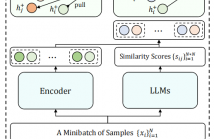
- Read more about Robust Self-Supervised Learning With Contrast Samples For Natural Language Understanding
- Log in to post comments
To improve the robustness of pre-trained language models (PLMs), previous studies have focused more on how to efficiently obtain adversarial samples with similar semantics, but less attention has been paid to the perturbed samples that change the gold label. Therefore, to fully perceive the effects of these different types of small perturbations on robustness, we propose a RObust Self-supervised leArning (ROSA) method, which incorporates different types of perturbed samples and the robustness improvements into a unified framework.
- Categories:
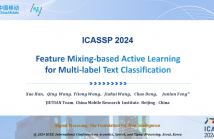
- Read more about Feature Mixing-based Active Learning for Multi-label Text Classification
- Log in to post comments
Active learning (AL) aims to reduce labeling costs by selecting the most valuable samples to annotate from a set of unlabeled data. However, recognizing these samples is particularly challenging in multi-label text classification tasks due to the high dimensionality but sparseness of label spaces. Existing AL techniques either fail to sufficiently capture label correlations, resulting in label imbalance in the selected samples, or suffer significant computing costs when analyzing the informative potential of unlabeled samples across all labels.
- Categories:
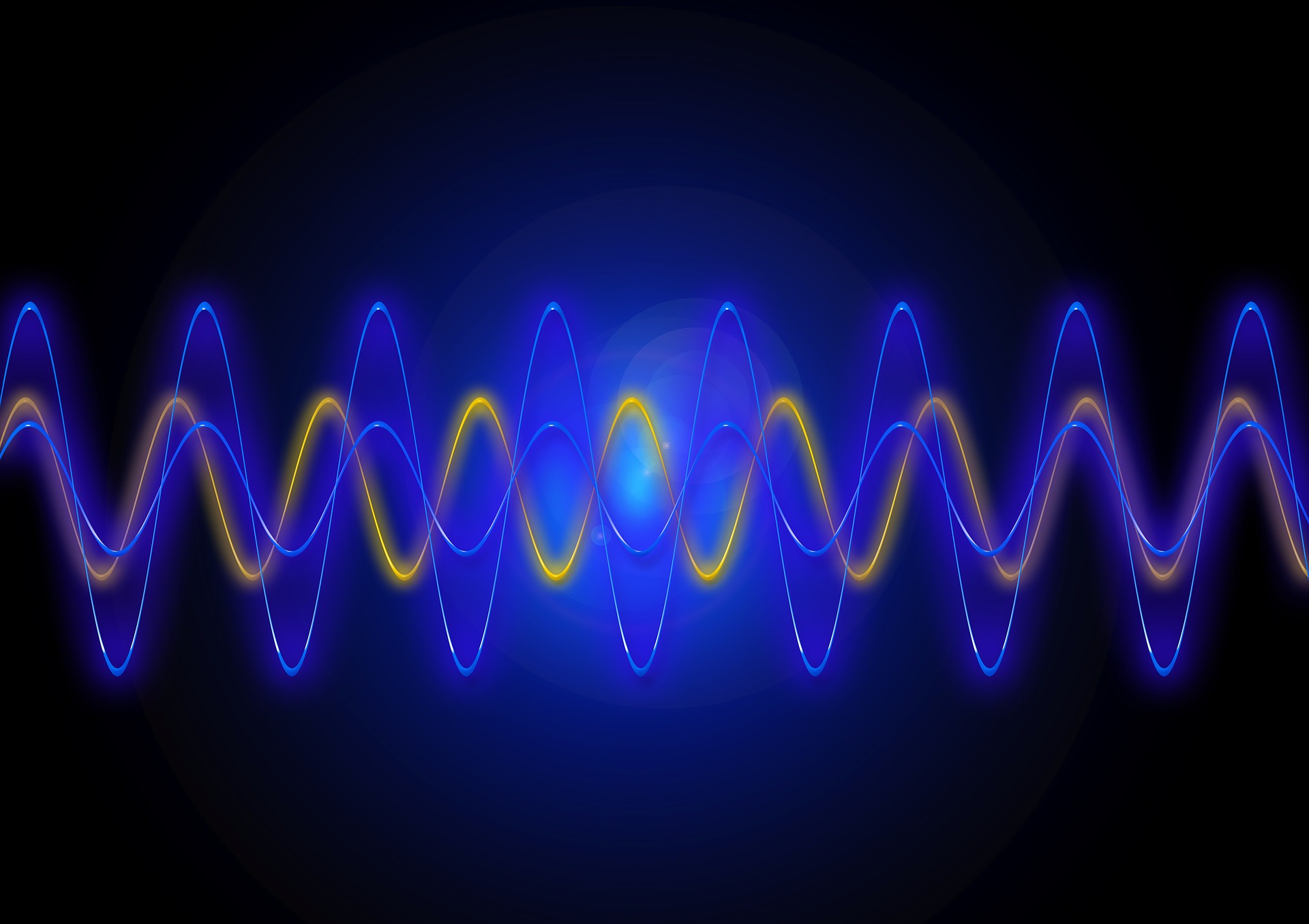
- Read more about A 3D VIRTUAL TRY-ON METHOD WITH GLOBAL-LOCAL ALIGNMENT AND DIFFUSION MODEL
- Log in to post comments
3D virtual try-on has recently received more attention due to its great practical and commercial value. However, there remains the problems that the garment cannot accurately correspond to a human body by geometric transformation and abnormal textures may be produced in the synthesis result. To address these issues, a 3D virtual try-on method with globallocal alignment and diffusion model is proposed. The globallocal alignment module is designed for more accurate garment warping, combined with the guidance of an “after-tryon” semantic map alignment.
poster.pdf

- Categories:
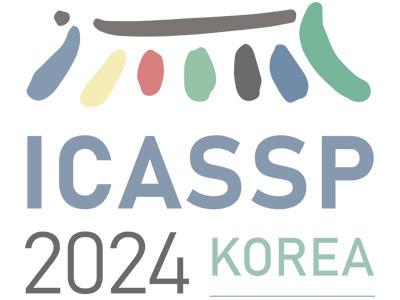
- Read more about NEURAL NETWORK-BASED SYMBOLIC REGRESSION FOR EMPIRICAL MODELING OF THE BEHAVIOR OF A PLANETARY GEARBOX
- Log in to post comments
Gearbox condition monitoring and quality surveillance are crucial techniques to ensure safe and cost-efficient machine operations. In condition monitoring, the interpretation of the different vibration spectrum elements is still an open question, many works show that some predefined vibration models are improper to explain the spectrum contents. In this paper, we investigate a method to identify the mixture model that describes a single-stage planetary gearbox vibration to properly interpret the vibration spectrum.
- Categories:
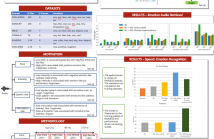
- Read more about PROMPTING AUDIOS USING ACOUSTIC PROPERTIES FOR EMOTION REPRESENTATION
- Log in to post comments
Emotions lie on a continuum, but current models treat emotions
as a finite valued discrete variable. This representation does not
capture the diversity in the expression of emotion. To better rep-
resent emotions we propose the use of natural language descrip-
tions (or prompts). In this work, we address the challenge of au-
tomatically generating these prompts and training a model to better
learn emotion representations from audio and prompt pairs. We use
acoustic properties that are correlated to emotion like pitch, intensity,
- Categories:
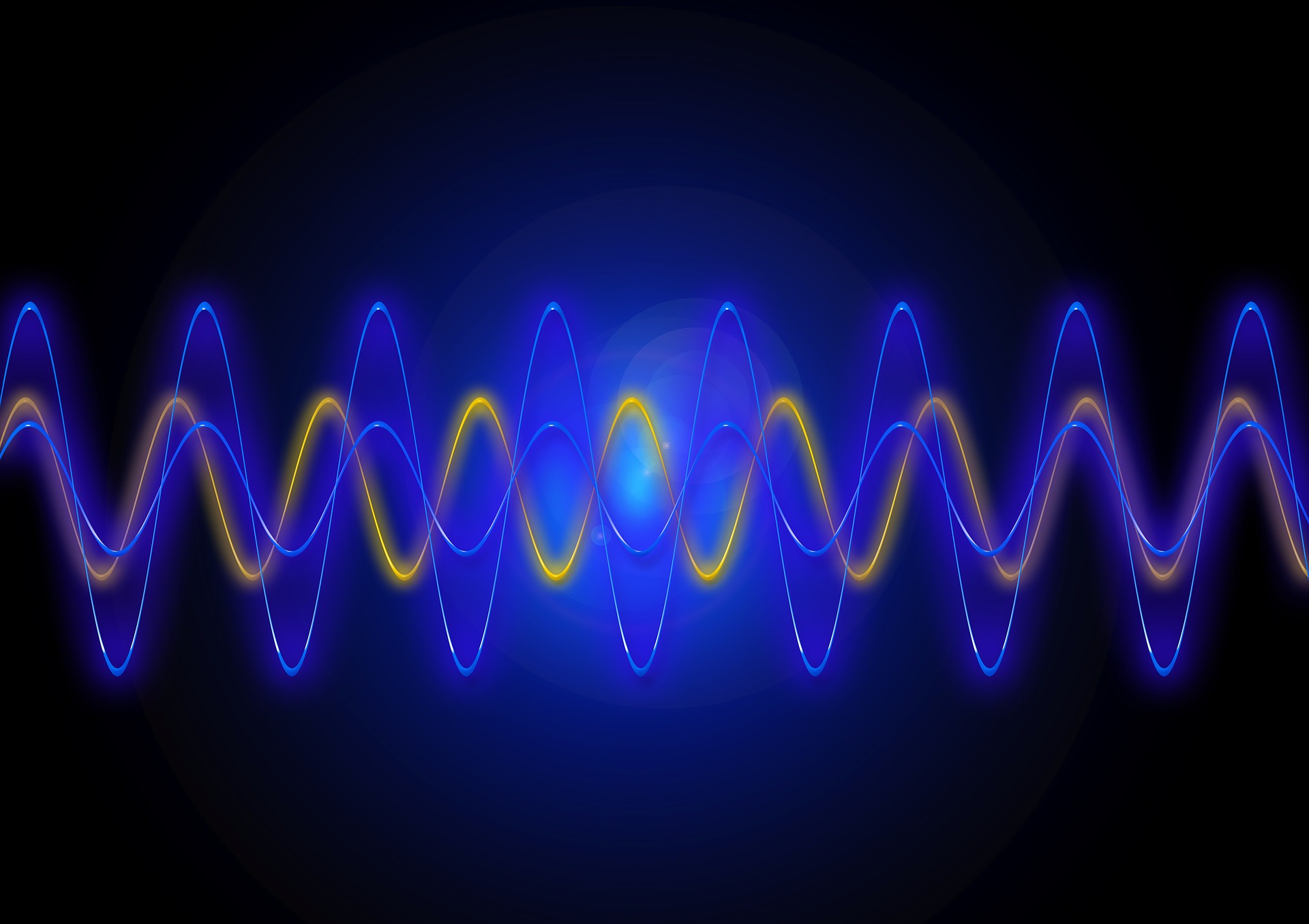
- Read more about FOVEA TRANSFORMER: EFFICIENT LONG-CONTEXT MODELING WITH STRUCTURED FINE-TO-COARSE ATTENTION
- Log in to post comments
The quadratic complexity of self-attention in Transformers has hindered the processing of long text. To alleviate this problem, previous works have proposed to sparsify the attention matrix, taking advantage of the observation that crucial information about a token can be derived from its neighbors. These methods typically combine one or another form of local attention and global attention. Such combinations introduce abrupt changes in contextual granularity when going from local to global, which may be undesirable.
- Categories:
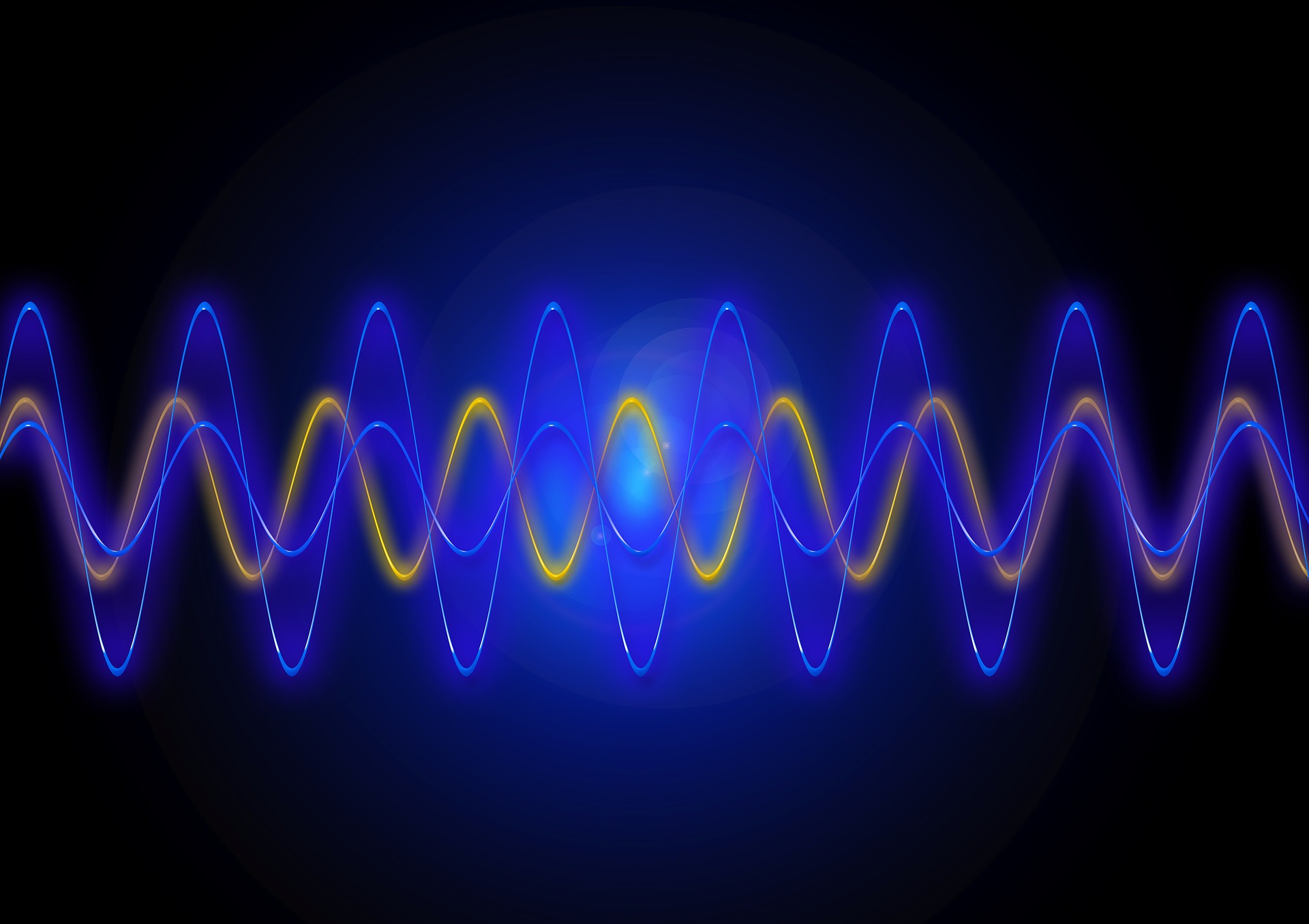
- Read more about NEW INTENT DISCOVERY WITH MULTI-VIEW CLUSTERING
- Log in to post comments
New intent discovery aims to identify new intents from unlabeled utterances in the open-world scenario. As the fundamental and challenging problem in dialogue systems, new intent discovery attracts increasing attention but is still under exploration. In this paper, we propose a simple and effective new intent discovery framework with multi-view clustering. Specifically, we first adopt a double-branch representation learning strategy to learn high-quality utterance representations.
- Categories:
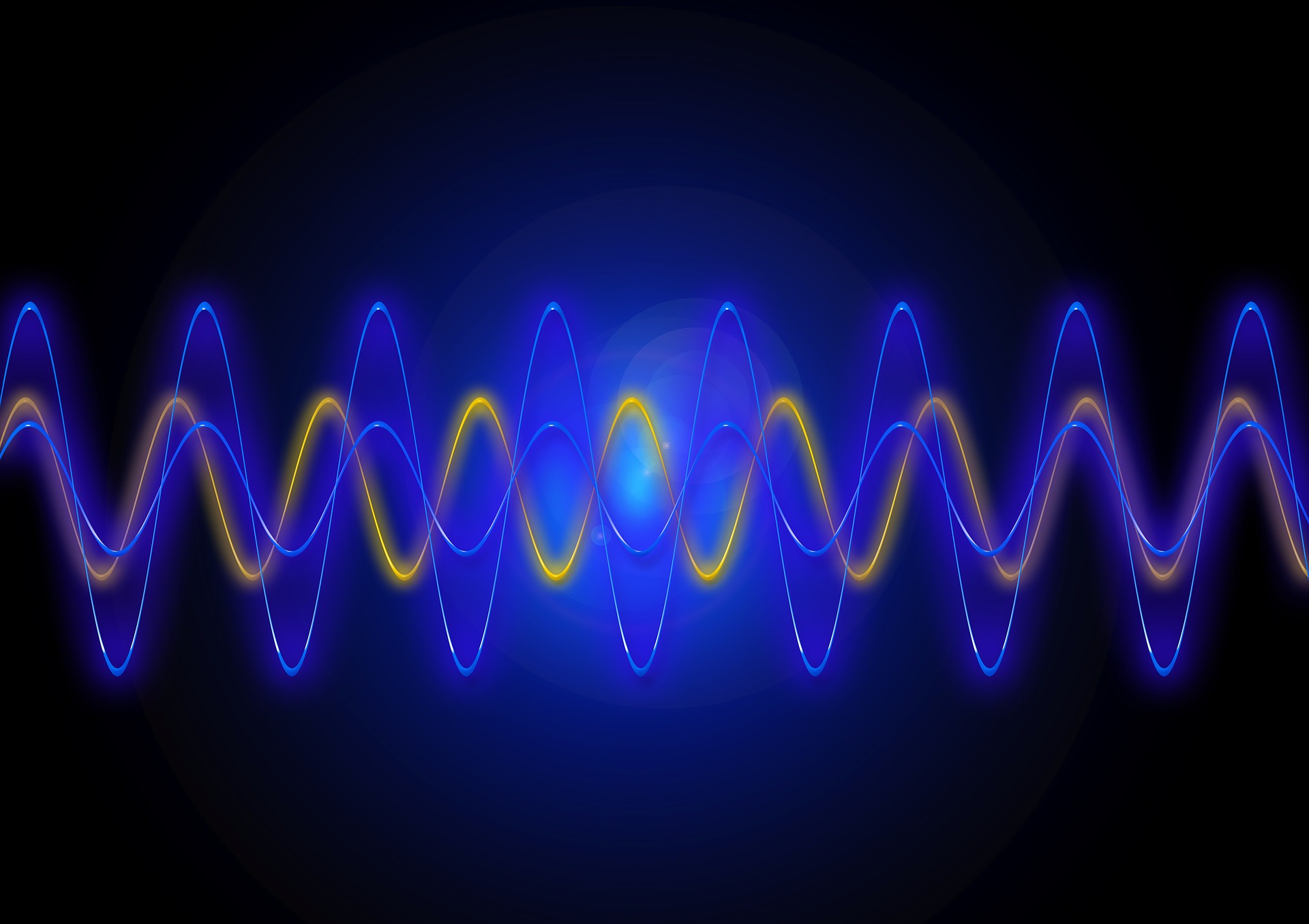
- Read more about Neural Ordinary differential equations with Trainable solvers
- 1 comment
- Log in to post comments
When considering the data-driven identification of non-linear differential equations, the choice of the integration scheme to use is far from being trivial and may dramatically impact the identification problem. In this work, we discuss this aspect and propose a novel architecture that jointly learns Neural Ordinary Differential Equations (NODEs) as well as the corresponding integration schemes that would minimize the forecast of a given sequence of observations. We demonstrate its relevance with numerical experiments on non-linear dynamics, including chaotic systems.
- Categories:
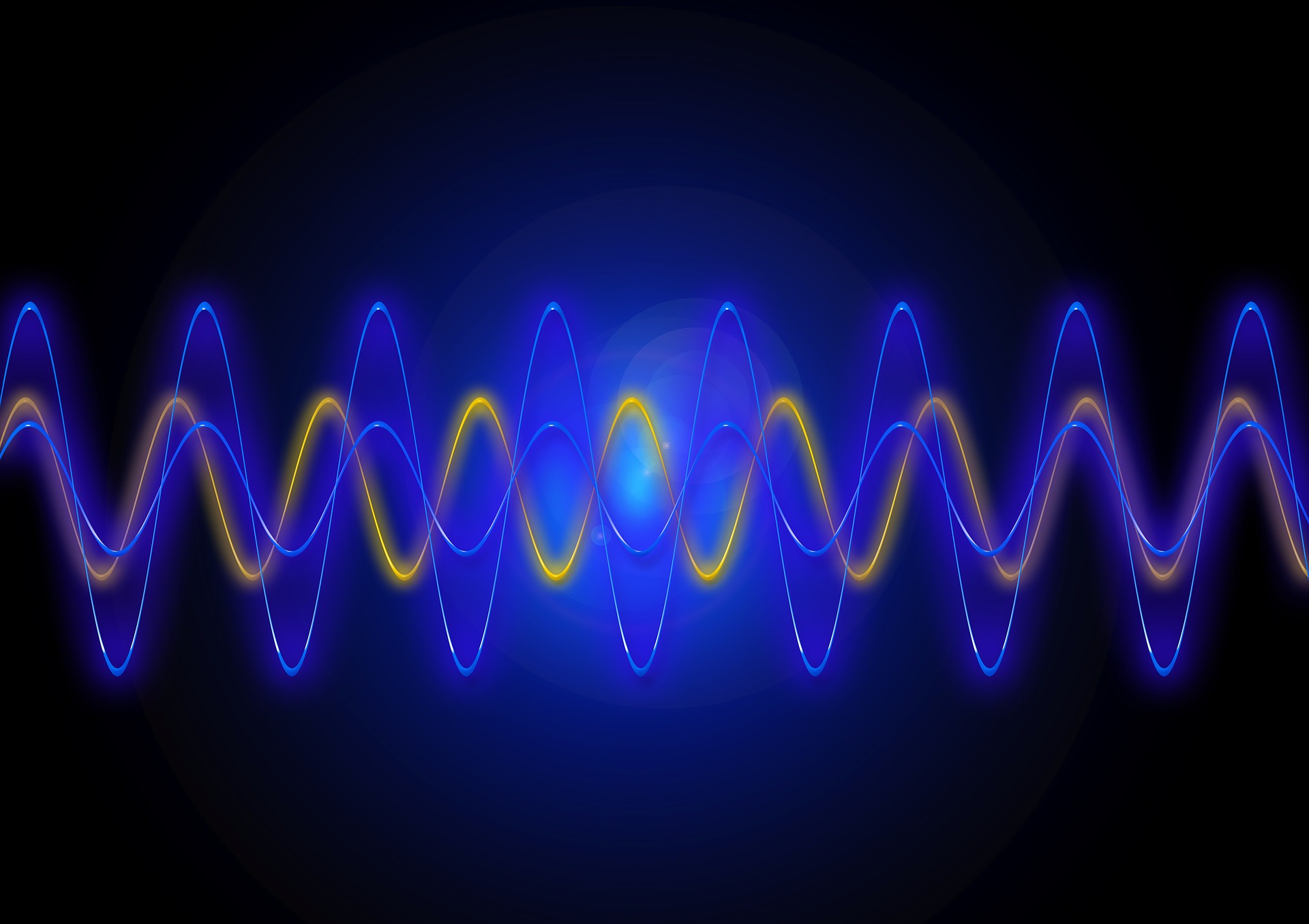
- Read more about CAN LLM FIND THE GREEN CIRCLE? INVESTIGATION AND HUMAN-GUIDED TOOL MANIPULATION FOR COMPOSITIONAL GENERALIZATION
- Log in to post comments
The meaning of complex phrases in natural language is composed of their individual components. The task of compositional generalization evaluates a model's ability to understand new combinations of components. Previous studies trained smaller, task-specific models, which exhibited poor generalization. While large language models (LLMs) exhibit impressive generalization abilities on many tasks through in-context learning (ICL), their potential for compositional generalization remains unexplored.
- Categories:
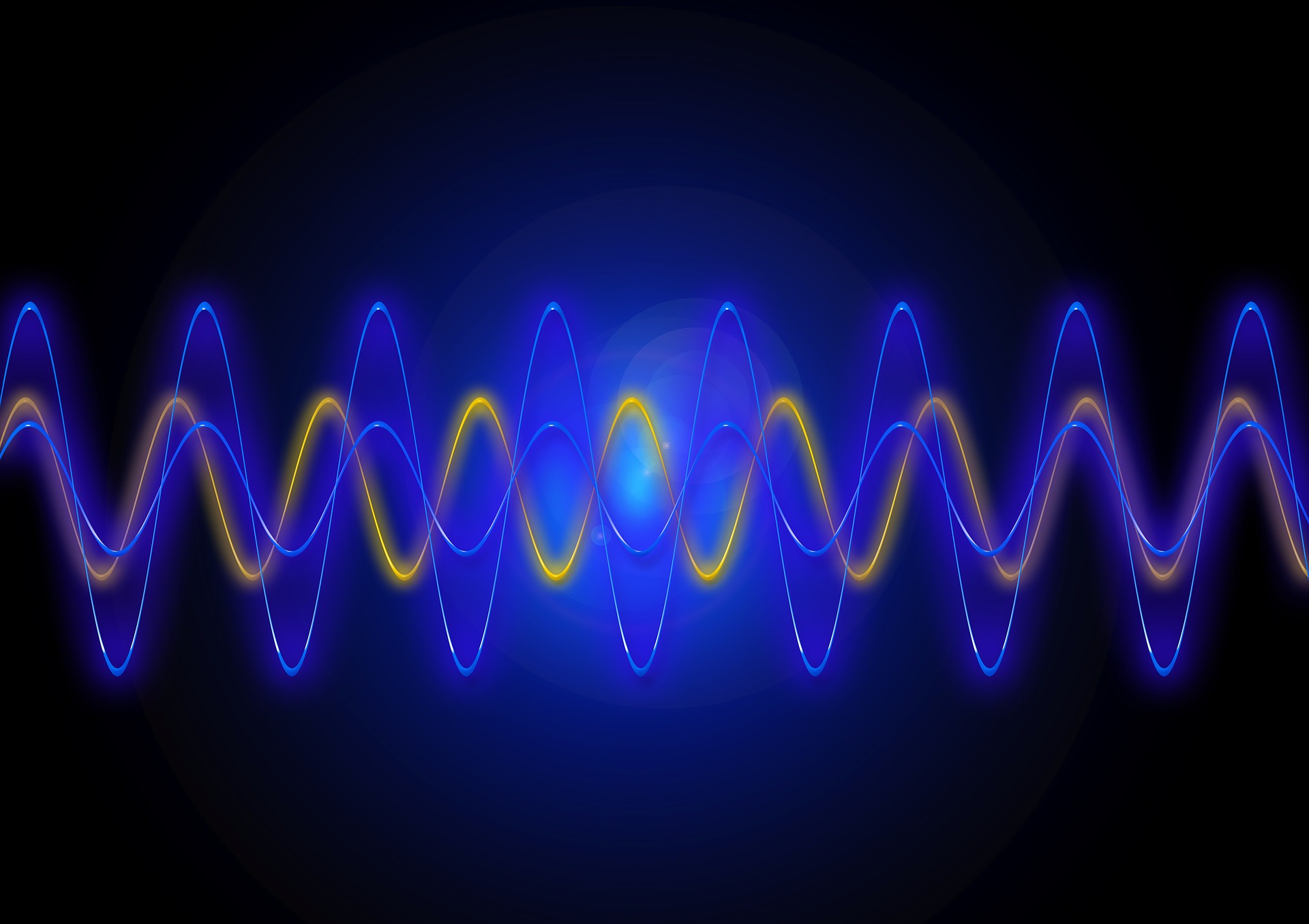
- Read more about BOOSTING ZERO-SHOT NODE CLASSIFICATION VIA DEPENDENCY CAPTURE AND DISCRIMINATIVE FEATURE LEARNING
- Log in to post comments
Zero-shot node classification aims to predict nodes belonging to novel classes that have not been seen in the training. Existing studies focus on transferring knowledge from seen classes to unseen classes, which have achieved good performance in most cases. However, they do not fully leverage the relationships between nodes and overlook the issue of domain bias, affecting overall performance. In this paper, we propose a novel dependency capture and discriminative feature learning (DCDFL) model for zero-shot node classification.
- Categories: