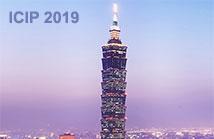
The International Conference on Image Processing (ICIP), sponsored by the IEEE Signal Processing Society, is the premier forum for the presentation of technological advances and research results in the fields of theoretical, experimental, and applied image and video processing. ICIP has been held annually since 1994, brings together leading engineers and scientists in image and video processing from around the world. Visit website.
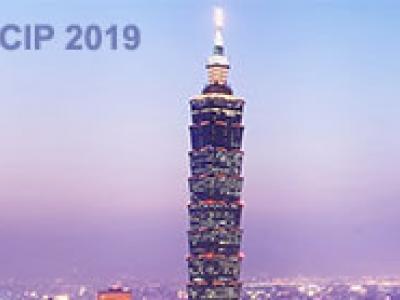
- Read more about Collaborative Method for Incremental Learning on Classification and Generation
- Log in to post comments
- Categories:
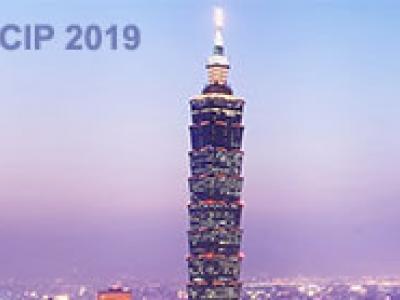
In this study, we propose an efficient approach for modelling and compressing large-scale datasets. The main idea is to subdivide each sample into smaller partitions where each partition constitutes a particular subset of attributes and then apply PCA to each partition separately. This simple approach enjoys several key advantages over the traditional holistic scheme in terms of reduced computational cost and enhanced reconstruction quality.
- Categories:
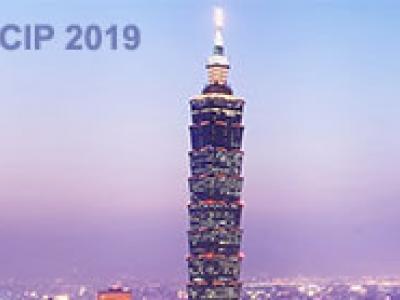
- Read more about Presentation Slides REVE: Regularizing Deep Learning using Variational Entropy Bound
- Log in to post comments
Studies on generalization performance of machine learning algorithms under the scope of information theory suggest that compressed representations can guarantee good generalization, inspiring many compression-based regularization methods. In this paper, we introduce REVE, a new regularization scheme. Noting that compressing the representation can be sub-optimal, our first contribution is to identify a variable that is directly responsible for the final prediction. Our method aims at compressing the class conditioned entropy of this latter variable.
- Categories:
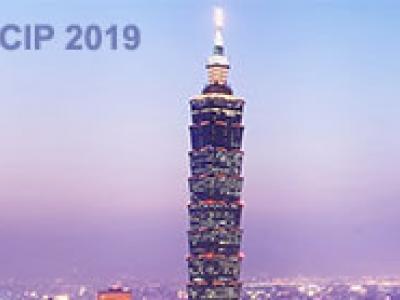
- Read more about Domain Agnostic Video Prediction from Motion Selective Kernels
- Log in to post comments
Existing conditional video prediction approaches train a network from large databases and generalise to previously unseen data. We take the opposite stance, and introduce a model that learns from the first frames of a given video and extends its content and motion, to, \eg double its length. To this end, we propose a dual network that can use in a flexible way both dynamic and static convolutional motion kernels, to predict future frames. We demonstrate experimentally the robustness of our approach on challenging videos in-the-wild and show that it is competitive related baselines.
- Categories:
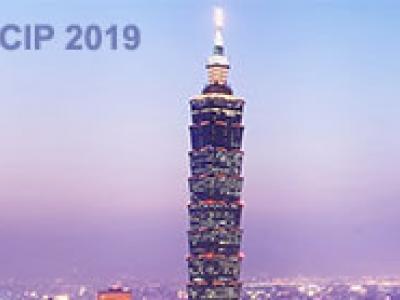
- Read more about Evolutionary Camera Pose Estimation of a Multi-Camera Setup for Computed Tomography
- Log in to post comments
- Categories:
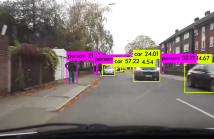
This paper proposed a modified YOLOv3 which has an extra object depth prediction module for obstacle detection and avoidance. We use a pre-processed KITTI dataset to train the proposed, unified model for (i) object detection and (ii) depth prediction and use the AirSim flight simulator to generate synthetic aerial images to verify that our model can be applied in different data domains.
- Categories:
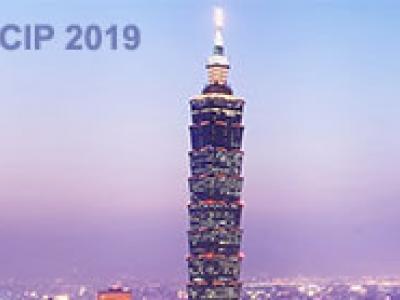
- Read more about Accurate 3D Cell Segmentation Using Deep Features and CRF Refinement
- Log in to post comments
ICIP2019_2.pdf

- Categories:
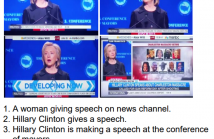
- Categories:
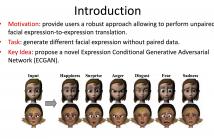
- Read more about Expression Conditional GAN for Facial Expression-To-Expression Translation
- 2 comments
- Log in to post comments
ICIP19_3236.pptx

- Categories:
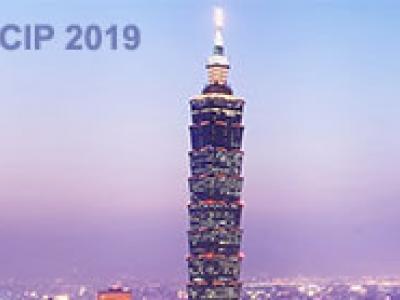
- Read more about High-Accuracy Automatic Person Segmentation with Novel Spatial Saliency Map
- Log in to post comments
In this work, we propose a person segmentation system that achieves high segmentation accuracy with a much smaller CNN network. In this approach, key-point detection annotation is incorporated for the first time and a novel spatial saliency map, in which the intensity of each pixel indicates the likelihood of forming a part of the human and reflects the distance from the body, is generated to provide more spatial information.
- Categories: