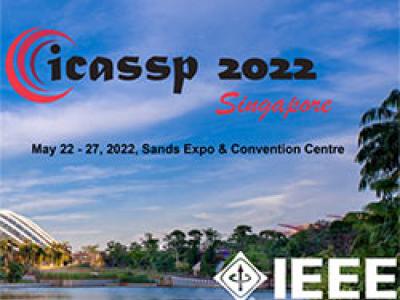
- Read more about Learning task-specific representation for Video anomaly detection with spatial-temporal attention
- Log in to post comments
The automatic detection of abnormal events in surveillance videos with weak supervision has been formulated as a multiple instance learning task, which aims to localize the clips containing abnormal events temporally with the video-level labels. However, most existing methods rely on the features extracted by the pre-trained action recognition models, which are not discriminative enough for video anomaly detection.
- Categories:
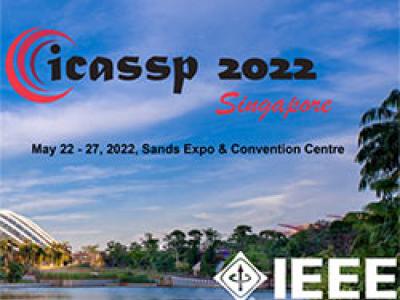
- Read more about CROSS-EPOCH LEARNING FOR WEAKLY SUPERVISED ANOMALY DETECTION IN SURVEILLANCE VIDEOS
- Log in to post comments
Weakly Supervised Anomaly Detection (WSAD) in surveillance videos is a complex task since usually only video-level annotations are available. Previous work treated it as a regression problem by giving different scores on normal and anomaly events. However, the widely used mini-batch training strategy may suffer from the data imbalance between these two types of events, which limits the model’s performance. In this work, a cross-epoch learning (XEL) strategy associated with a hard instance bank (HIB) is proposed to introduce additional information from previous training epochs.
- Categories:
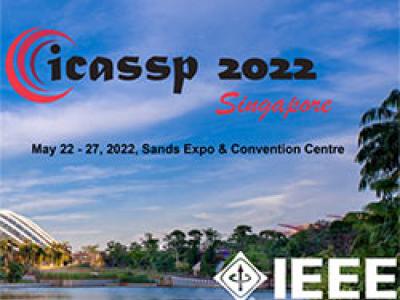
- Read more about Poster for ICASSP 2022 IVMSP-15.1
- Log in to post comments
Poster.pdf

- Categories:
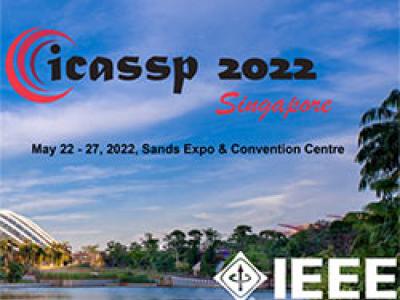
- Read more about Presentation Slides for ICASSP 2022 IVMSP-15.1
- Log in to post comments
- Categories:
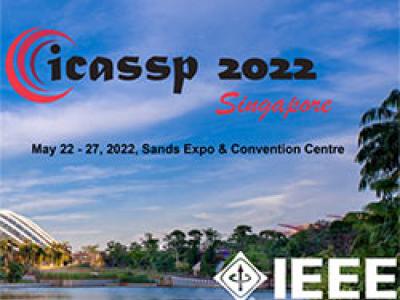
- Read more about Camera Calibration through Camera Projection Loss
- Log in to post comments
Camera calibration is a necessity in various tasks including 3D reconstruction, hand-eye coordination for a robotic interaction, autonomous driving, etc. In this work we propose a novel method to predict extrinsic (baseline, pitch, and translation), intrinsic (focal length and principal point offset) parameters using an image pair. Unlike existing methods, instead of designing an end-to-end solution, we proposed a new representation that incorporates camera model equations as a neural network in a multi-task learning framework.
- Categories:
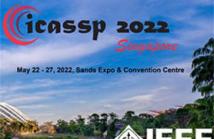
- Read more about HIRL: Hybrid Image Restoration based on Hierarchical Deep Reinforcement Learning via Two-Step Analysis
- Log in to post comments
- Categories:
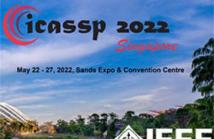
- Read more about JE2Net: Joint Exploitation and Exploration in Reinforcement Learning Based Image Restoration
- Log in to post comments
- Categories:
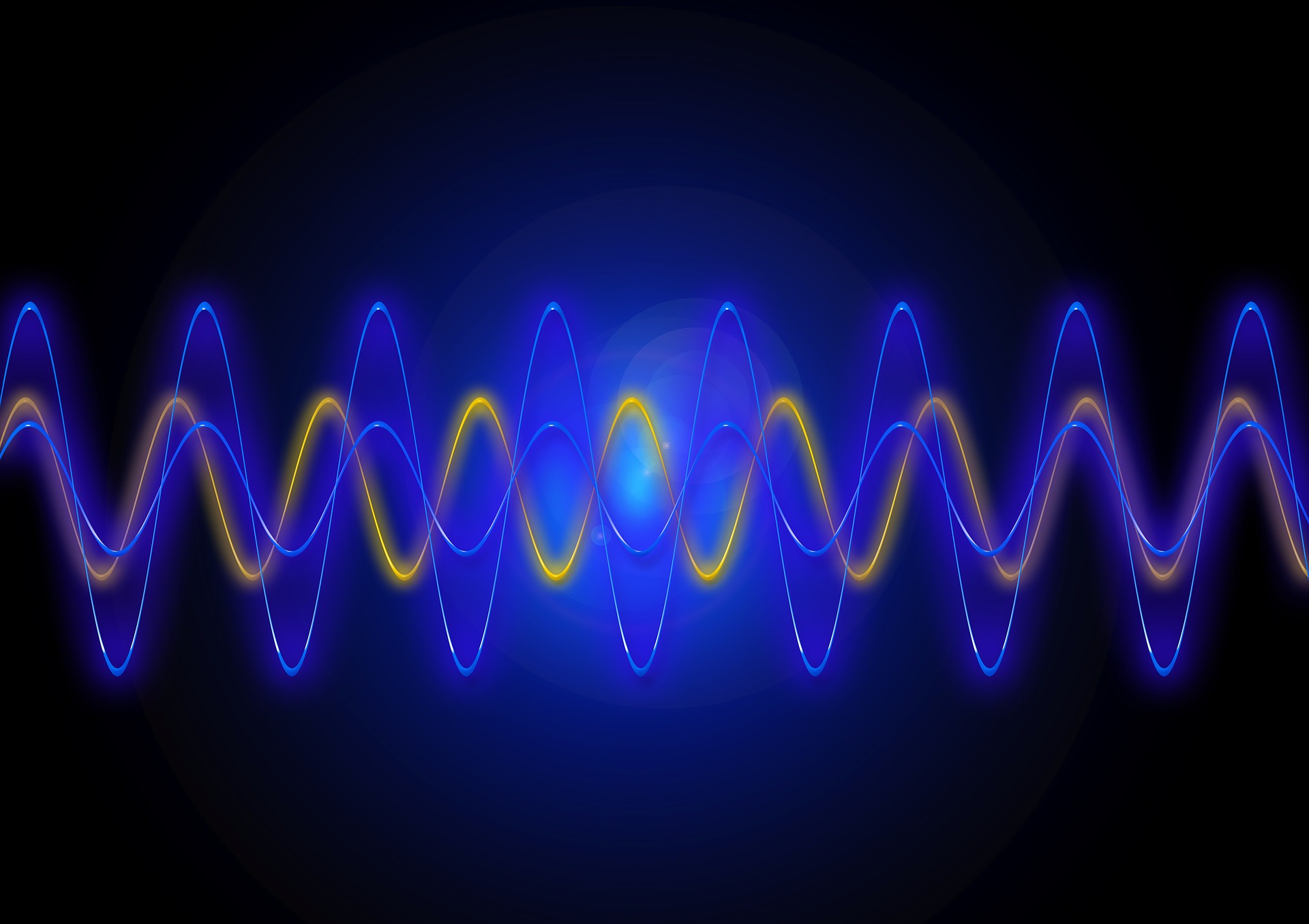
- Read more about Genre-Conditioned Long-Term 3D Dance Generation Driven by Music
- Log in to post comments
Poster.pdf

- Categories:
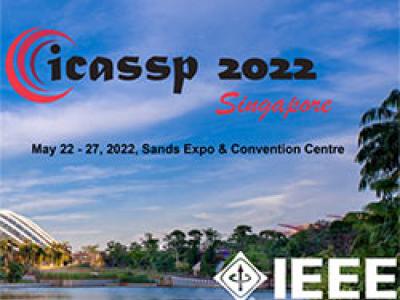
- Read more about Compression-aware Projection with Greedy Dimension Reduction for Activations
- Log in to post comments
Convolutional neural networks (CNNs) achieve remarkable performance in a wide range of fields. However, intensive memory access of activations introduces considerable energy consumption, impeding deployment of CNNs on resource-constrained edge devices. Existing works in activation compression propose to transform feature maps for higher compressibility, thus enabling dimension reduction. Nevertheless, in the case of aggressive dimension reduction, these methods lead to severe accuracy drop.
- Categories:
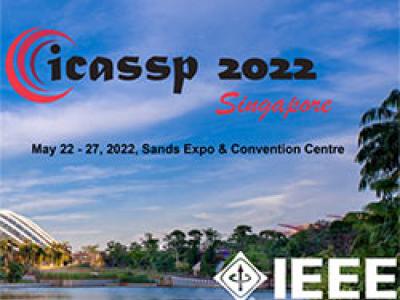
- Read more about Presentation Slides of TOWARDS CONTROLLABLE AND PHYSICAL INTERPRETABLE UNDERWATER SCENE SIMULATION
- Log in to post comments
slides.pptx

- Categories: