- Read more about OBJECTIVE ASSESSMENT OF ENVELOPE ENHANCEMENT ALGORITHMS FOR ASSISTIVE HEARING DEVICES
- Log in to post comments
Individuals with auditory neuropathy spectrum disorder (ANSD) or auditory processing disorders (APDs) often suffer from temporal processing deficits leading to degraded speech perception. The situation becomes worse in the presence of background noise. Evidence exists that the exaggeration of speech envelope may enhance intelligibility, although a comprehensive evaluation of envelope enhancement algorithms is lacking.
- Categories:
- Read more about Heart Sound Segmentation using Switching Linear Dynamical Models
- Log in to post comments
Localization of exact positions of the fundamental heart sounds (FHS) is an essential step towards automatic analysis of heart sound phonocardiogram (PCG) recordings, the automatic segmentation allows for data-driven classification of heart pathological events. Current approach using probabilistic models such as hidden Markov models (HMMs) has improved accuracy of heart sound segmentation.
- Categories:
- Read more about ENHANCED SLEEP SPINDLE DETECTOR BASED ON THE FUJIMORI METHOD
- Log in to post comments
Afternoon sleepiness in daily life reduces arousal level, performance, and so on. It has been cleared that short naps are effective to cancel the sleepiness. Sleep stage 2 is one of important factors about sleeping especially in short time nap. Sleep spindles are especially important hallmarks of sleep stage 2. Therefore, it is necessary to find a spindle for analysis in sleep stage 2.
- Categories:
- Read more about QUALITY ASSESSMENT OF MPEG-4 AVC/H.264 AND HEVC COMPRESSED VIDEO IN A TELEMEDICINE CONTEXT
- Log in to post comments
- Categories:
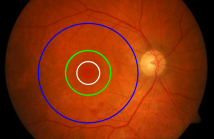
- Read more about AUTOMATIC DELINEATION OF MACULAR REGIONS BASED ON A LOCALLY DEFINED CONTRAST FUNCTION
- Log in to post comments
We consider the problem of fovea segmentation and develop
a technique for delineation of macular regions based on the
active-disc formalism that we recently introduced. The outlining
problem is posed as one of the optimization of a locally
defined contrast function using gradient-ascent maximization
with respect to the affine transformation parameters
that characterize the active disc. For automatic localization
of the fovea and initialization of the active disc, we
use the directional-derivative-based matched filter. We report
- Categories:
- Read more about CSMSDL: A Common Sequential Dictionary Learning Algorithm for Multi-Subject fMRI Data Sets Analysis
- Log in to post comments
Sequential dictionary learning algorithms has gained widespread acceptance in functional magnetic resonance imaging (fMRI) data analysis. However, many problems in fMRI data analysis involve the analysis of multiple-subject fMRI data sets and the existing algorithms do not extend naturally to this case. In this paper we propose an algorithm dedicated to multiple-subject fMRI data analysis. The algorithm is named SMSDL for sequential multi-subject dictionary learning and differs from existing dictionary learning algorithms in its dictionary update stage.
- Categories:
- Read more about RESPIRATORY AIRFLOW ESTIMATION FROM LUNG SOUNDS BASED ON REGRESSION
- Log in to post comments
poster.pdf

- Categories:
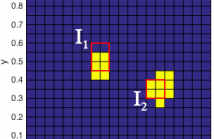
While convex optimization for low-light imaging has received some attention by the imaging community, non-convex optimization techniques for photon-limited imaging are still in their nascent stages. In this thesis, we developed a stage-based non-convex approach to recover high-resolution sparse signals from low-dimensional measurements corrupted by Poisson noise. We incorporate gradient-based information to construct a sequence of quadratic subproblems with an $\ell_p$-norm ($0 \leq p < 1$) penalty term to promote sparsity.
PhDForum.pdf

- Categories:
- Read more about Reduction of Necessary Data Rate for Neural Data Through Exponential and Sinusoidal Spline Decomposition using the Finite Rate of Innovation Framework
- Log in to post comments
The sampling of neural signals plays an important role in modern neuroscience, especially for prosthetics. However, due to hardware and data rate constraints, only spike trains can get recovered reliably. State of the art prosthetics can still achieve impressive results, but to get higher resolutions the used data rate needs to be reduced. In this paper, this is done by expressing the data with exponential and sinusoidal splines.
FRIVortrag.pdf

FRIVortrag.pdf

- Categories:
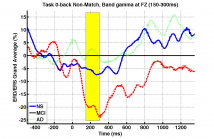
- Read more about EVENT-RELATED SYNCHRONISATION RESPONSES TO N-BACK MEMORY TASKS DISCRIMINATE BETWEEN HEALTHY AGEING, MILD COGNITIVE IMPAIRMENT, AND MILD ALZHEIMER’S DISEASE
- Log in to post comments
In this study we investigate whether or not event-related (de)synchronisation (ERD/ERS) can be used to differenti- ate between 27 healthy elderly, 21 subjects diagnosed with amnestic mild cognitive impairment (aMCI) and 16 mild Alzheimer’s disease (AD) patients. Using 32-channel EEG recordings, we measured ERD responses to a three-level vi- sual N-back task (N = 0, 1, 2) on the well-known delta, theta, alpha, beta and gamma bands.
- Categories: