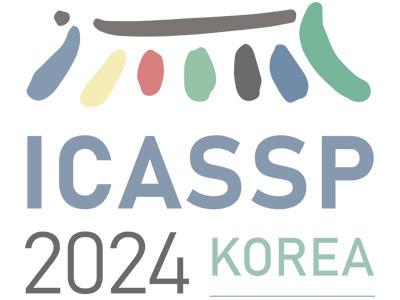
- Read more about Speech Emotion Recognition with Distilled Prosodic and Linguistic Affect Representations
- Log in to post comments
We propose EmoDistill, a novel speech emotion recognition (SER) framework that leverages cross-modal knowledge distillation during training to learn strong linguistic and prosodic representations of emotion from speech. During inference, our method only uses a stream of speech signals to perform unimodal SER thus reducing computation overhead and avoiding run-time transcription and prosodic feature extraction errors. During training, our method distills information at both embedding and logit levels from a pair of pre-trained Prosodic and Linguistic teachers that are fine-tuned for SER.
- Categories:
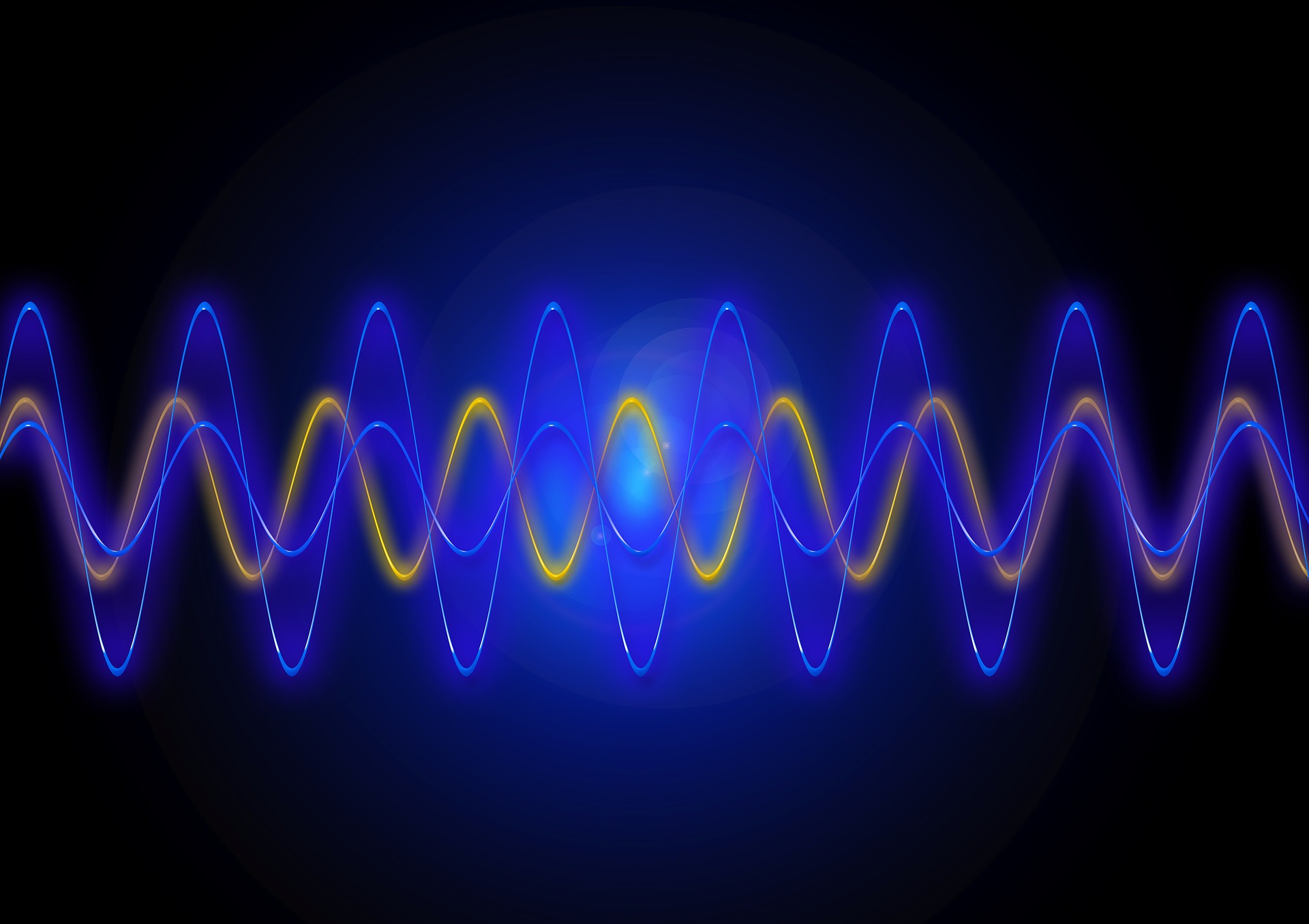
- Read more about FRAME-LEVEL EMOTIONAL STATE ALIGNMENT METHOD FOR SPEECH EMOTION RECOGNITION
- Log in to post comments
Speech emotion recognition (SER) systems aim to recognize human emotional state during human-computer interaction. Most existing SER systems are trained based on utterance-level labels. However, not all frames in an audio have affective states consistent with utterance-level label, which makes it difficult for the model to distinguish the true emotion of the audio and perform poorly. To address this problem, we propose a frame-level emotional state alignment method for SER.
- Categories:
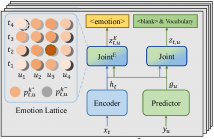
- Read more about Emotion Neural Transducer for Fine-Grained Speech Emotion Recognition
- Log in to post comments
The mainstream paradigm of speech emotion recognition (SER) is identifying the single emotion label of the entire utterance. This line of works neglect the emotion dynamics at fine temporal granularity and mostly fail to leverage linguistic information of speech signal explicitly. In this paper, we propose Emotion Neural Transducer for fine-grained speech emotion recognition with automatic speech recognition (ASR) joint training. We first extend typical neural transducer with emotion joint network to construct emotion lattice for fine-grained SER.
- Categories:
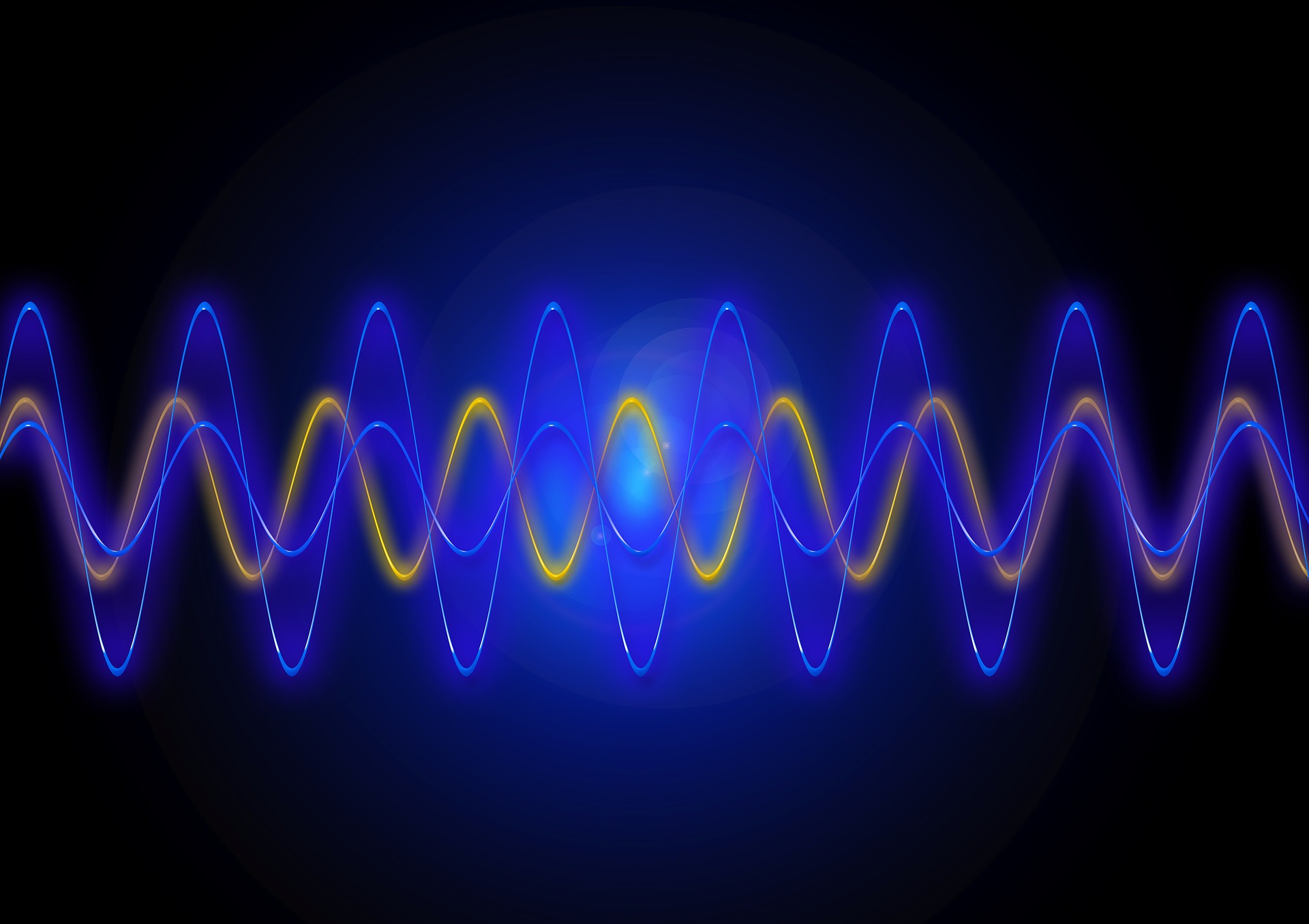
- Read more about HUBERTOPIC: ENHANCING SEMANTIC REPRESENTATION OF HUBERT THROUGH SELF-SUPERVISION UTILIZING TOPIC MODEL
- Log in to post comments
Recently, the usefulness of self-supervised representation learning (SSRL) methods has been confirmed in various downstream tasks. Many of these models, as exemplified by HuBERT and WavLM, use pseudo-labels generated from spectral features or the model’s own representation features. From previous studies, it is known that the pseudo-labels contain semantic information. However, the masked prediction task, the learning criterion of HuBERT, focuses on local contextual information and may not make effective use of global semantic information such as speaker, theme of speech, and so on.
- Categories:
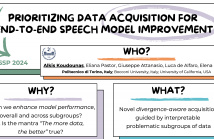
- Read more about PRIORITIZING DATA ACQUISITION FOR END-TO-END SPEECH MODEL IMPROVEMENT
- Log in to post comments
As speech processing moves toward more data-hungry models, data selection and acquisition become crucial to building better systems. Recent efforts have championed quantity over quality, following the mantra ``The more data, the better.''
However, not every data brings the same benefit. This paper proposes a data acquisition solution that yields better models with less data -- and lower cost.
Given a model, a task, and an objective to maximize, we propose a process with three steps. First, we assess the model's baseline performance on the task.
- Categories:
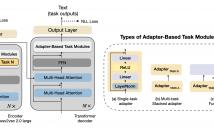
- Read more about An Adapter-Based Unified Model for Multiple Spoken Language Processing Tasks
- Log in to post comments
Self-supervised learning models have revolutionized the field of speech processing. However, the process of fine-tuning these models on downstream tasks requires substantial computational resources, particularly when dealing with multiple speech-processing tasks. In this paper, we explore the potential of adapter-based fine-tuning in developing a unified model capable of effectively handling multiple spoken language processing tasks. The tasks we investigate are Automatic Speech Recognition, Phoneme Recognition, Intent Classification, Slot Filling, and Spoken Emotion Recognition.
suresh_3826.pdf

- Categories:
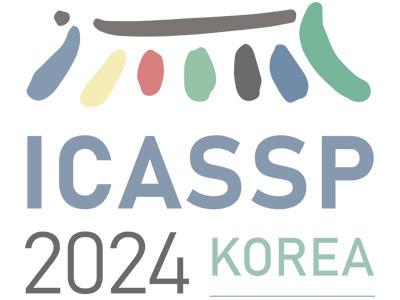
- Read more about S2E: Towards an End-to-End Entity Resolution Solution from Acoustic Signal
- Log in to post comments
Traditional cascading Entity Resolution (ER) pipeline suffers from propagated errors from upstream tasks. We address this is-sue by formulating a new end-to-end (E2E) ER problem, Signal-to-Entity (S2E), resolving query entity mentions to actionable entities in textual catalogs directly from audio queries instead of audio transcriptions in raw or parsed format. Additionally, we extend the E2E Spoken Language Understanding framework by introducing a novel dimension to ER research.
- Categories:
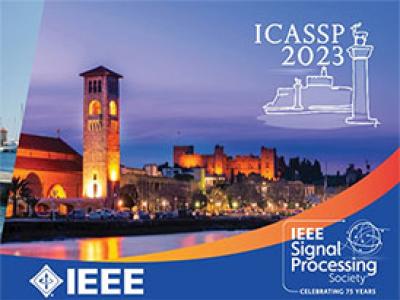
End-to-End Spoken Language Understanding models are generally evaluated according to their overall accuracy, or separately on (a priori defined) data subgroups of interest.
- Categories:
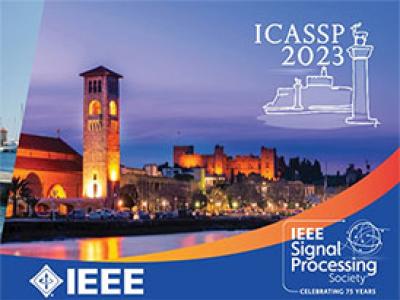
- Read more about MEETING ACTION ITEM DETECTION WITH REGULARIZED CONTEXT MODELING
- Log in to post comments
Meetings are increasingly important for collaborations. Action items in meeting transcripts are crucial for managing post-meeting to-do tasks, which usually are summarized laboriously.
- Categories:
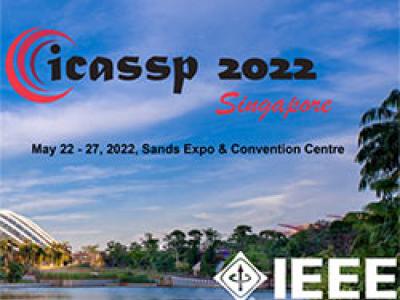
- Read more about TED TALK TEASER GENERATION WITH PRE-TRAINED MODELS
- Log in to post comments
While we have seen significant advances in automatic summarization for text, research on speech summarization is still limited. In this work, we address the challenge of automatically generating teasers for TED talks. In the first step, we create a corpus for automatic summarization of TED and TEDx talks consisting of the talks' recording, their transcripts and their descriptions. The corpus is used to build a speech summarization system for the task. We adapt and combine pre-trained models for automatic speech recognition (ASR) and text summarization using the collected data.
- Categories: