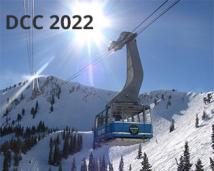
DCC 2022 Conference - The Data Compression Conference (DCC) is an international forum for current work on data compression and related applications. Both theoretical and experimental work are of interest. Visit the DCC 2022 website.
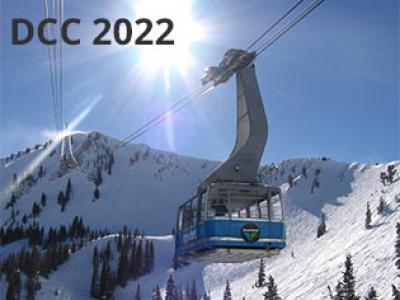
- Read more about Converting RLBWT to LZ77 in smaller space
- 1 comment
- Log in to post comments
RLBWT2LZ77.pdf

- Categories:
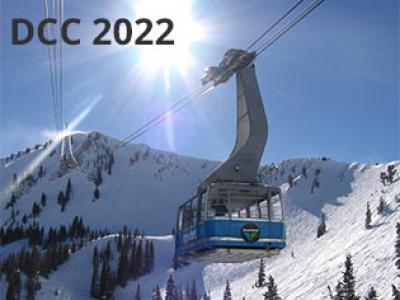
- Read more about Synergies between in-loop and out-of-loop mapping for HDR-PQ content
- Log in to post comments
This paper presents experimental results related to adaptive video content mapping used as a compression tool for HDR-PQ content. The purpose of adaptive video content mapping is to adapt the video signal dynamically depending on its statistical properties in order to better exploit the signal codewords range. Adaptive video content mapping has been investigated during the Versatile Video Coding (VVC) standard development with two main implementation designs: in-loop mapping, and out-of-loop mapping.
- Categories:
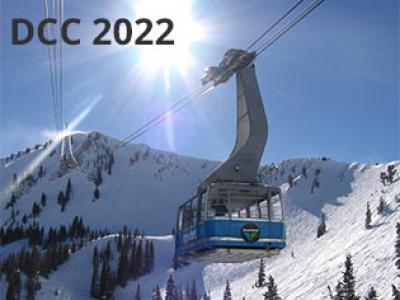
- Read more about Beyond Keypoint Coding: Temporal Evolution Inference with Compact Feature Representation for Talking Face Video Compression
- Log in to post comments
We propose a talking face video compression framework by implicitly transforming the temporal evolution into compact feature representation. More specifically, the temporal evolution of faces, which is complex, non-linear and difficult to extrapolate, is modelled in an end-to-end inference framework based upon very compact features. This enables the high-quality rendering of the face videos, which benefits from the learning of dense motion map with compact feature representation.
- Categories:
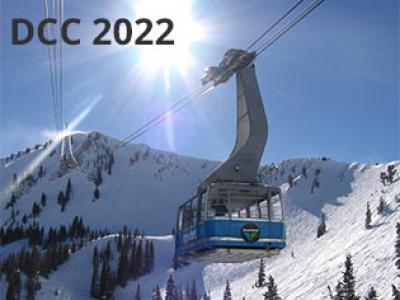
- Read more about Efficient decoder for learned image compression via structured pruning
- Log in to post comments
We propose a structured pruning method to achieve a light-weighted decoder of learned image compression to accommodate various terminals. The structured pruning method identifies the effectiveness of each channel of decoder via gradient ascent and gradient descent while maintaining the encoder and entropy model. To our best knowledge, this paper is the first attempt to design a structured pruning method for universal pretrained learned image compression.
- Categories:
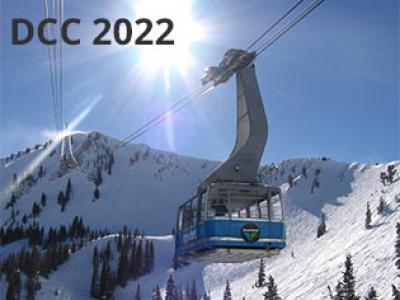
- Read more about Non-Linear Mapping for Image Enhancement
- Log in to post comments
The existing low-light image enhancement methods may cause under enhancement, unbalanced brightness and blurriness. To address these shortcomings, we proposed the non-linear mapping method based on the Retinex theory (NMMR). We use an improved traditional gamma function to estimate the reflectance, and we proposed the maximum brightness channel to estimate the illumination.
2022 DCC.pdf

- Categories:
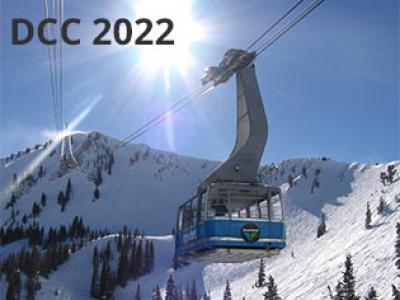
- Read more about Iterative Enhancement Scheme of Synthesized Color and Depth Images for Immersive Video System
- Log in to post comments
- Categories:
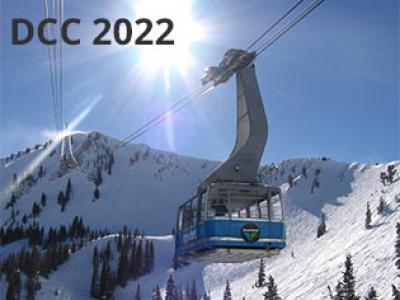
- Read more about Learning Tucker Compression for Deep CNN
- 1 comment
- Log in to post comments
Recently, tensor decomposition approaches are used to compress deep convolutional neural networks (CNN) for getting a faster CNN with fewer parameters. However, there are two problems of tensor decomposition based CNN compression approaches, one is that they usually decompose CNN layer by layer, ignoring the correlation between layers, the other is that training and compressing a CNN is separated, easily leading to local optimum of ranks. In this paper, Learning Tucker Compression (LTC) is proposed.
164-Paper.pdf

- Categories:
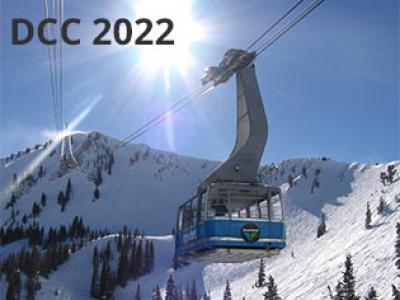
- Read more about Datastructure Lower Bounds for Depth First Search
- Log in to post comments
- Categories:
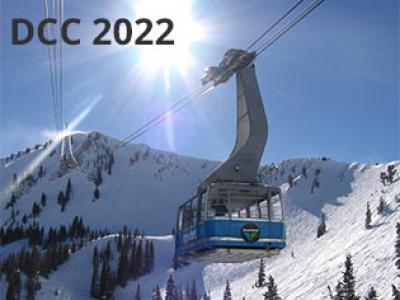
- Read more about A Physics-Informed Vector Quantized Autoencoder for Data Compression of Turbulent Flow
- Log in to post comments
Analyzing large-scale data from simulations of turbulent flows is memory intensive, requiring significant resources. This major challenge highlights the need for data compression techniques. In this study, we apply a physics-informed Deep Learning technique based on vector quantization to generate a discrete, low-dimensional representation of data from simulations of three-dimensional turbulent flows.
- Categories:
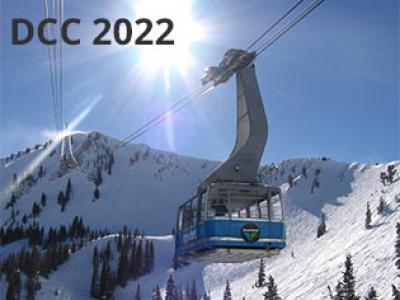
- Read more about Compressing Cipher Images by Using Semi-tensor Product Compressed Sensing and Pre-mapping
- Log in to post comments
As a new signal processing technology, compressed sensing (CS) has been showed to be a promising solution for compressing cipher images. However, the previous CS-based schemes are unsatisfactory in terms of ratio-distortion (R-D) performance. In order to solve this problem, an image encryption-then-compression (ETC) scheme by using semi-tensor product CS (STP-CS) and pre-mapping is proposed in this paper. In the proposed scheme, the original image is encrypted by using the scrambling operation. After image encryption, the cipher image is compressed through three steps.
DCC 2022.pdf

- Categories: