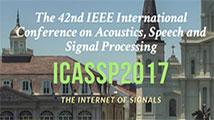
ICASSP is the world's largest and most comprehensive technical conference on signal processing and its applications. It provides a fantastic networking opportunity for like-minded professionals from around the world. ICASSP 2017 conference will feature world-class presentations by internationally renowned speakers and cutting-edge session topics. Visit ICASSP 2017
- Read more about HOUSE FLY (MUSCA DOMESTICA) INSPIRED COMPOUND VISION SENSOR AND BIO-MIMETIC SIGNAL PROCESSING
- Log in to post comments
- Categories:
- Read more about STOCHASTIC TRUNCATED WIRTINGER FLOW ALGORITHM FOR PHASE RETRIEVAL USING BOOLEAN CODED APERTURES
- Log in to post comments
- Categories:
- Read more about Quality Assessment of Mobile Videos with In-Capture Distortions
- Log in to post comments
- Categories:
- Read more about Efficient Methods to Train Multilingual Bottleneck Feature Extractors for Low Resource Keyword Search
- Log in to post comments
- Categories:
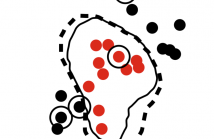
Despite the importance of distributed learning, few fully distributed support vector machines exist. In this paper, not only do we provide a fully distributed nonlinear SVM; we propose the first distributed constrained-form SVM. In the fully distributed context, a dataset is distributed among networked agents that cannot divulge their data, let alone centralize the data, and can only communicate with their neighbors in the network. Our strategy is based on two algorithms: the Douglas-Rachford algorithm and the projection-gradient method.
Poster.pdf

- Categories:
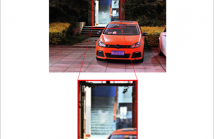
- Read more about Retinex-Based Perceptual Contrast Enhancement in Images Using Luminance Adaptation
- Log in to post comments
In this paper, we propose retinex-based perceptual contrast enhancement in images using luminance adaptation. We use the retinex theory to decompose an image into illumination and reflectance layers, and adopt luminance adaptation to handle the illumination layer which causes detail loss. First, we obtain the illumination layer using adaptive Gaussian filtering to remove halo artifacts. Then, we adaptively remove illumination of the illumination layer in the multi-scale retinex (MSR) process based on luminance adaptation to preserve details.
- Categories:
- Read more about SIMULTANEOUS CODED PLANE WAVE IMAGING IN ULTRASOUND: PROBLEM FORMULATION AND CONSTRAINTS
- Log in to post comments
In this paper, we propose a new emission strategy in the context of plane wave imaging. Plane wave imaging indeed implies compounding in order to preserve a good image quality. Such compounding is usually obtained using multiple, successive emissions, which in turn yields a decrease of the frame rate. As opposed to this approach, our method is based on the simultaneous emission of several coded plane waves. This allows the reconstruction of all the images corresponding to the different plane waves, by using an inverse problem approach.
- Categories:
- Read more about STOCHASTIC TRUNCATED WIRTINGER FLOW ALGORITHM FOR PHASE RETRIEVAL USING BOOLEAN CODED APERTURES
- Log in to post comments
- Categories:
- Read more about STOCHASTIC TRUNCATED WIRTINGER FLOW ALGORITHM FOR PHASE RETRIEVAL USING BOOLEAN CODED APERTURES
- Log in to post comments
- Categories:
- Read more about D2L: DECENTRALIZED DICTIONARY LEARNING OVER DYNAMIC NETWORKS
- Log in to post comments
D4L_slides.pdf

- Categories: