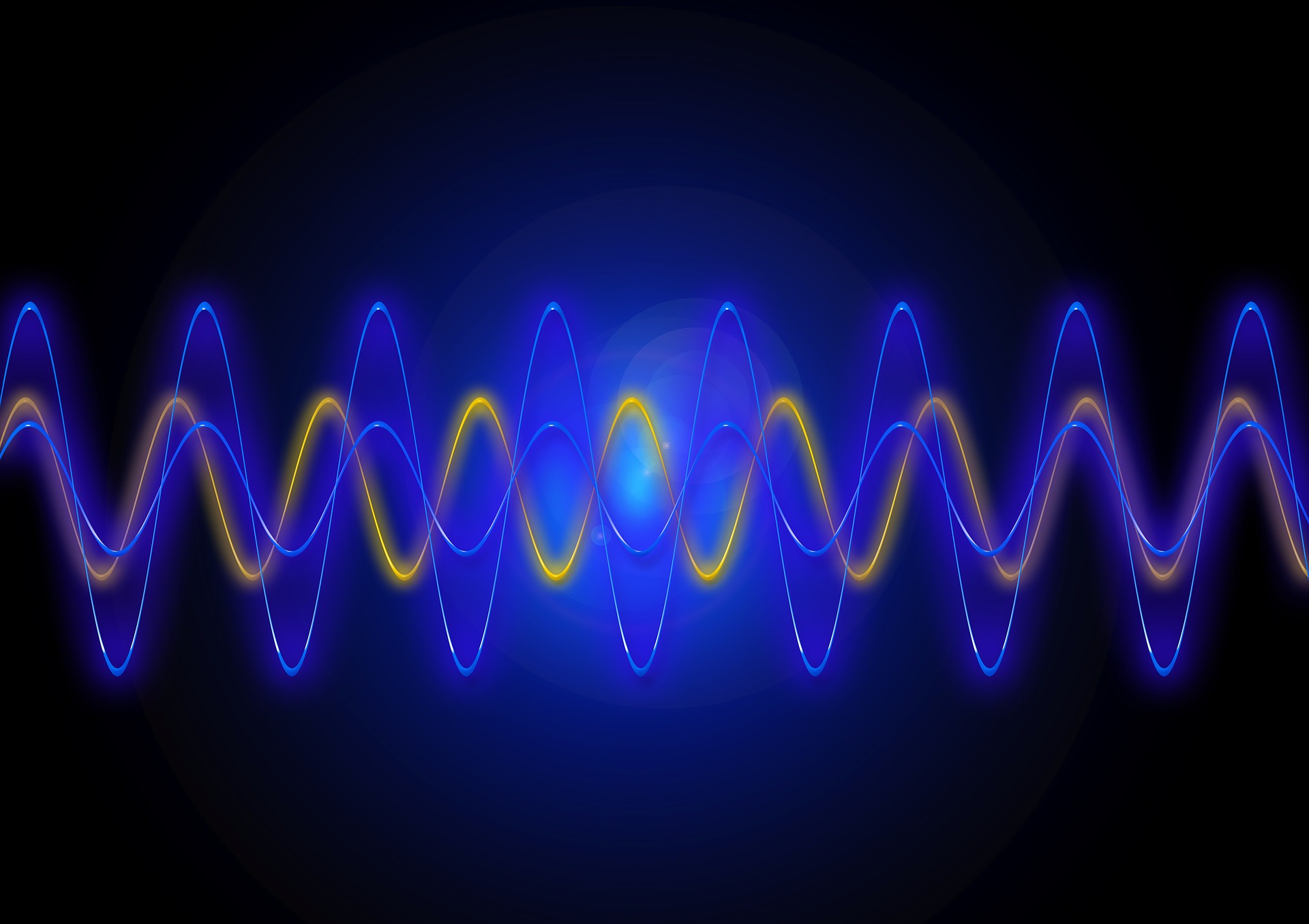
- Read more about (Appendix) Rethinking the Backbone in Class Imbalanced Federated Source Free Domain Adaptation: The Utility of Vision Foundation Models
- Log in to post comments
Appendix of our submitted paper: "Rethinking the Backbone in Class Imbalanced Federated Source Free Domain Adaptation: The Utility of Vision Foundation Models"
- Categories:
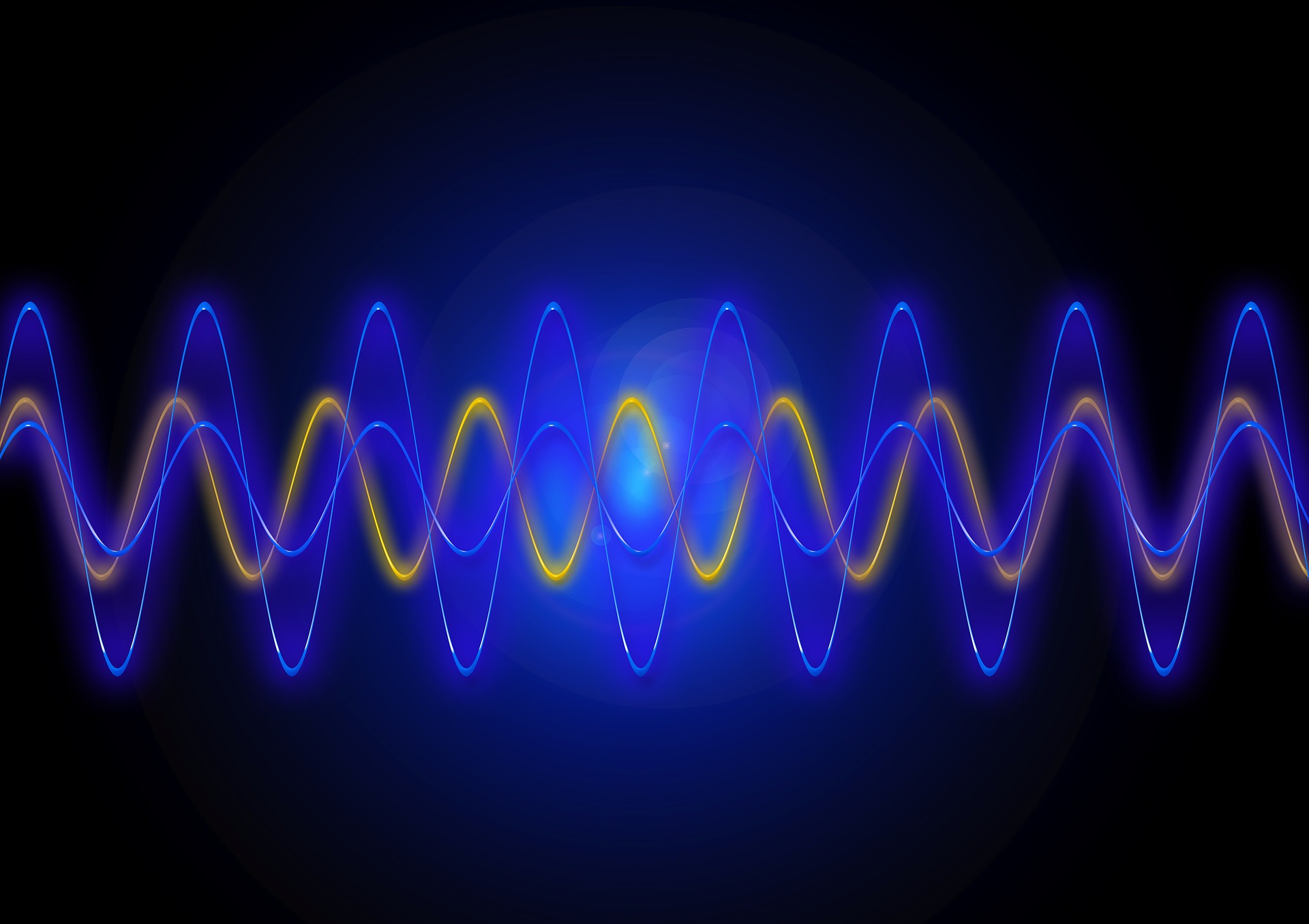
- Read more about ICIP2025 supplementary material camera ready
- Log in to post comments
We address the challenges of local feature matching under large scale and rotation changes by focusing on keypoint positions.
First, we propose a novel module called similarity normalization (SN).
This module normalizes keypoint positions to remove translation, rotation and scale differences between image pairs.
By performing positional encoding on these normalized positions, a network incorporating with SN can effectively avoid encoding largely different positions into descriptors from the two images.
- Categories:
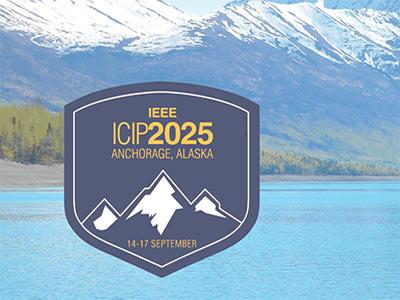
- Read more about ICIP 2025 Supplementary
- Log in to post comments
This supplementary material accompanies our paper titled "Texturing Endoscopic 3D Stomach via Neural Radiance Field under Uneven Lighting."
- Categories:
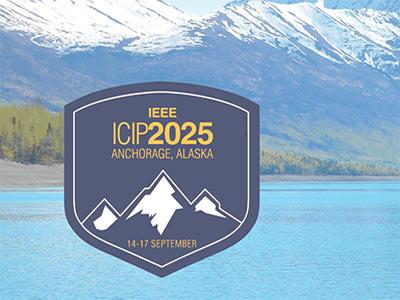
- Read more about (Appendix) MultiMAE Meets Earth Observation: Pre-training Multi-modal Multi-task Masked Autoencoders for Earth Observation Tasks
- Log in to post comments
MULTIMAE MEETS EARTH OBSERVATION: PRE-TRAINING MULTI-MODAL MULTI-TASK MASKED AUTOENCODERS FOR EARTH OBSERVATION TASKS (APPENDIX)
- Categories:
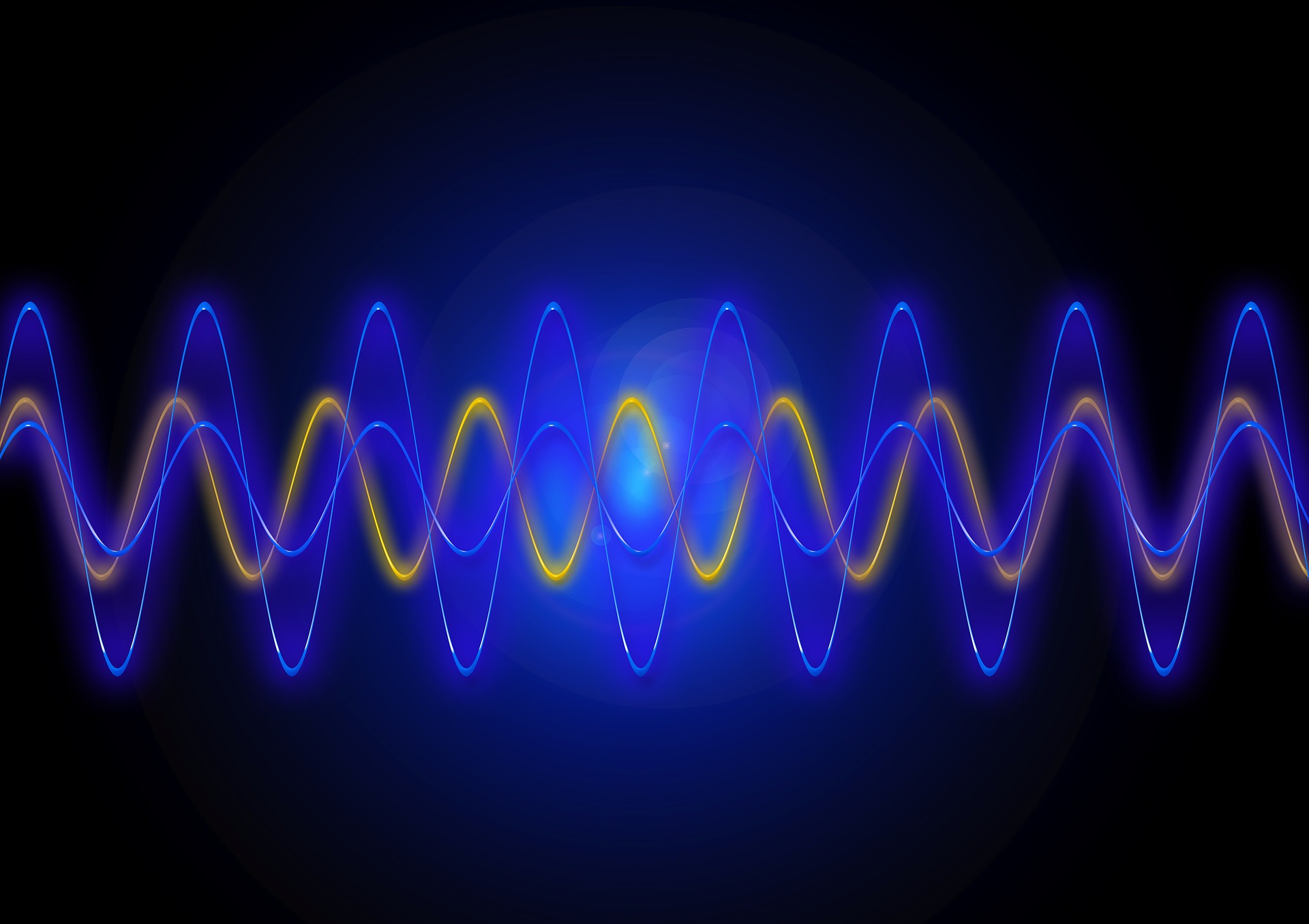
- Read more about Supplmental - o1-mini prompt
- Log in to post comments
O1-mini prompt to expose the seven components of agricultural disease management evaluation framework
- Categories:
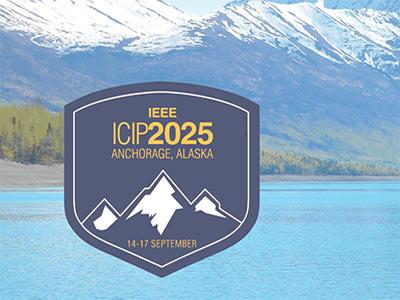
- Read more about Shuffle PatchMix Augmentation with Confidence Margin Weighted Pseudo-Labels for Enhanced Source-Free Domain Adaptation
- Log in to post comments
Supplementary Material.
- Categories:
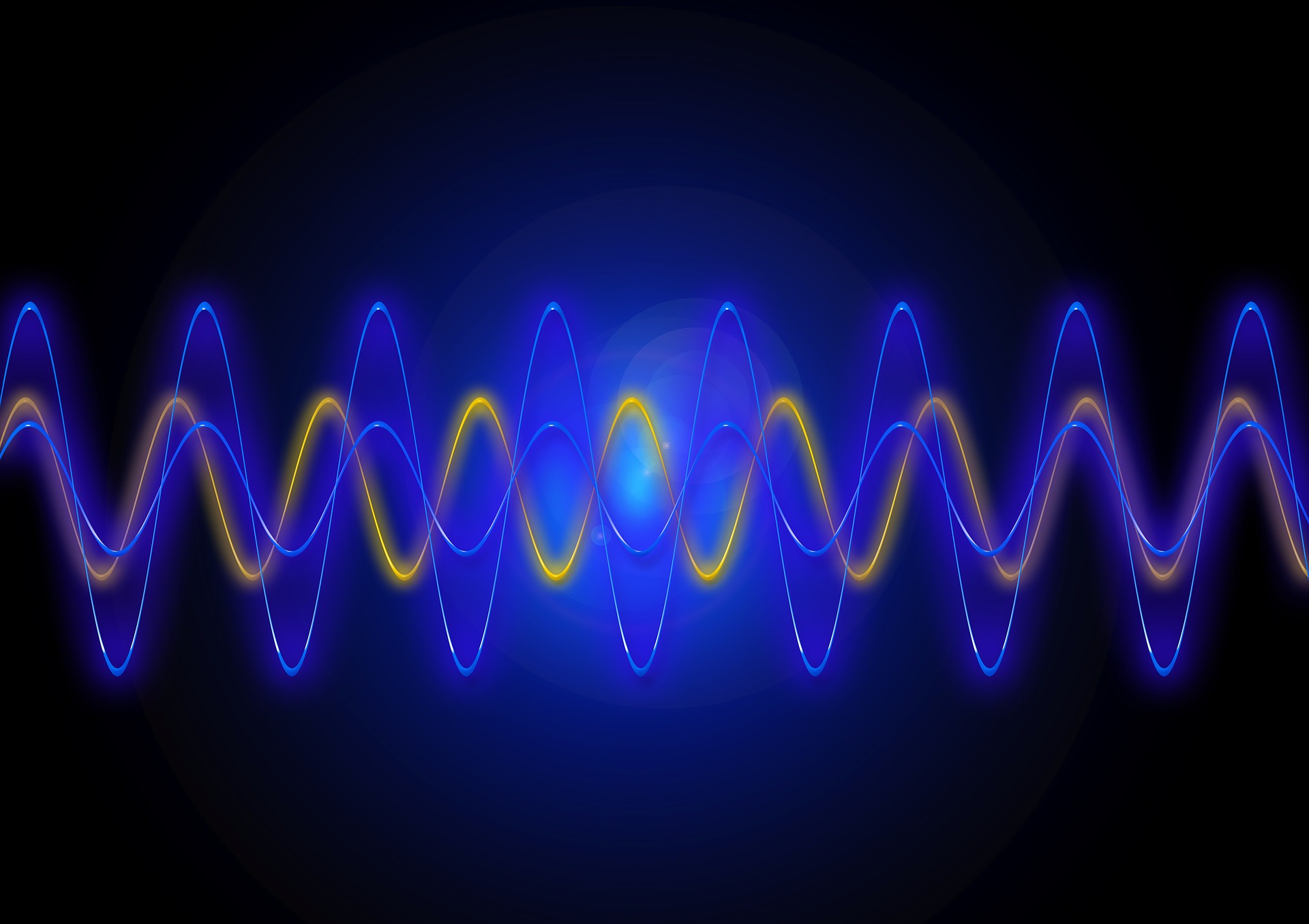
- Read more about Supplementary Material ICCE-TW 2025
- Log in to post comments
This supplementary material presents detailed transformer model architectures, training parameters, and comprehensive evaluation metrics to complement our comparison of RNN and transformer models for Indonesian news classification. Our analysis provides deeper insights into why transformer models outperform RNN approaches despite their larger parameter counts.
TT-TW25.pdf

- Categories:
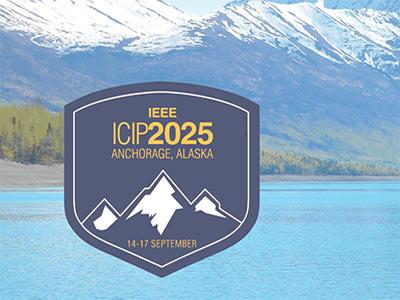
- Read more about TASE 2025 supp
- Log in to post comments
Supp ro TASE 2025 submission
- Categories:
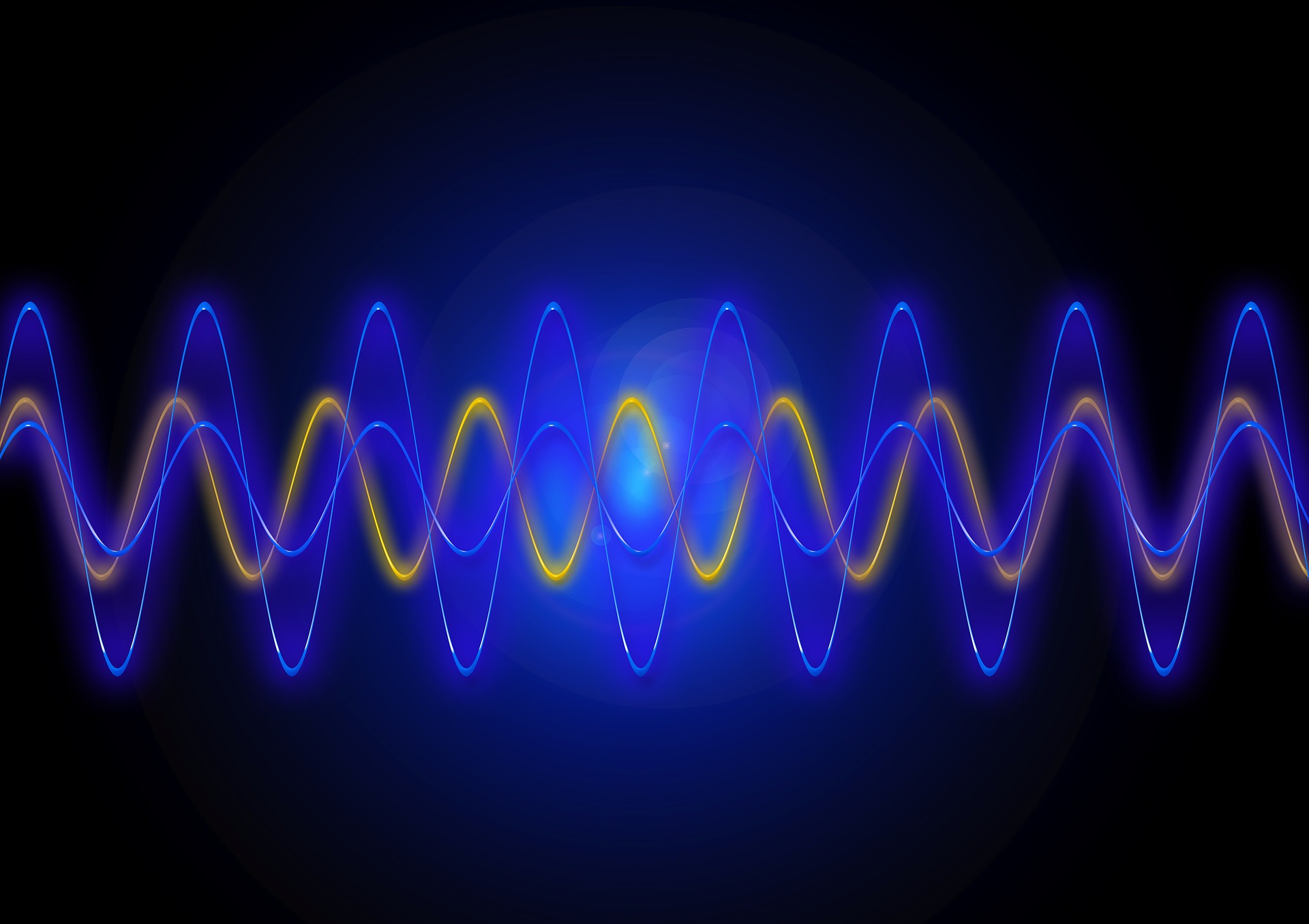