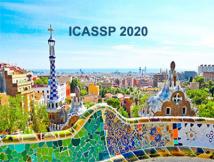
ICASSP is the world’s largest and most comprehensive technical conference focused on signal processing and its applications. The ICASSP 2020 conference will feature world-class presentations by internationally renowned speakers, cutting-edge session topics and provide a fantastic opportunity to network with like-minded professionals from around the world. Visit website.
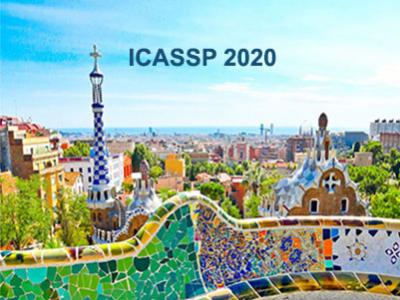
- Read more about Lightweight V-Net for Liver Segmentation
- Log in to post comments
The V-Net based 3D fully convolutional neural networks have been widely used in liver volumetric data segmentation. However, due to the large number of parameters of these networks, 3D FCNs suffer from high computational cost and GPU memory usage. To address these issues, we design a lightweight V-Net (LV-Net) for liver segmentation in this paper. The proposed network makes two contributions. The first is that we design an inverted residual bottleneck block (IRB block) and a 3D average pooling block and apply them to the proposed LV-Net.
- Categories:
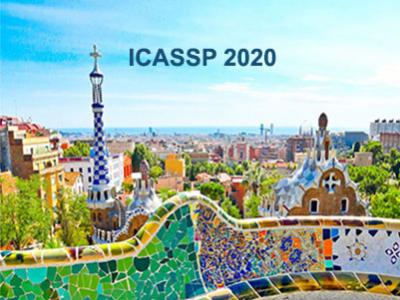
- Read more about Exploration Methodology for BTI-Induced Failures on RRAM-Based Edge AI Systems
- Log in to post comments
Resistive switching memory technologies (RRAM) are seen by most of the scientific community as an enabler for Edge-level applications such as embedded deep Learning, AI or signal processing of audio and video signals. However, going beyond a ``simple'' replacement of eFlash in micro-controller and introducing RRAM inside the memory hierarchy is not a straightforward move. Indeed, integrating a RRAM technology inside the cache hierarchy requires higher endurance requirement than for eFlash replacement, and thus necessitates relaxed programming conditions.
ICASSP_VF.pdf

- Categories:
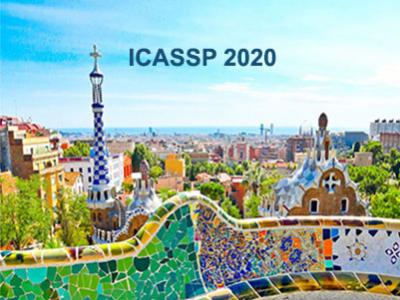
- Read more about Performance Bounds for Displaced Sensor Automotive Radar Imaging
- Log in to post comments
In automotive radar imaging, displaced sensors offer improvement in localization accuracy by jointly processing the data acquired from multiple radar units, each of which may have limited individual resources. In this paper, we derive performance bounds on the estimation error of target parameters processed by displaced sensors that correspond to several independent radars mounted at different locations on the same vehicle. Unlike previous studies, we do not assume a very accurate time synchronization among the sensors.
- Categories:
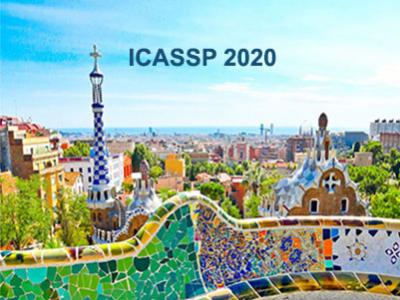
- Read more about Effectiveness of random deep feature selection for securing image manipulation detectors against adversarial examples
- Log in to post comments
We investigate if the random feature selection approach proposed in [1] to improve the robustness of forensic detectors to targeted attacks, can be extended to detectors based on deep learning features. In particular, we study the transferability of adversarial examples targeting an original CNN image manipulation detector to other detectors (a fully connected neural network and a linear SVM) that rely on a random subset of the features extracted from the flatten layer of the original network.
- Categories:
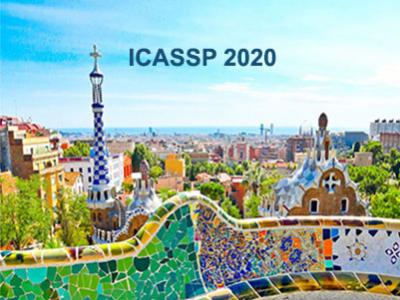
- Read more about Effectiveness of Random Deep Feature Selection for securing image manipulation detectors against adversarial examples
- Log in to post comments
We investigate if the random feature selection approach proposed in [1] to improve the robustness of forensic detectors to targeted attacks, can be extended to detectors based on deep learning features. In particular, we study the transferability of adversarial examples targeting an original CNN image manipulation detector to other detectors (a fully connected neural network and a linear SVM) that rely on a random subset of the features extracted from the flatten layer of the original network.
- Categories: