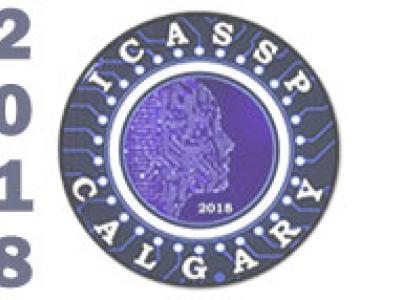
- Read more about NEURAL NETWORK LANGUAGE MODELING WITH LETTER-BASED FEATURES AND IMPORTANCE SAMPLING
- Log in to post comments
In this paper we describe an extension of the Kaldi software toolkit to support neural-based language modeling, intended for use in automatic speech recognition (ASR) and related tasks. We combine the use of subword features (letter ngrams) and one-hot encoding of frequent words so that the models can handle large vocabularies containing infrequent words. We propose a new objective function that allows for training of unnormalized probabilities. An importance sampling based method is supported to speed up training when the vocabulary is large.
- Categories:
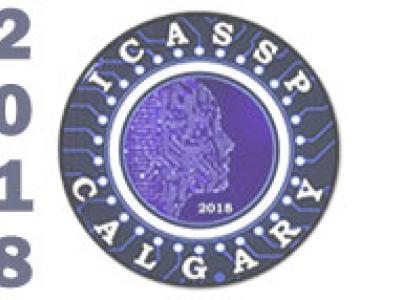
- Read more about ENTROPY BASED PRUNING OF BACKOFF MAXENT LANGUAGE MODELS WITH CONTEXTUAL FEATURES
- Log in to post comments
In this paper, we present a pruning technique for maximum en- tropy (MaxEnt) language models. It is based on computing the exact entropy loss when removing each feature from the model, and it ex- plicitly supports backoff features by replacing each removed feature with its backoff. The algorithm computes the loss on the training data, so it is not restricted to models with n-gram like features, al- lowing models with any feature, including long range skips, triggers, and contextual features such as device location.
poster.pdf

- Categories:
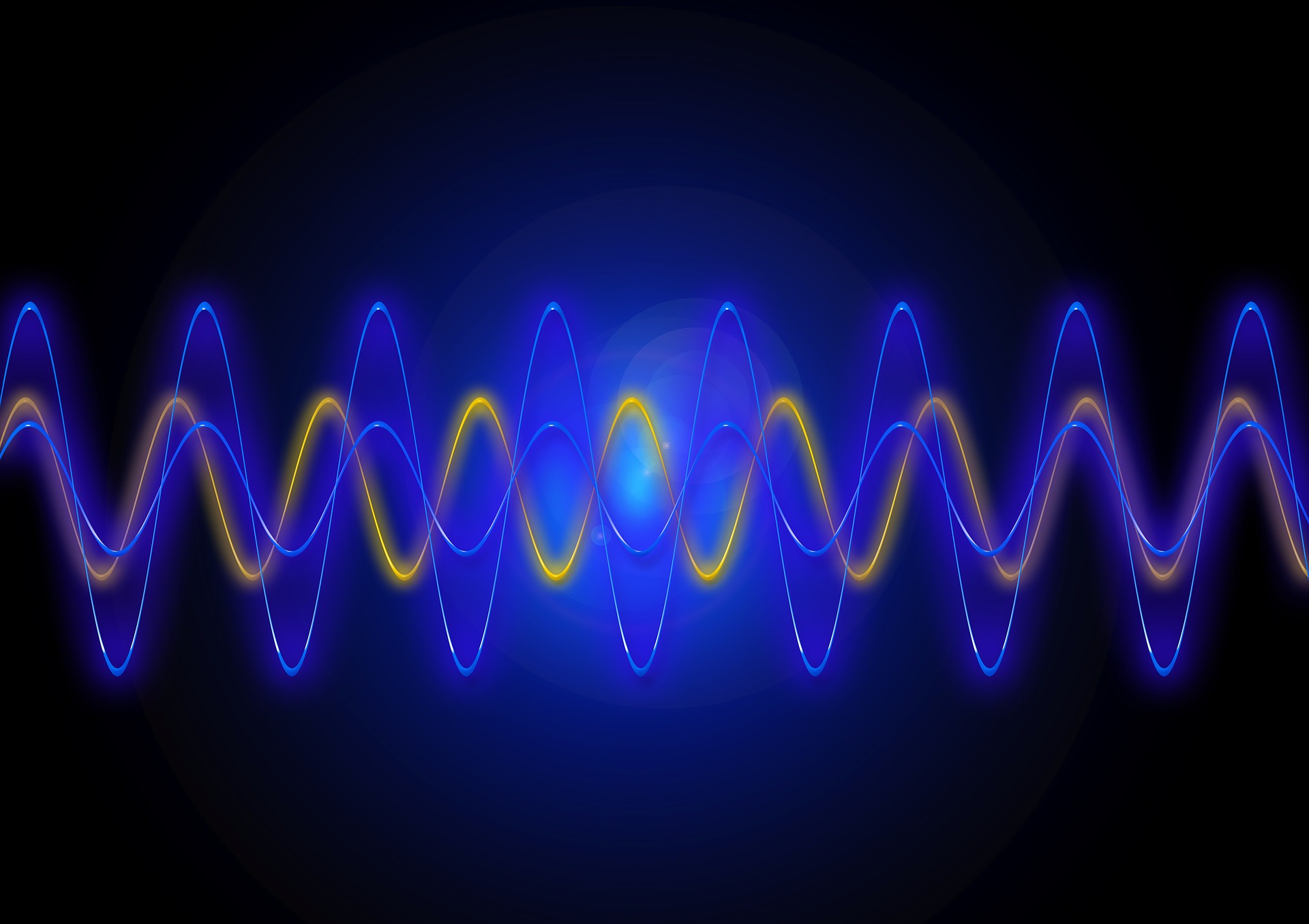
- Read more about LIMITED-MEMORY BFGS OPTIMIZATION OF RECURRENT NEURAL NETWORK LANGUAGE MODELS FOR SPEECH RECOGNITION
- Log in to post comments
- Categories:
- Read more about Dialog Context Language Modeling with Recurrent Neural Networks
- Log in to post comments
We propose contextual language models that incorporate dialog level discourse information into language modeling. Previous works on contextual language model treat preceding utterances as a sequence of inputs, without considering dialog interactions. We design recurrent neural network (RNN) based contextual language models that specially track the interactions between speakers in a dialog. Experiment results on Switchboard Dialog Act Corpus show that the proposed model outperforms conventional single turn based RNN language model by 3.3% on perplexity.
- Categories:
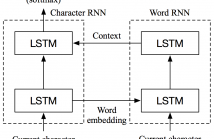
- Read more about CHARACTER-LEVEL LANGUAGE MODELING WITH HIERARCHICAL RECURRENT NEURAL NETWORKS
- Log in to post comments
Recurrent neural network (RNN) based character-level language models (CLMs) are extremely useful for modeling out-of-vocabulary words by nature. However, their performance is generally much worse than the word-level language models (WLMs), since CLMs need to consider longer history of tokens to properly predict the next one. We address this problem by proposing hierarchical RNN architectures, which consist of multiple modules with different timescales.
poster.pdf

- Categories:
- Read more about Directed Automatic Speech Transcription Error Correction Using Bidirectional LSTM
- Log in to post comments
In automatic speech recognition (ASR), error correction after the initial search stage is a commonly used technique to improve performance. Whilst completely automatic error correction, such as full second pass rescoring using complex language models, is widely used, directed error correction, where the error locations are manually given, is of great interest in many scenarios. Previous works on directed error correction usually uses the error location information to change search space with original ASR models.
poster.pdf

- Categories:
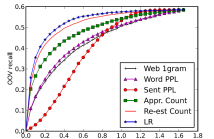
- Read more about Exploiting noisy web data by OOV ranking for low-resource keyword search
- Log in to post comments
Spoken keyword search in low-resource condition suffers from out-of-vocabulary (OOV) problem and insufficient text data for language model (LM) training. Web-crawled text data is used to expand vocabulary and to augment language model. However, the mismatching between web text and the target speech data brings difficulties to effective utilization. New words from web data need an evaluation to exclude noisy words or introduce proper probabilities. In this paper, several criteria to rank new words from web data are investigated and are used as features
- Categories:
- Read more about Learning FOFE based FNN-LMs with noise contrastive estimation and part-of-speech features
- Log in to post comments
A simple but powerful language model called fixed-size
ordinally-forgetting encoding (FOFE) based feedforward neural
network language models (FNN-LMs) has been proposed recently.
Experimental results have shown that FOFE based FNNLMs
can outperform not only the standard FNN-LMs but also
the popular recurrent neural network language models (RNNLMs).
In this paper, we extend FOFE based FNN-LMs from
several aspects. Firstly, we have proposed a new method to
further improve the performance of FOFE based FNN-LMs by
- Categories:
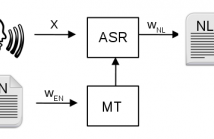
- Read more about Language Model Adaptation for ASR of Spoken Translations Using Phrase-based Translation Models and Named Entity Models
- Log in to post comments
- Categories:
- Read more about CUED-RNNLM – An Open-Source Toolkit for Efficient Training and Evaluation of Recurrent Neural Network Language Models
- Log in to post comments
In recent years, recurrent neural network language models (RNNLMs) have become increasingly popular for a range of applications including speech recognition. However, the training of RNNLMs is computationally expensive, which limits the quantity of data, and size of network, that can be used. In order to fully exploit the power of RNNLMs, efficient training implementations are required. This paper introduces an open-source toolkit, the CUED-RNNLM toolkit, which supports efficient GPU-based training of RNNLMs.
slides.pdf

- Categories: