- Read more about FAST AND EFFICIENT REJECTION OF BACKGROUND WAVEFORMS IN INTERICTAL EEG
- Log in to post comments
- Categories:
- Read more about EPILEPTIFORM SPIKE TEMPLATE SELECTION USING DYNAMIC TIME WARPING AND AFFINITY PROPAGATION
- Log in to post comments
- Categories:
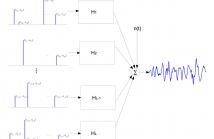
- Read more about TRANSIENT MODEL OF EEG USING GINI INDEX-BASED MATCHING PURSUIT
- Log in to post comments
We introduce a novel, transient model for the electroencephalogram (EEG) as the noisy addition of linear filters responding to trains of delta functions. We set the synthesis part as a parameter-tuning problem and obtain synthetic EEG-like data that visually resembles brain activity in the time and frequency domains. For the analysis counterpart, we use sparse approximation to decompose the signal in relevant events via Matching Pursuit.
- Categories:
- Read more about EPILEPTIFORM SPIKE DETECTION VIA CONVOLUTIONAL NEURAL NETWORKS
- Log in to post comments
- Categories:
- Read more about Brain Functional Connectivity Analysis Using Mutual Information
- Log in to post comments
- Categories:
- Read more about Brain Functional Connectivity Analysis Using Mutual Information
- Log in to post comments
- Categories:
- Read more about Motor Imagery Classification Using Multiresolution Analysis and Sparse Representation of EEG Signals
- Log in to post comments
- Categories:
- Read more about Subject Independent Affective States Classification Using EEG Signals
- Log in to post comments
Affective states classification has become an important part of the Human-Computer Interface (HCI) study. In recent years, studies of physiological signals, such as ECG, GSR and EEG on affective expression have shown very promising results. In this study, we carried out two experiments to better understand the neurological expression of emotions through the use of EEG signals. In particular, we carried out a subject- independent affective states classification study using narrow- band spectral power of the EEG signals.
- Categories:
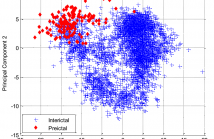
- Read more about Seizure Prediction using Hilbert Huang Transform on Field Programmable Gate Array
- Log in to post comments
The Hilbert Huang Transform (HHT) has been used extensively in the time-frequency analysis of electroencephalography (EEG) signals and Brain-Computer Interfaces. Most studies utilizing the HHT for extracting features in seizure prediction have used intracranial EEG recordings. Invasive implants in the cortex have unknown long term consequences and pose the risk of complications during surgery. This added risk dimension makes them unsuitable for continuous monitoring as would be the requirement in a Body Area Network.
- Categories: