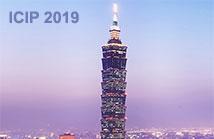
The International Conference on Image Processing (ICIP), sponsored by the IEEE Signal Processing Society, is the premier forum for the presentation of technological advances and research results in the fields of theoretical, experimental, and applied image and video processing. ICIP has been held annually since 1994, brings together leading engineers and scientists in image and video processing from around the world. Visit website.
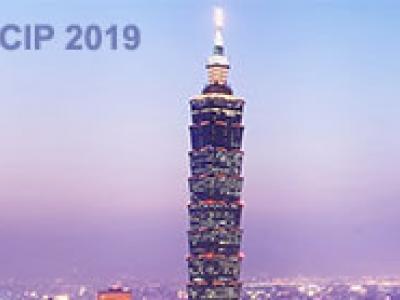
- Read more about Enhanced Video Compression based on Effective Bit depth Adaptation
- Log in to post comments
- Categories:
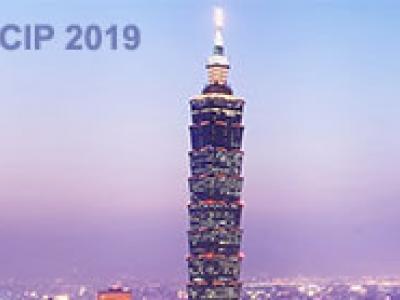
- Read more about IDENTIFICATION OF BUILDINGS IN STREET IMAGES USING MAP INFORMATION
- Log in to post comments
ICIP2019.pdf

- Categories:
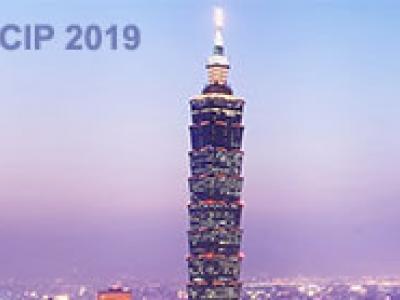
In this paper, we present a novel incremental and decremental learning method for the least-squares support vector machine (LS-SVM). The goal is to adapt a pre-trained model to changes in the training dataset, without retraining the model on all the data, where the changes can include addition and deletion of data samples. We propose a provably exact method where the updated model is exactly the same as a model trained from scratch using the entire (updated) training dataset.
- Categories:
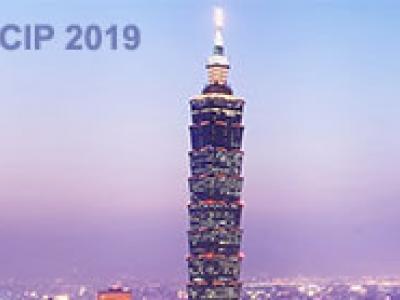
- Read more about DECODING HIGH-THROUGHPUT JPEG2000 (HTJ2K) ON A GPU
- Log in to post comments
High-throughput JPEG2000 (HTJ2K), also known as JPEG 2000 Part 15, is the most recent addition to the JPEG2000 suite of coding tools. The file extension JPH has been designated for compressed images employing this new part of the standard. This new part describes a “fast” block coder for the JPEG 2000 format, while retaining most other JPEG2000 features and capabilities intact.
- Categories:
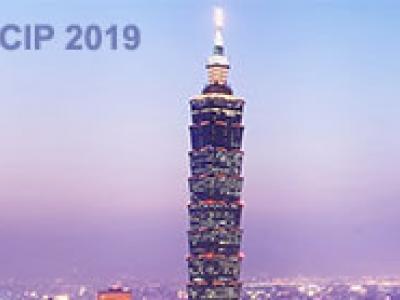
- Read more about JOINT LEARNING OF SELF-REPRESENTATION AND INDICATOR FOR MULTI-VIEW IMAGE CLUSTERING
- Log in to post comments
- Categories:
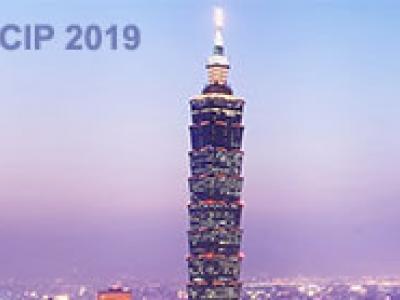
- Read more about RELAXED ORIENTED IMAGE FORESTING TRANSFORM FOR SEEDED IMAGE SEGMENTATION
- Log in to post comments
In this work, we propose a hybrid method for seeded image segmentation, named Relaxed OIFT, which extends a method by Malmberg et al. to directed graphs, to properly incorporate the boundary polarity constraint. Relaxed OIFT lies between the pure Oriented Image Foresting Transform (OIFT) at one end and the extension of Random Walks (RW) to directed graphs as proposed by Singaraju et al. Relaxed OIFT is evaluated in MR and CT medical images, producing more intuitively correct segmentation results than both OIFT and RW, and being easy to be extended to multi-dimensional images.
- Categories:
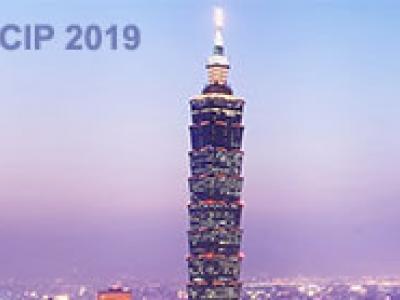
- Read more about Single-image rain removal via multi-scale cascading image generation
- Log in to post comments
A novel single-image rain removal method is proposed based on multi-scale cascading image generation (MSCG). In particular, the proposed method consists of an encoder extracting multi-scale features from images and a decoder generating de-rained images with a cascading mechanism. The encoder ensembles the convolution neural networks using the kernels with different sizes, and integrates their outputs across different scales.
- Categories:
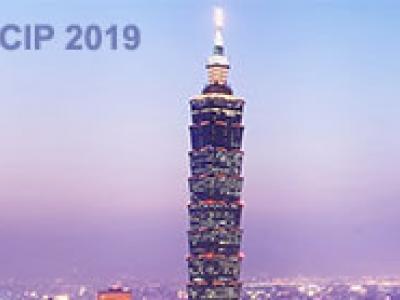
- Read more about A No-Reference Autoencoder Video Quality Metric
- Log in to post comments
In this work, we introduce the No-reference Autoencoder VidEo (NAVE) quality metric, which is based on a deep au-toencoder machine learning technique. The metric uses a set of spatial and temporal features to estimate the overall visual quality, taking advantage of the autoencoder ability to produce a better and more compact set of features. NAVE was tested on two databases: the UnB-AVQ database and the LiveNetflix-II database.
- Categories:

- Read more about Shift R-CNN: Deep Monocular 3D Object Detection with Closed-Form Geometric Constraints
- Log in to post comments
We propose Shift R-CNN, a hybrid model for monocular 3D object detection, which combines deep learning with the power of geometry. We adapt a Faster R-CNN network for regressing initial 2D and 3D object properties and combine it with a least squares solution for the inverse 2D to 3D geometric mapping problem, using the camera projection matrix. The closed-form solution of the mathematical system, along with the initial output of the adapted Faster R-CNN are then passed through a final ShiftNet network that refines the result using our newly proposed Volume Displacement Loss.
- Categories:
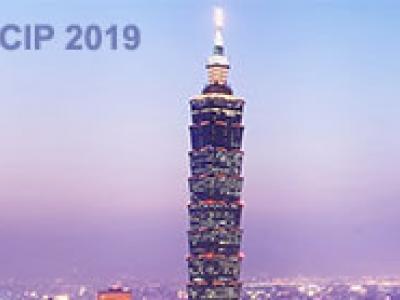
- Read more about 3D Shape Retrieval Through Multilayer RBF Neural Network
- 1 comment
- Log in to post comments
3D object retrieval involves more efforts mainly because major computer vision features are designed for 2D images, which is rarely applicable for 3D models. In this paper, we propose to retrieve the 3D models based on the implicit parameters learned from the radial base functions that represent the 3D objects. The radial base functions are learned from the RBF neural network. As deep neural networks can represent the data that is not linearly separable, we apply multiple layers' neural network to train the radial base functions.
icip3549.pdf

- Categories: