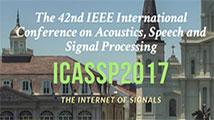
ICASSP is the world's largest and most comprehensive technical conference on signal processing and its applications. It provides a fantastic networking opportunity for like-minded professionals from around the world. ICASSP 2017 conference will feature world-class presentations by internationally renowned speakers and cutting-edge session topics. Visit ICASSP 2017
- Read more about APPLYING COMPENSATION TECHNIQUES ON I-VECTORS EXTRACTED FROM SHORT-TEST UTTERANCES FOR SPEAKER VERIFICATION USING DEEP NEURAL NETWORK
- Log in to post comments
We propose a method to improve speaker verification performance when a test utterance is very short. In some situations with short test utterances, performance of i-vector/probabilistic linear discriminant analysis systems degrades. The proposed method transforms short-utterance feature vectors to adequate vectors using a deep neural network, which compensate for short utterances.
poster.pdf

- Categories:
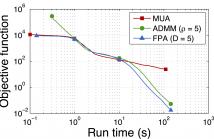
- Read more about PRIMAL-DUAL ALGORITHMS FOR NON-NEGATIVE MATRIX FACTORIZATION WITH THE KULLBACK-LEIBLER DIVERGENCE
- Log in to post comments
Non-negative matrix factorization (NMF) approximates a given matrix as a product of two non-negative matrix factors. Multiplicative algorithms deliver reliable results, but they show slow convergence for high-dimensional data and may be stuck away from local minima. Gradient descent methods have better behavior, but only apply to smooth losses. For non-smooth losses such as the Kullback-Leibler (KL) loss, surprisingly, these methods are lacking.
- Categories:
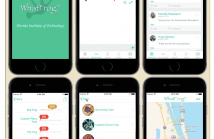
- Read more about Automated Robust Anuran Classification by Extracting Elliptical Feature Pairs from Audio Spectrograms
- Log in to post comments
Ecologists can assess the health of wetlands by monitoring populations of animals such as Anurans (i.e., frogs and toads), which are sensitive to habitat changes. But, surveying anurans requires trained experts to identify species from the animals’ mating calls. This identification task can be streamlined by automation. To this end, we propose an automatic frog-call classification algorithm and a smartphone application that drastically simplify the monitoring of anuran populations.
- Categories:
- Read more about An M-Channel Critically Sampled Graph Filter Bank
- Log in to post comments
We investigate an M-channel critically sampled filter bank for graph signals where each of the M filters is supported on a different subband of the graph Laplacian spectrum. We partition the graph vertices such that the mth set comprises a uniqueness set for signals supported on the mth subband. For analysis, the graph signal is filtered on each subband and downsampled on the corresponding set of vertices.
- Categories:
- Categories:
- Read more about Secure Genomic Susceptibility Testing based on Lattice Encryption
- Log in to post comments
Recent advances in Next Generation Sequencing have increased the availability of genomic data for more accurate analyses, like testing for the genetic susceptibility to a disease. Current laboratories' facilities cannot cope with this data growth, and genomic processing needs to be outsourced, comprising serious privacy risks.
- Categories:
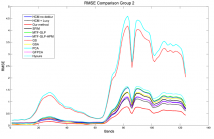
- Read more about Resolution Enhancement for Hyperspectral Images: A Super-Resolution and Fusion Approach
- Log in to post comments
Many remote sensing applications require a high-resolution hyperspectral image. However, resolutions of most hyperspectral imagers are limited to tens of meters. Existing resolution enhancement techniques either acquire additional multispectral band images or use a pan band image. The former poses hardware challenges, whereas the latter has limited performance. In this paper, we present a new resolution enhancement method that only requires a color image.
- Categories:
- Read more about SENTIMENT ANALYSIS WITH RECURRENT NEURAL NETWORK AND UNSUPERVISED NEURAL LANGUAGE MODEL
- Log in to post comments
This paper describes a simple and efficient Neural Language Model approach for text classification that relies only on unsupervised word representation inputs. Our model employs Recurrent Neural Network Long Short-Term Memory (RNN-LSTM), on top of pre-trained word vectors for sentence-level classification tasks. In our hypothesis we argue that using word vectors obtained from an unsupervised neural language model as an extra feature with RNN-LSTM for Natural Language Processing (NLP) system can increase the performance of the system.
- Categories:
- Read more about Building Recurrent Networks by Unfolding Iterative Thresholding for Sequential Sparse Recovery
- Log in to post comments
Historically, sparse methods and neural networks, particularly modern deep learning methods, have been relatively disparate areas. Sparse methods are typically used for signal enhancement, compression,and recovery, usually in an unsupervised framework, while neural networks commonly rely on a supervised training set.
- Categories: