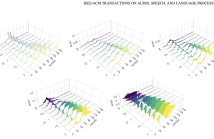
- Read more about Interpreting Intermediate Convolutional Layers of Generative CNNs Trained on Waveforms
- Log in to post comments
This paper presents a technique to interpret and visualize intermediate layers in generative CNNs trained on raw speech data in an unsupervised manner. We argue that averaging over feature maps after ReLU activation in each transpose convolutional layer yields interpretable time-series data. This technique allows for acoustic analysis of intermediate layers that parallels the acoustic analysis of human speech data: we can extract F0, intensity, duration, formants, and other acoustic properties from intermediate layers in order to test where and how CNNs encode various types of information.
- Categories:
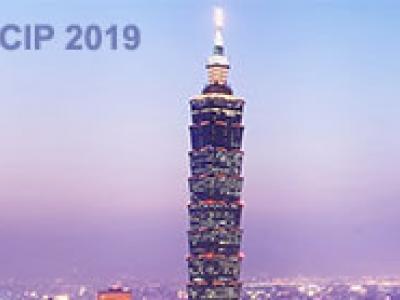
- Read more about Temporal Interframe Pattern Analysis for Static and Dynamic Hand Gesture Recognition
- Log in to post comments
Hand gesture, a common non-verbal language, is being studied for Human Computer Interaction. Hand gestures can be categorized as static hand gestures and dynamic hand gestures. In recent years, effective approaches have been applied to hand gesture recognition.
- Categories:
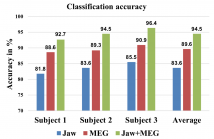
- Read more about OVERT SPEECH RETRIEVAL FROM NEUROMAGNETIC SIGNALS USING WAVELETS AND ARTIFICIAL NEURAL NETWORKS
- Log in to post comments
Speech production involves the synchronization of neural activity between the speech centers of the brain and the oralmotor system, allowing for the conversion of thoughts into
- Categories:
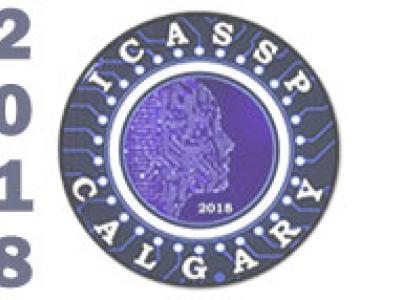
- Read more about Cognitive Analysis of Working Memory Load from EEG, by a Deep Recurrent Neural Network
- Log in to post comments
One of the common modalities for observing mental activity is electroencephalogram (EEG) signals. However, EEG recording is highly susceptible to various sources of noise and to inter-subject differences. In order to solve these problems, we present a deep recurrent neural network (RNN) architecture to learn robust features and predict the levels of the cognitive load from EEG recordings. Using a deep learning approach, we first transform the EEG time series into a sequence of multispectral images which carries spatial information.
Kuanar_ICASSP-2018.pdf

- Categories:
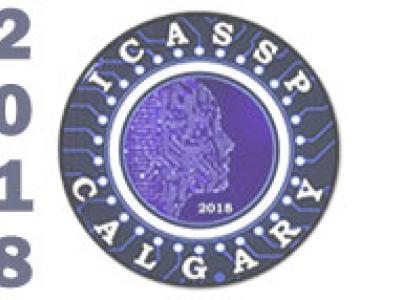
- Read more about CONVOLUTIONAL NEURAL NETWORK APPROACH FOR EEG-BASED EMOTION RECOGNITION USING BRAIN CONNECTIVITY AND ITS SPATIAL INFORMATION
- Log in to post comments
Emotion recognition based on electroencephalography (EEG) has received attention as a way to implement human-centric
services. However, there is still much room for improvement, particularly in terms of the recognition accuracy. In this paper, we propose a novel deep learning approach using convolutional neural networks (CNNs) for EEG-based emotion recognition. In particular, we employ brain connectivity features that have not been used with deep learning models in
- Categories: