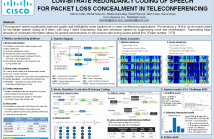
- Read more about Low-bitrate redundancy coding of speech for packet loss concealment in teleconferencing
- Log in to post comments
conferencing applications. We introduced a novel neural codec for low-bitrate speech coding at 6 kbit/s, with long 1 kbit/s redundancy, that also enhances speech by suppressing noise and reverberation. Transmitting large amounts of redundant information allows for speech reconstruction on the receiver side during severe packet loss – see ICASSP paper ID 7175: “Ultra low bitrate loss resilient neural speech enhancing codec”.
- Categories:
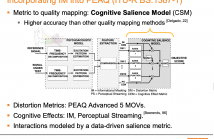
- Read more about An Improved Metric of Informational Masking for Perceptual Audio Quality Measurement
- Log in to post comments
Perceptual audio quality measurement systems algorithmically analyze the output of audio processing systems to estimate possible perceived quality degradation using perceptual models of human audition. In this manner, they save the time and resources associated with the design and execution of listening tests (LTs). Models of disturbance audibility predicting peripheral auditory masking in quality measurement systems have considerably increased subjective quality prediction performance of signals processed by perceptual audio codecs.
- Categories:
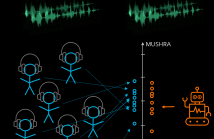
- Read more about Generative Machine Listener
- Log in to post comments
We show how a neural network can be trained on individual intrusive listening test scores to predict a distribution of scores for each pair of reference and coded input stereo or binaural signals. We nickname this method the Generative Machine Listener (GML), as it is capable of generating an arbitrary amount of simulated listening test data. Compared to a baseline system using regression over mean scores, we observe lower outlier ratios (OR) for the mean score predictions, and obtain easy access to the prediction of confidence intervals (CI).
- Categories:
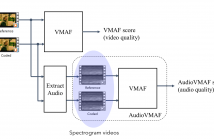
- Read more about AudioVMAF: Audio Quality Prediction with VMAF
- Log in to post comments
Video Multimethod Assessment Fusion (VMAF) [1],[2],[3] is a popular tool in the industry for measuring coded video quality. In this study, we propose an auditory-inspired frontend in existing VMAF for creating videos of reference and coded spectrograms, and extended VMAF for measuring coded audio quality. We name our system AudioVMAF. We demonstrate that image replication is capable of further enhancing prediction accuracy, especially when band-limited anchors are present.
- Categories:
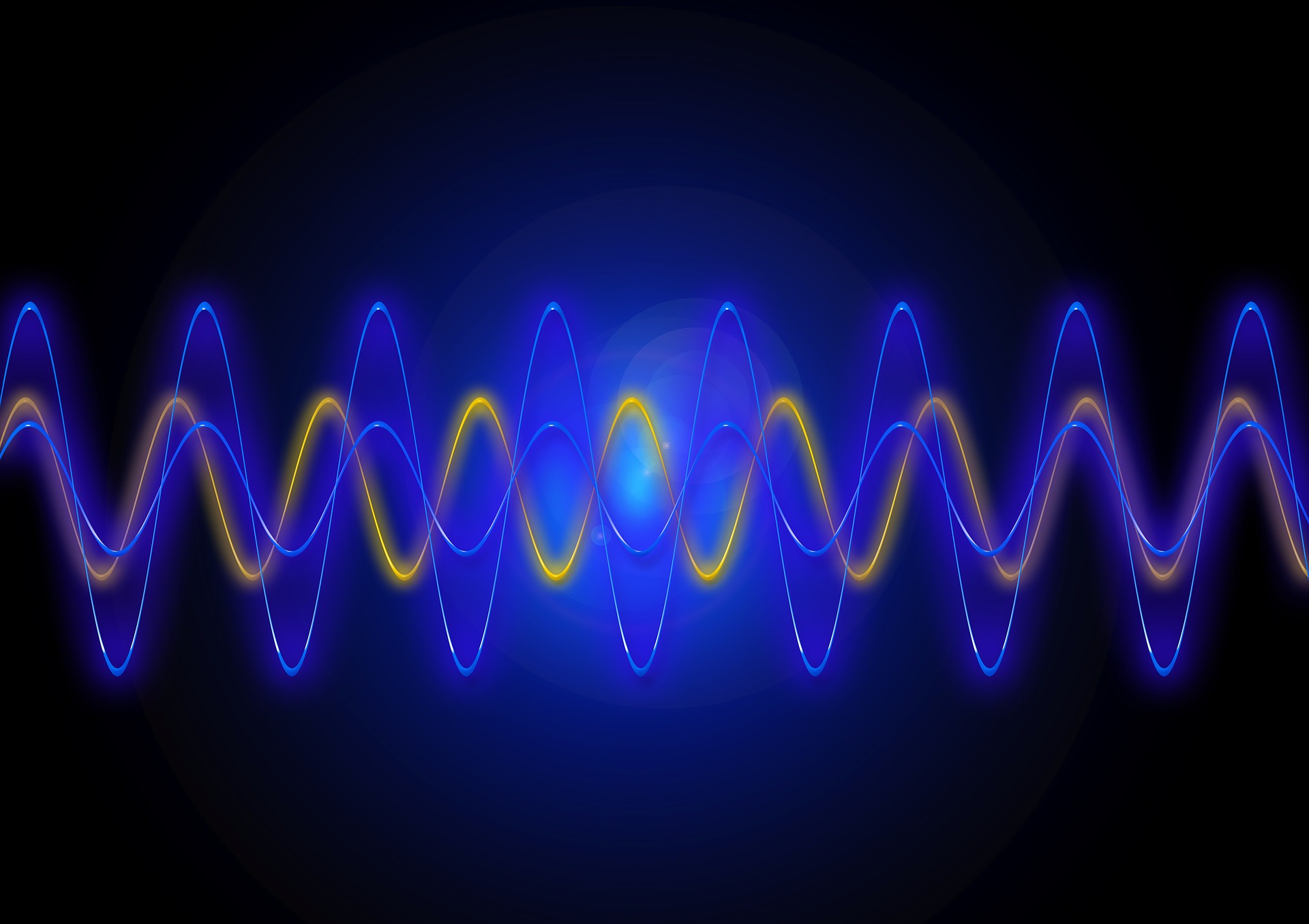
- Read more about Stereo InSE-NET: Stereo Audio Quality Predictor Transfer Learned from Mono InSE-NET
- Log in to post comments
This is a presentation of the paper (http://www.aes.org/e-lib/browse.cfm?elib=21902), presented at the 153rd Audio Engineering Society Convention (https://sched.co/1CK3O).
- Categories:
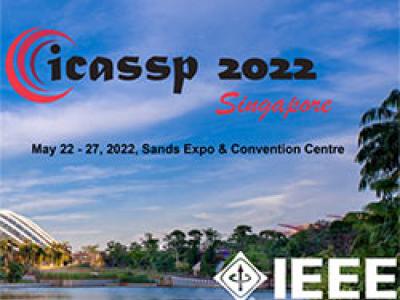
- Read more about End-to-End Neural Speech Coding for Real-Time Communications
- Log in to post comments
- Categories:
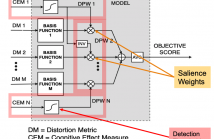
- Read more about A Data-Driven Cognitive Salience Model for Objective Perceptual Audio Quality Assessment
- Log in to post comments
Objective audio quality assessment systems often use perceptual models to predict the subjective quality scores of processed signals, as reported in listening tests. Most systems map different metrics of perceived degradation into a single quality score predicting subjective quality. This requires a quality mapping stage that is informed by real listening test data using statistical learning (\iec a data-driven approach) with distortion metrics as input features.
- Categories:
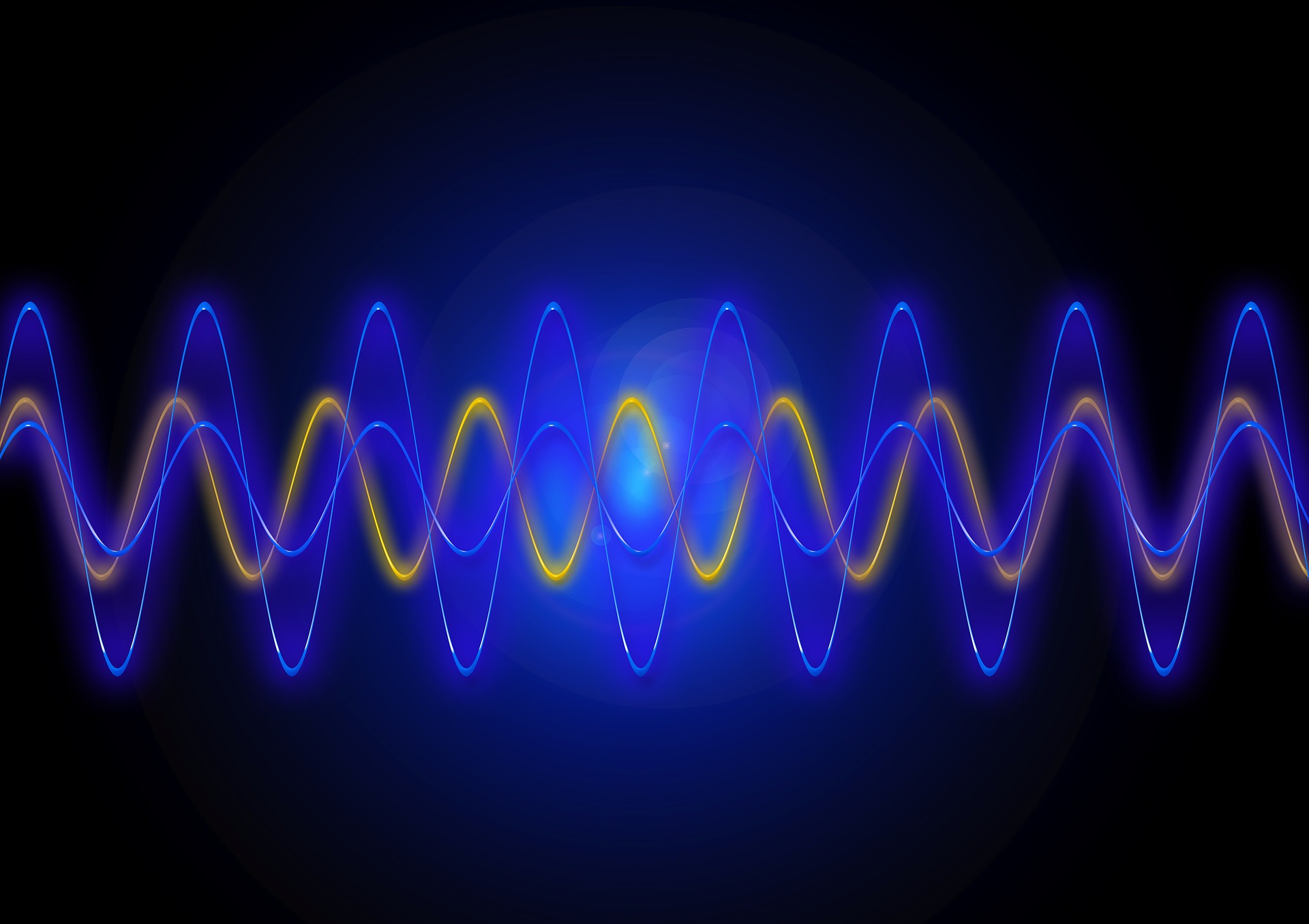
- Read more about InSE-NET: A Perceptually Coded Audio Quality Model based on CNN
- Log in to post comments
This is a presentation of the paper (http://www.aes.org/e-lib/browse.cfm?elib=21478), presented virtually at the 151st Audio Engineering Society Convention (https://sched.co/mIhg).
- Categories:
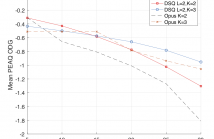
- Read more about Low Delay Robust Audio Coding by Noise Shaping, Fractional Sampling, and Source Prediction
- Log in to post comments
It was recently shown that the combination of source prediction, two-times oversampling, and noise shaping, can be used to obtain a robust (multiple-description) audio coding frame- work for networks with packet loss probabilities less than 10%. Specifically, it was shown that audio signals could be encoded into two descriptions (packets), which were separately sent over a communication channel. Each description yields a desired performance by itself, and when they are combined, the performance is improved.
- Categories:
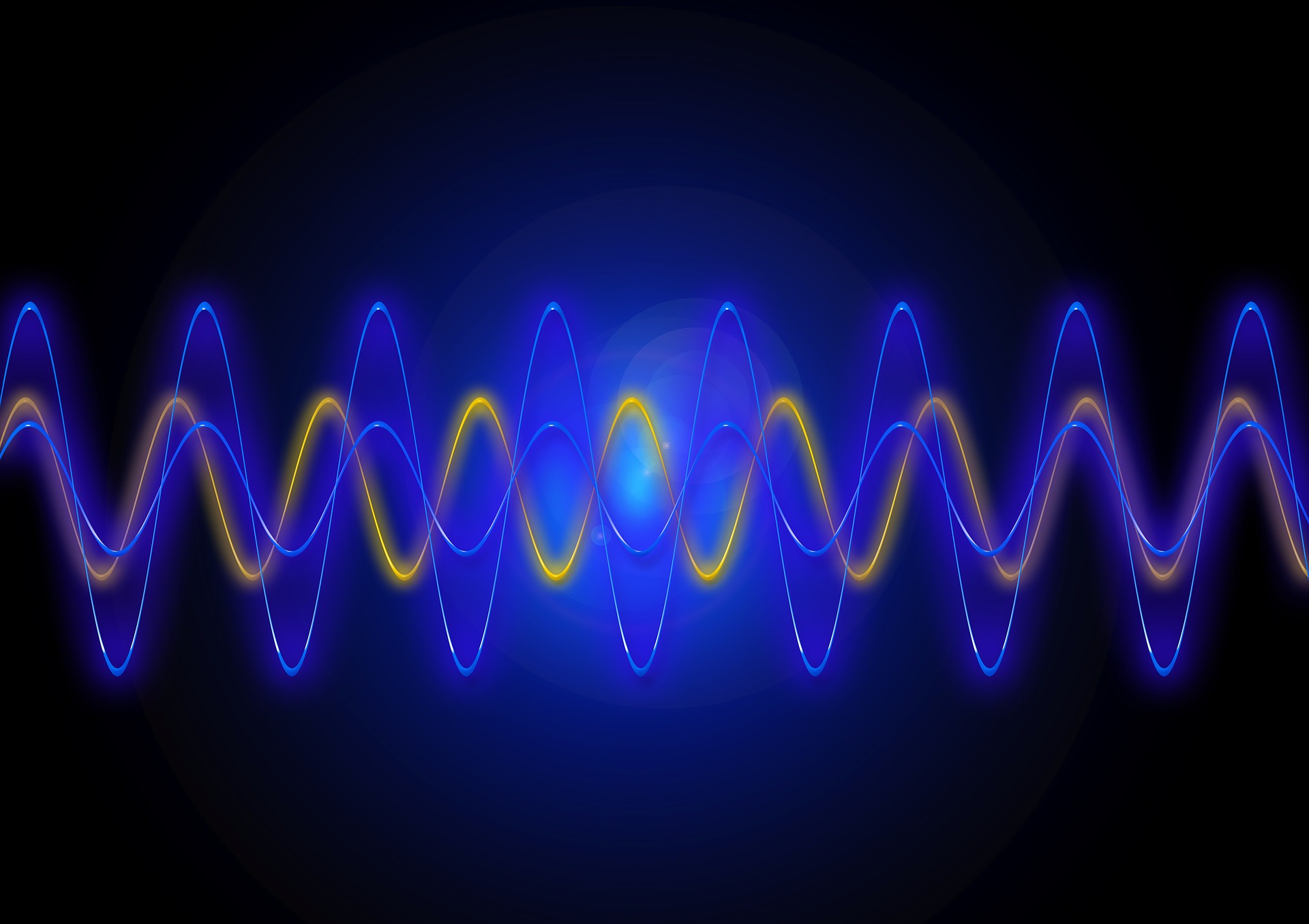
- Read more about Pruning of an Audio Enhancing Deep Generative Neural Network
- Log in to post comments
This is a presentation of the paper (http://www.aes.org/e-lib/browse.cfm?elib=20769), presented virtually at the 148th Audio Engineering Society Convention (https://www.eventscribe.com/2020/VirtualVienna/).
- Categories: