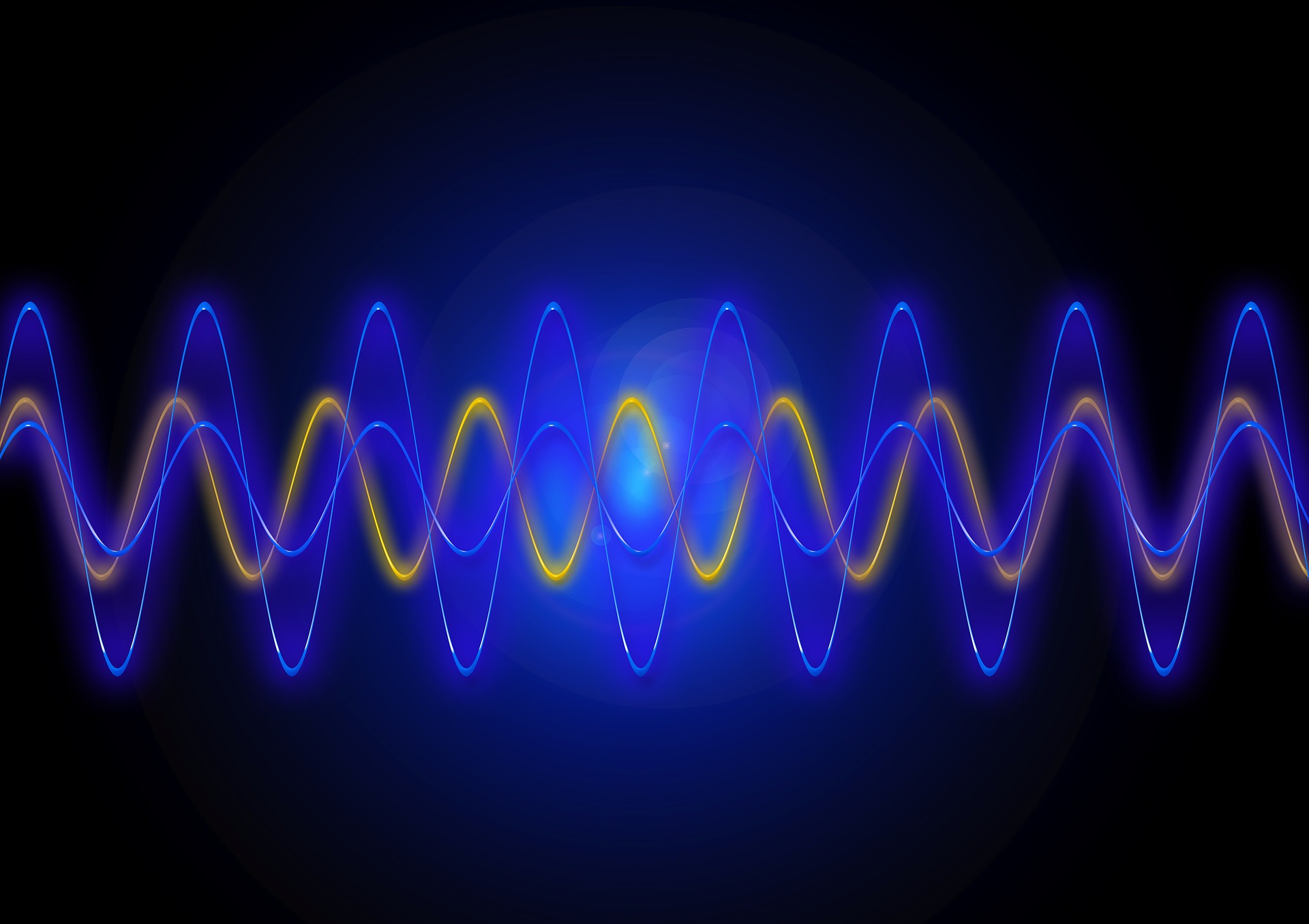
- Read more about PSEUDO STRONG LABELS FOR LARGE SCALE WEAKLY SUPERVISED AUDIO TAGGING
- Log in to post comments
- Categories:
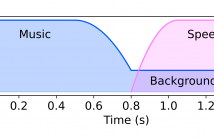
- Read more about ARTIFICIALLY SYNTHESISING DATA FOR AUDIO CLASSIFICATION AND SEGMENTATION TO IMPROVE SPEECH AND MUSIC DETECTION IN RADIO BROADCAST
- Log in to post comments
Segmenting audio into homogeneous sections such as music and speech helps us understand the content of audio. It is useful as a pre-processing step to index, store, and modify audio recordings, radio broadcasts and TV programmes. Deep learning models for segmentation are generally trained on copyrighted material, which cannot be shared. Annotating these datasets is time-consuming and expensive and therefore, it significantly slows down research progress. In this study, we present a novel procedure that artificially synthesises data that resembles radio signals.
- Categories:
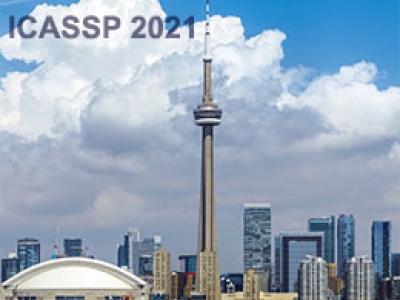
We propose an adapter based multi-domain Transformer based language model (LM) for Transformer ASR. The model consists of a big size common LM and small size adapters. The model can perform multi-domain adaptation with only the small size adapters and its related layers. The proposed model can reuse the full fine-tuned LM which is fine-tuned using all layers of an original model. The proposed LM can be expanded to new domains by adding about 2% of parameters for a first domain and 13% parameters for after second domain.
- Categories:
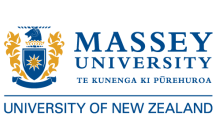
- Read more about DEEPF0: END-TO-END FUNDAMENTAL FREQUENCY ESTIMATION FOR MUSIC AND SPEECH SIGNALS
- Log in to post comments
We propose a novel pitch estimation technique called DeepF0, which leverages the available annotated data to directly learns from the raw audio in a data-driven manner. F0 estimation is important in various speech processing and music information retrieval applications. Existing deep learning models for pitch estimations have relatively limited learning capabilities due to their shallow receptive field. The proposed model addresses this issue by extending the receptive field of a network by introducing the dilated convolutional blocks into the network.
- Categories:
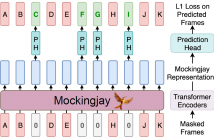
- Read more about Mockingjay: Unsupervised Speech Representation Learning with Deep Bidirectional Transformer Encoders
- Log in to post comments
We present Mockingjay as a new speech representation learning approach, where bidirectional Transformer encoders are pre-trained on a large amount of unlabeled speech. Previous speech representation methods learn through conditioning on past frames and predicting information about future frames. Whereas Mockingjay is designed to predict the current frame through jointly conditioning on both past and future contexts.
- Categories:
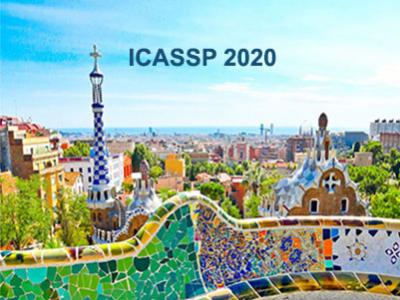
- Read more about TASK-AWARE MEAN TEACHER METHOD FOR LARGE SCALE WEAKLY LABELED SEMI-SUPERVISED SOUND EVENT DETECTION
- Log in to post comments
- Categories:
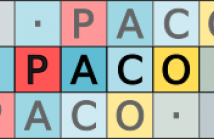
- Read more about PACO and PCO-DCT: Patch Consensus and Its Application To Inpainting
- Log in to post comments
Many signal processing methods break the target signal into overlapping patches, process them separately, and then stitch them back to produce an output. How to merge the resulting patches at the overlaps is central to such methods. We propose a novel framework for this type of problem based on the idea that estimated patches should coincide at the overlaps (consensus), and develop an algorithm for solving the general problem. In particular, an efficient method for projecting patches onto the consensus constraint is presented.
- Categories:
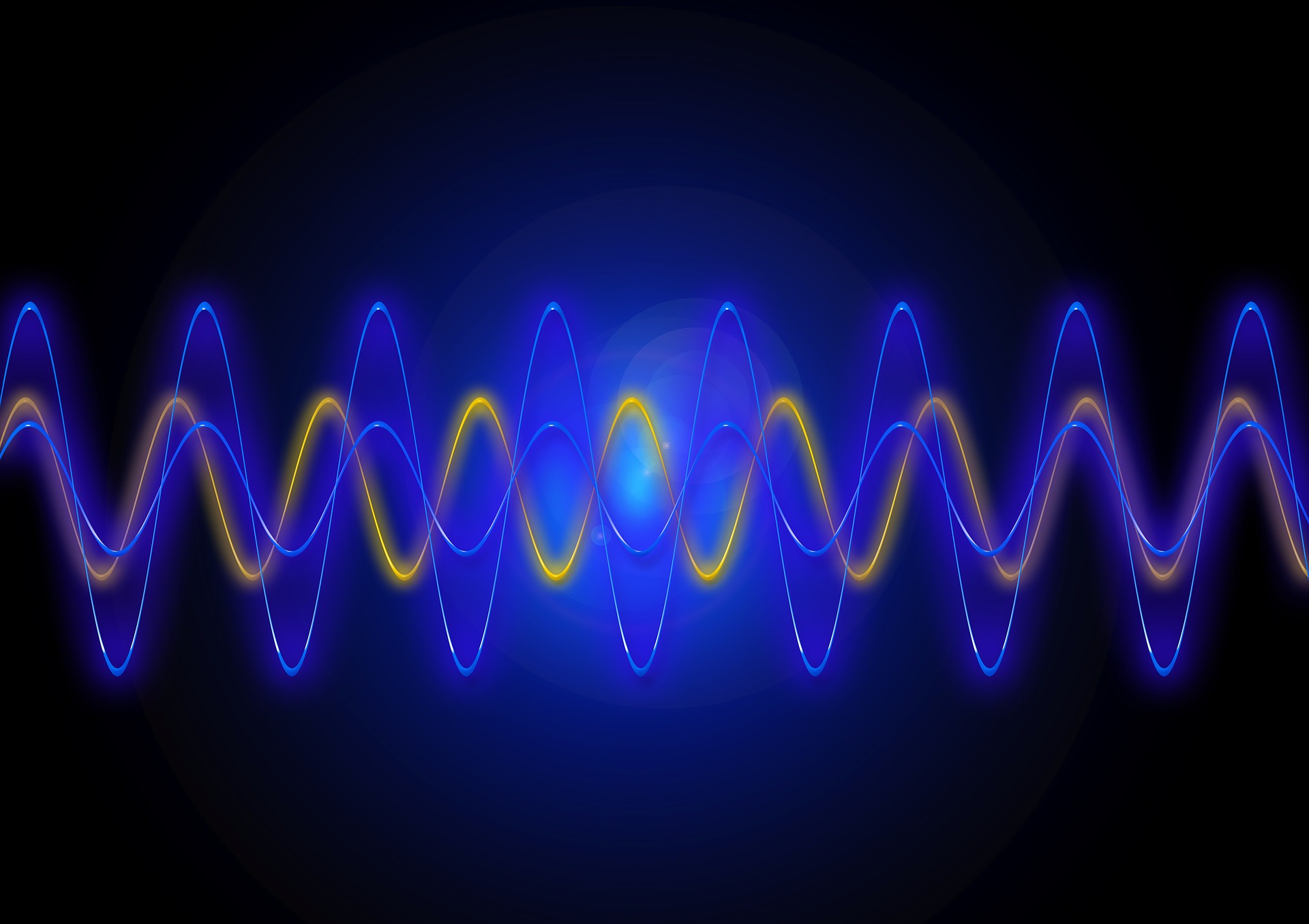
- Read more about High Resolution Attention Network with Acoustic Segment Model for Acoustic Scene Classification
- Log in to post comments
- Categories:
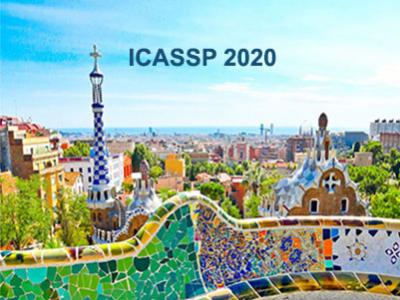
- Read more about Transfer Learning from Youtube Soundtracks to Tag Arctic Ecoacoustic Recordings
- Log in to post comments
- Categories:
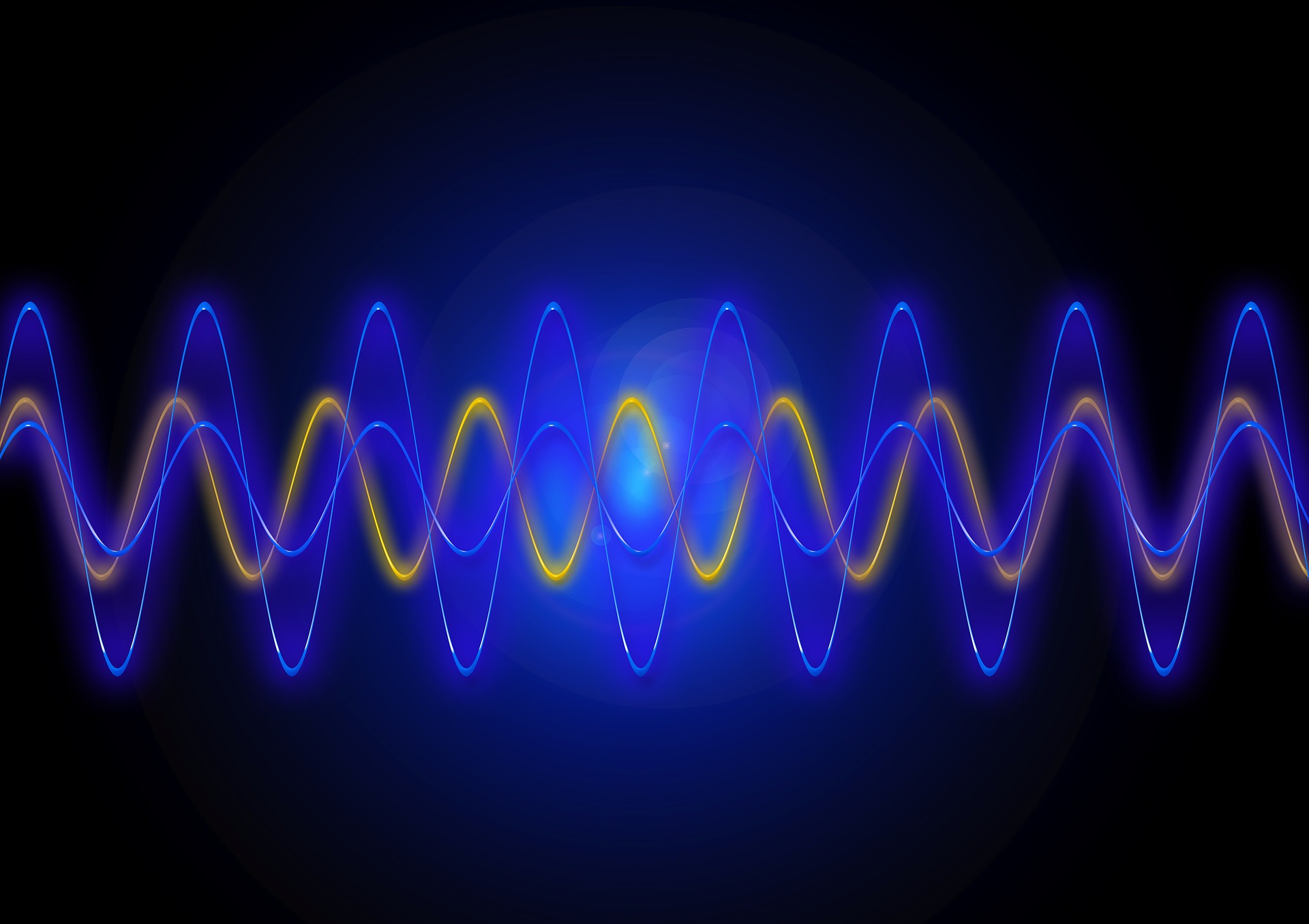
- Read more about SOUND EVENT DETECTION VIA DILATED CONVOLUTIONAL RECURRENT NEURAL NETWORKS
- Log in to post comments
- Categories: