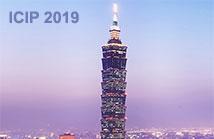
The International Conference on Image Processing (ICIP), sponsored by the IEEE Signal Processing Society, is the premier forum for the presentation of technological advances and research results in the fields of theoretical, experimental, and applied image and video processing. ICIP has been held annually since 1994, brings together leading engineers and scientists in image and video processing from around the world. Visit website.
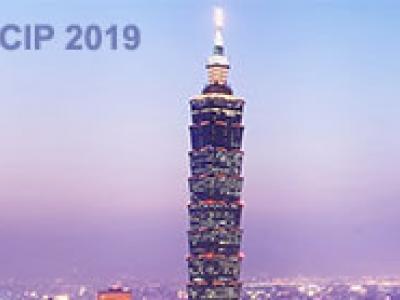
- Read more about From Mapping to Localization: A Complete Framework to Visually Estimate Position and Attitude for Autonomous Vehicles
- 1 comment
- Log in to post comments
Autonomous vehicle framework relies on localization algorithms to position itself and navigates to the destination. In this paper, we explore a light-weight visual localization method to realize the vehicle position and attitude estimation based on images rather than the dominant LIDAR data. We apply SLAM and an offline map correction method to generate a high precision map, which composes 3D points and feature descriptors. For each image, we extract the features and match against the map to explore correspondences.
icip3500.pdf

- Categories:
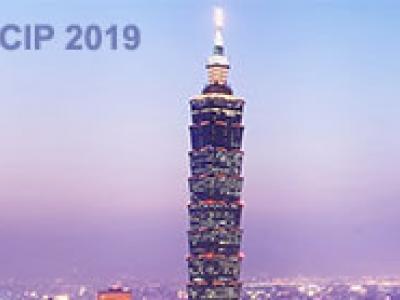
- Read more about AN IMAGE IDENTIFICATION SCHEME OF ENCRYPTED JPEG IMAGES FOR PRIVACY PRESERVING PHOTO SHARING SERVICES
- Log in to post comments
- Categories:
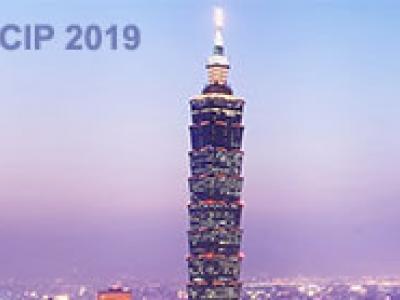
- Read more about Learning The Set Graphs: Image-Set Classification Using Sparse Graph Convolutional Networks
- Log in to post comments
- Categories:
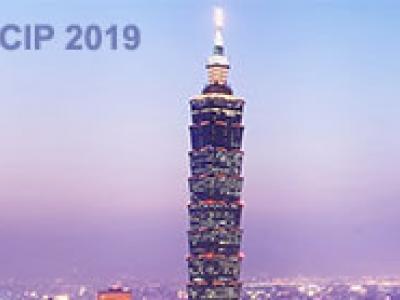
- Read more about VARIABLE-LENGTH QUANTIZATION STRATEGY FOR HASHING
- Log in to post comments
- Categories:
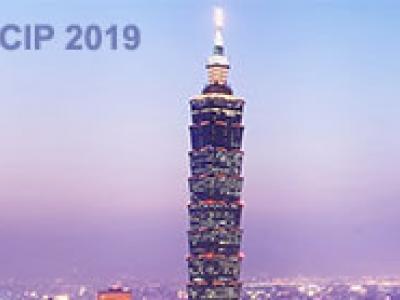
- Read more about When Causal Intervention Meets Adversarial Examples and Image Masking for Deep Neural Networks
- 2 comments
- Log in to post comments
Discovering and exploiting the causality in deep neural networks (DNNs) are crucial challenges for understanding and reasoning causal effects (CE) on an explainable visual model. "Intervention" has been widely used for recognizing a causal relation ontologically. In this paper, we propose a causal inference framework for visual reasoning via do-calculus. To study the intervention effects on pixel-level features for causal reasoning, we introduce pixel-wise masking and adversarial perturbation.
- Categories:
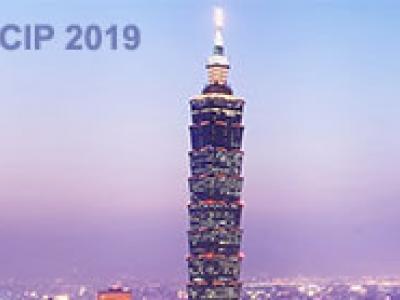
- Read more about RATE-DISTORTION DRIVEN SEPARATION OF DIFFUSE AND SPECULAR COMPONENTS IN MULTIVIEW IMAGERY
- Log in to post comments
In this work we explore an overcomplete representation of
multiview imagery for the purpose of compression. We
present a rate-distortion (R-D) driven approach to decompose
multiview datasets into two additive parts which can
be interpreted as being the diffuse and specular components.
We apply different transforms to each component such that
the compressibility of input data is improved. We describe
a framework which performs the R-D optimized separation
in a registered domain to avoid the complexity of warping
- Categories:
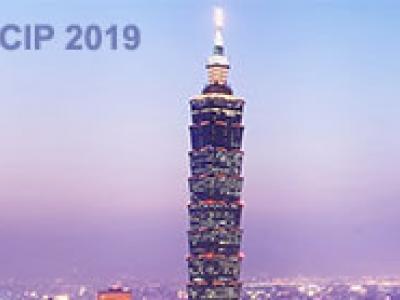
Depth estimation from a single underwater image is one of the most challenging problems and is highly ill-posed. Due to the absence of large generalized underwater depth datasets and the difficulty in obtaining ground truth depth-maps, supervised learning techniques such as direct depth regression cannot be used. In this paper, we propose an unsupervised method for depth estimation from a single underwater image taken "in the wild" by using haze as a cue for depth.
- Categories:
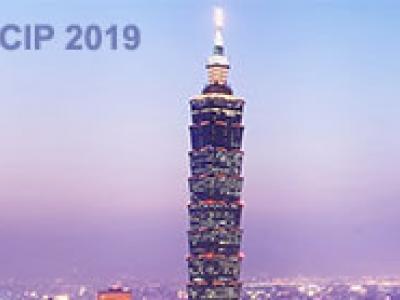
- Read more about REAL-TIME LIGHT FIELD DEPTH ESTIMATION VIA GPU-ACCELERATED MULTI-VIEW SEMI-GLOBAL MATCHING
- Log in to post comments
- Categories: