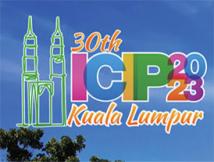
IEEE ICIP 2023 - The International Conference on Image Processing (ICIP), sponsored by the IEEE Signal Processing Society, is the premier forum for the presentation of technological advances and research results in the fields of theoretical, experimental, and applied image and video processing. ICIP has been held annually since 1994, brings together leading engineers and scientists in image and video processing from around the world. Visit website.
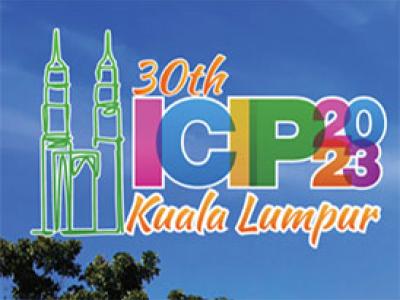
- Read more about DESIGNING STRONG BASELINES FOR TERNARY NEURAL NETWORK QUANTIZATION THROUGH SUPPORT AND MASS EQUALIZATION
- Log in to post comments
- Categories:
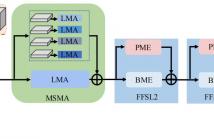
- Read more about GaitMM: Multi-Granularity Motion Sequence Learning for Gait Recognition
- Log in to post comments
Gait recognition aims to identify individual-specific walking patterns by observing the different periodic movements of each body part. However, most existing methods treat each part equally and fail to account for the data redundancy caused by the different step frequencies and sampling rates of gait sequences. In this study, we propose a multi-granularity motion representation network (GaitMM) for gait sequence learning. In GaitMM, we design a combined full-body and fine-grained sequence learning module (FFSL) to explore part-independent spatio-temporal representations.
- Categories:
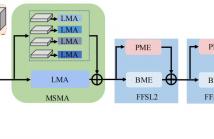
- Read more about GaitMM: Multi-Granularity Motion Sequence Learning for Gait Recognition
- Log in to post comments
Gait recognition aims to identify individual-specific walking patterns by observing the different periodic movements of each body part. However, most existing methods treat each part equally and fail to account for the data redundancy caused by the different step frequencies and sampling rates of gait sequences. In this study, we propose a multi-granularity motion representation network (GaitMM) for gait sequence learning. In GaitMM, we design a combined full-body and fine-grained sequence learning module (FFSL) to explore part-independent spatio-temporal representations.
- Categories:
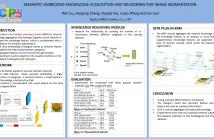
- Read more about SEMANTIC-EMBEDDED KNOWLEDGE ACQUISITION AND REASONING FOR IMAGE SEGMENTATION
- Log in to post comments
Image segmentation is a difficult and challenging task because of the complex object appearance and diverse object categories. Traditional methods directly use visual features for segmentation but ignore the correlation between objects. We introduce a knowledge reasoning module (KRM) for external knowledge aggregation and leverage a graphic neural network to aggregate the knowledge feature, which is concatenated with a visual feature for semantic segmentation. To this end, we use word embedding of category names as semantic feature and establish the relationship between categories.
- Categories:
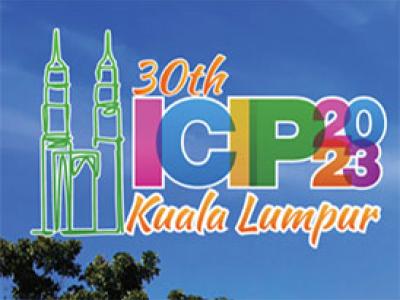
- Read more about ASYMMETRIC SCALABLE CROSS-MODAL HASHING
- 1 comment
- Log in to post comments
pre_icip.pdf

- Categories:
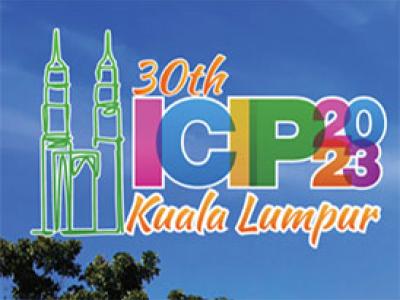
- Read more about A Gradient Boosting Approach for Training Convolutional and Deep Neural Networks
- Log in to post comments
- Categories:
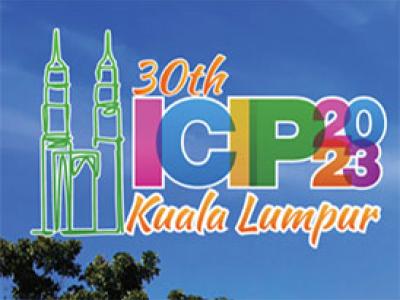
- Read more about HALF OF AN IMAGE IS ENOUGH FOR QUALITY ASSESSMENT
- Log in to post comments
ICIP23.pdf

- Categories: