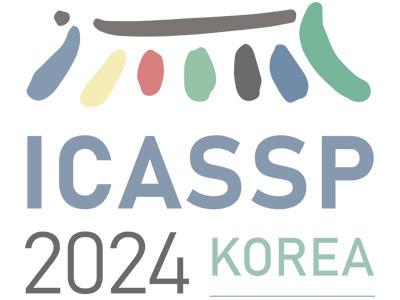
- Read more about ENHANCING NOISY LABEL LEARNING VIA UNSUPERVISED CONTRASTIVE LOSS WITH LABEL CORRECTION BASED ON PRIOR KNOWLEDGE
- Log in to post comments
To alleviate the negative impacts of noisy labels, most of the noisy label learning (NLL) methods dynamically divide the training data into two types, “clean samples” and “noisy samples”, in the training process. However, the conventional selection of clean samples heavily depends on the features learned in the early stages of training, making it difficult to guarantee the cleanliness of the selected samples in scenarios where the noise ratio is high.
- Categories:
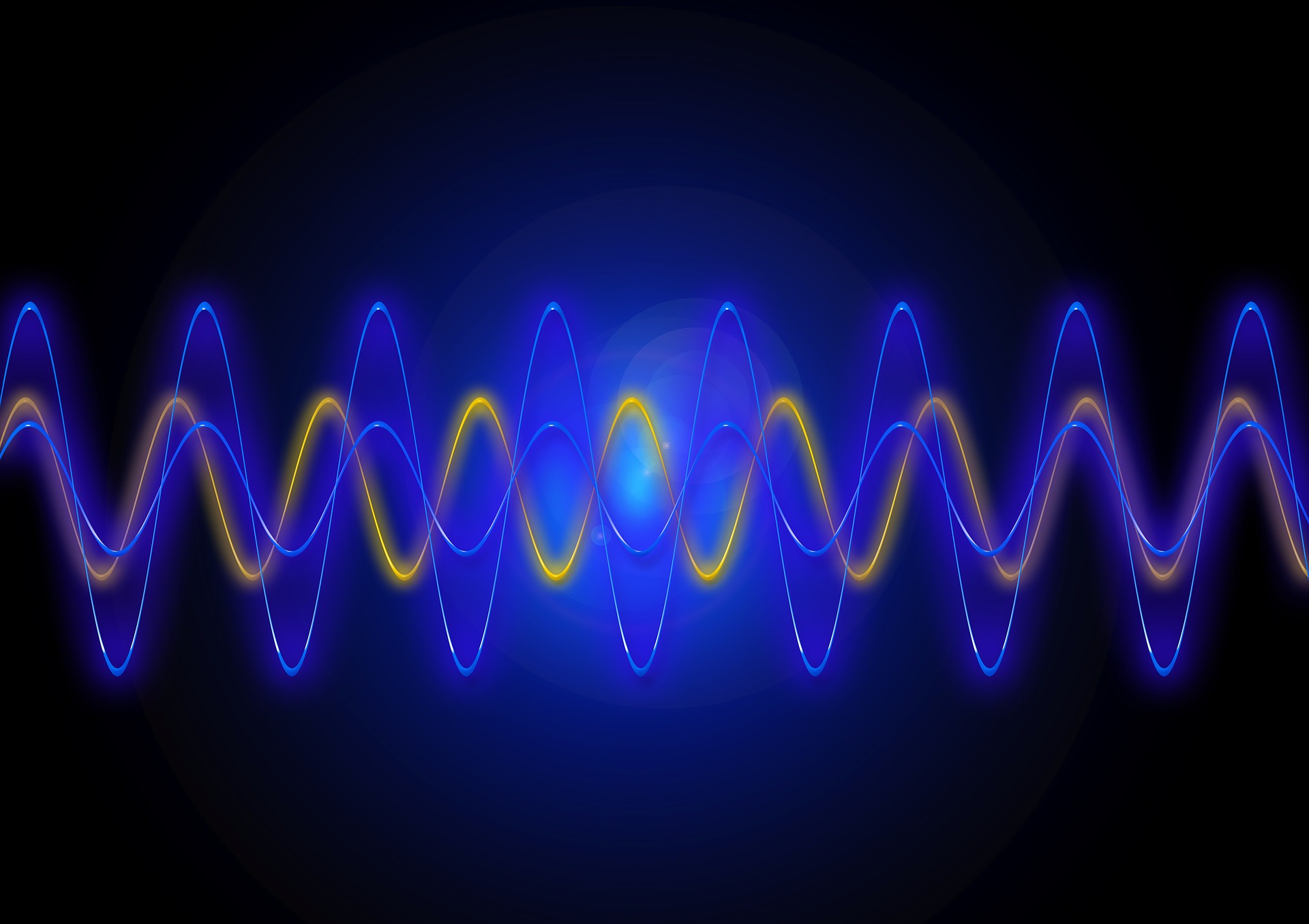
- Read more about A_Binary_BP_Decoding_using_Posterior_Adjustment_for_Quantum_LDPC_Codes
- Log in to post comments
Although BP decoders are efficient and provide significant
performance for classical low-density parity-check (LDPC)
codes, they will suffer a degradation in performance for quantum
LDPC (QLDPC) codes due to the limitations in the quantum
field. In this paper, we propose a posterior adjustment of
either a single qubit or multiple qubits within binary belief
propagation (BP). The adjustment process changes the posterior
likelihood ratio for one or multiple qubits according to the
- Categories:
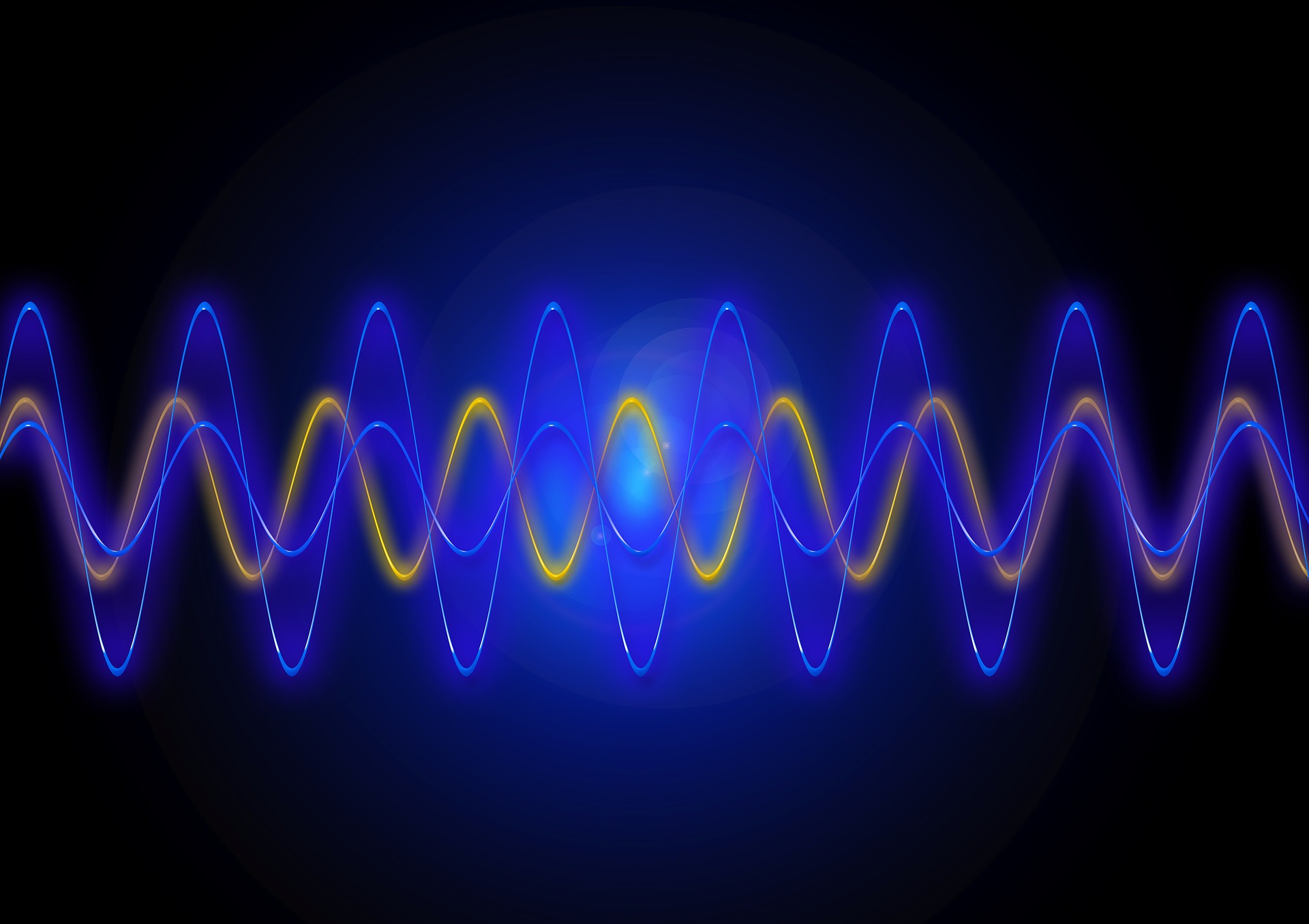
- Read more about Inference of genetic effects via Approximate Message Passing
- Log in to post comments
Efficient utilization of large-scale biobank data is crucial for inferring the genetic basis of disease and predicting health outcomes from the DNA. Yet we lack efficient, accurate methods that scale to data where electronic health records are linked to whole genome sequence information. To address this issue, our paper develops a new algorithmic paradigm based on Approximate Message Passing (AMP), which is specifically tailored for genomic prediction and association testing.
- Categories:
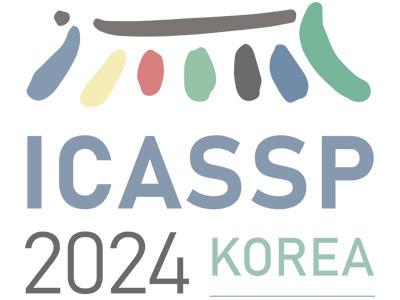
- Read more about EFFICIENT VIDEO AND AUDIO PROCESSING WITH LOIHI 2
- Log in to post comments
Loihi 2 is a fully event-based neuromorphic processor that supports a wide range of synaptic connectivity configurations and temporal neuron dynamics. Loihi 2's temporal and event-based paradigm is naturally well-suited to processing data from an event-based sensor, such as a Dynamic Vision Sensor (DVS) or a Silicon Cochlea. However, this begs the question: How general are signal processing efficiency gains on Loihi 2 versus conventional computer architectures?
- Categories:
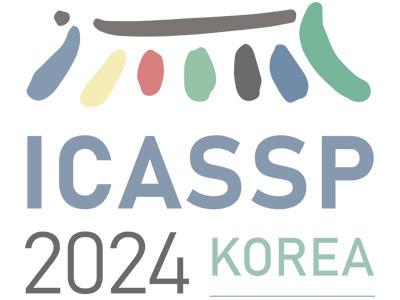
- Read more about Slides for Renyi Divergences Learning for explainable classification of SAR Image Pairs
- Log in to post comments
We consider the problem of classifying a pair of Synthetic Aperture Radar (SAR) images by proposing an explainable and frugal algorithm that integrates a set of divergences. The approach relies on a statistical framework that takes standard probability distributions into account for modelling SAR data. Then, by learning a combination of parameterized Renyi divergences and their parameters from the data, we are able to classify the pair of images with fewer parameters than regular machine learning approaches while also allowing an interpretation of the results related to the priors used.
- Categories:
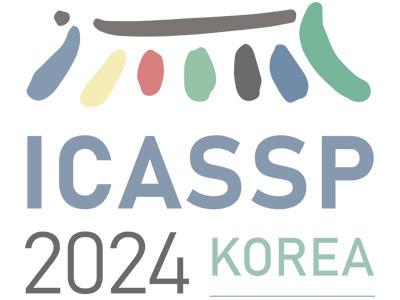
- Read more about GENERATING PERSONA-AWARE EMPATHETIC RESPONSES WITH RETRIEVAL-AUGMENTED PROMPT LEARNING
- 2 comments
- Log in to post comments
Empathetic response generation requires perceiving and un- derstanding the user’s emotion to deliver a suitable response. However, existing models generally remain oblivious of an interlocutor’s persona, which has been shown to play a vital role in expressing appropriate empathy to different users. To address this problem, we propose a novel Transformer-based architecture that incorporates retrieval-augmented prompt learning to generate persona-aware empathetic responses.
- Categories:
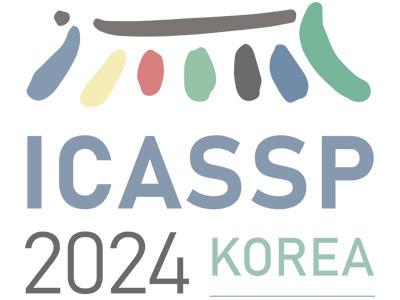
- Read more about Enhanced Axle-Based Vehicle Classification Using Angle-Based Micro-Doppler Signature
- Log in to post comments
This study introduces an angle-based micro-Doppler analysis using Frequency Modulated Continuous Wave (FMCW) radar tailored for axle-based vehicle classification. The novel approach exploits the signal angle of arrival to separate incoming signals and noise from distinct targets. This is done by analysing the phase difference of a dual antenna radar system based on the time-frequency representation of the radar beat signal. Vehicles driving side by side can now be discriminated. Multipath signals and clutter are more easily identified and filtered out.
- Categories:
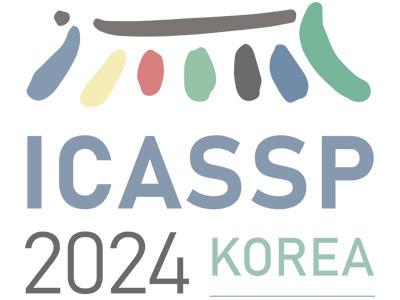
- Read more about CO-OCCURRENCE GRAPH-ENHANCED HIERARCHICAL PREDICTION OF ICD CODES
- Log in to post comments
Recent healthcare applications of natural language processing involve multi-label classification of health records using the International Classification of Diseases (ICD). While prior research highlights intricate text models and explores external knowledge like hierarchical ICD ontology, fewer studies integrate code relationships from whole datasets to enhance ICD coding accuracy. This study presents a modular approach, sequentially combining graph-based integration of ICD code co-occurrence with a hard-coded hierarchical enriched text representation drawn from the ICD ontology.
- Categories:
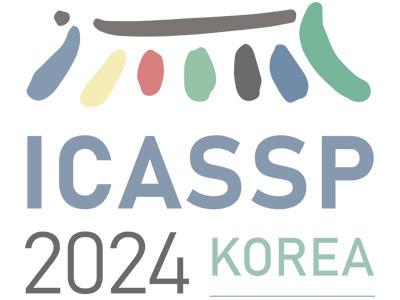
- Read more about Enabling Device Control Planning Capabilities of Small Language Model
- Log in to post comments
Smart home device control is a difficult task if the instruction is abstract and the planner needs to adjust dynamic home configurations. With the increasing capability of Large Language Model (LLM), they have become the customary model for zero-shot planning tasks similar to smart home device control. Although cloud supported large language models can seamlessly do device control tasks, on-device small language models show limited capabilities. In this work, we show how we can leverage large language models to enable small language models for device control task.
- Categories:
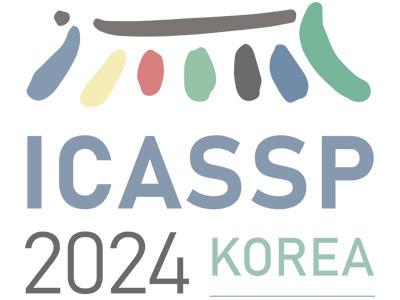
- Read more about dklement_dvbx_slides
- Log in to post comments
Bayesian HMM clustering of x-vector sequences (VBx) has become a widely adopted diarization baseline model in publications and challenges. It uses an HMM to model speaker turns, a generatively trained probabilistic linear discriminant analysis (PLDA) for speaker distribution modeling, and Bayesian inference to estimate the assignment of x-vectors to speakers. This paper presents a new framework for updating the VBx parameters using discriminative training, which directly optimizes a predefined loss.
- Categories: