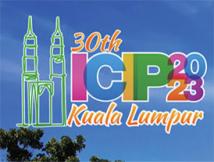
IEEE ICIP 2023 - The International Conference on Image Processing (ICIP), sponsored by the IEEE Signal Processing Society, is the premier forum for the presentation of technological advances and research results in the fields of theoretical, experimental, and applied image and video processing. ICIP has been held annually since 1994, brings together leading engineers and scientists in image and video processing from around the world. Visit website.
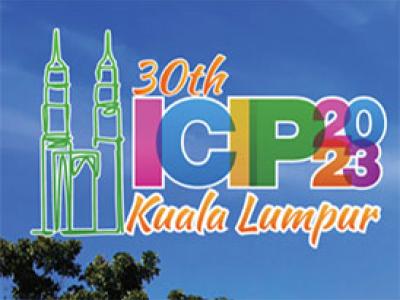
- Read more about ADVERSARIAL EXAMPLE DETECTION BAYESIAN GAME
- Log in to post comments
Despite the increasing attack ability and transferability of adversarial examples (AE), their security, i.e., how unlikely they can be detected, has been ignored more or less. Without the ability to circumvent popular detectors, the chance that an AE successfully fools a deep neural network is slim. This paper gives a game theory analysis of the interplay between an AE attacker and an AE detection investigator. Taking the perspective of a third party, we introduce a game theory model to evaluate the ultimate performance when both the attacker and the investigator are aware of each other.
- Categories:
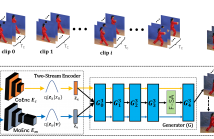
- Read more about ENABLING THE ENCODER-EMPOWERED GAN-BASED VIDEO GENERATORS FOR LONG VIDEO GENERATION
- Log in to post comments
We propose Recall Encoder-empowered GAN3 (REncGAN3), employing the recall mechanism to enable a standard short video (16-frame) generation model EncGAN3 for generating long videos of hundreds of frames.
The recall mechanism utilizes simple changes that enable the generation of connectable short video clips for merging into long sequences, maintaining long-duration consistency.
- Categories:
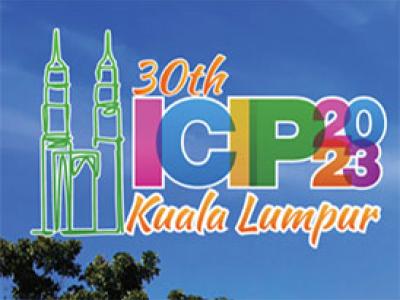
- Read more about Simple Self-Distillation Learning for Noisy Image Classification
- Log in to post comments
- Categories:
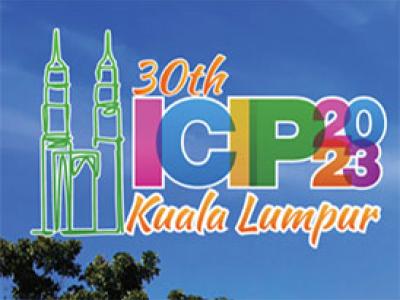
- Read more about Simple Self-Distillation Learning for Noisy Image Classification
- Log in to post comments
- Categories:
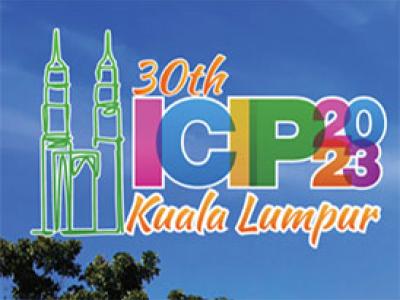
- Read more about CLOT: Contrastive Learning-Driven and Optimal Transport-Based Training for Simultaneous Clustering
- Log in to post comments
Clustering via representation learning is one of the most promising approaches for self-supervised learning of deep neural networks. It aims at obtaining artificial supervisory signals from unlabeled data. In this paper, we propose an online clustering method called CLOT (\underline{C}ontrastive \underline{L}earning-Driven and \underline{O}ptimal \underline{T}ransport-Based Clustering) that is based on robust and multiple losses training settings. More specifically, CLOT learns representations by contrasting both the features at the latent space and the cluster assignments.
- Categories:
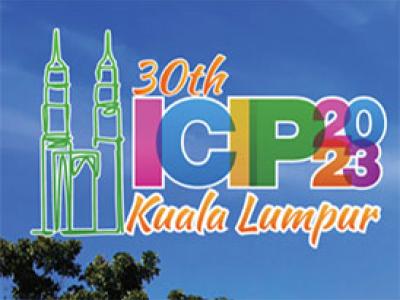
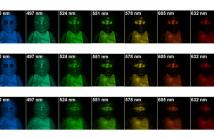
- Read more about Optimized Coded Aperture Design in Compressive Spectral Imaging via Coherence Minimization
- Log in to post comments
The coded aperture snapshot spectral imager (CASSI) system senses spatial and spectral information using a binary coded aperture and a dispersive element, thus the quality of reconstructed hyperspectral images is mainly determined by the structure of coded apertures. Traditional coded apertures (Random, Bernoulli, etc.), encoding hyperspectral images in focal array plane, suffer from suboptimal reconstruction accuracy. Therefore, optimizing coded aperture design improves the reconstruction quality for the scene.
- Categories:
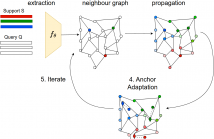
- Read more about Adaptive Anchor Label Propagation for Transductive Few-Shot Learning
- Log in to post comments
Few-shot learning addresses the issue of classifying images using limited labeled data. Exploiting unlabeled data through the use of transductive inference methods such as label propagation has been shown to improve the performance of few-shot learning significantly. Label propagation infers pseudo-labels for unlabeled data by utilizing a constructed graph that exploits the underlying manifold structure of the data.
- Categories:
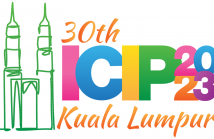
- Read more about QVRF: A QUANTIZATION-ERROR-AWARE VARIABLE RATE FRAMEWORK FOR LEARNED IMAGE COMPRESSION
- Log in to post comments
Learned image compression has exhibited promising compression performance, but variable bitrates over a wide range remain a challenge. State-of-the-art variable rate methods compromise the loss of model performance and require numerous additional parameters. In this paper, we present a Quantization-error-aware Variable Rate Framework (QVRF) that utilizes a univariate quantization regulator a to achieve wide-range variable rates within a single model.
- Categories:
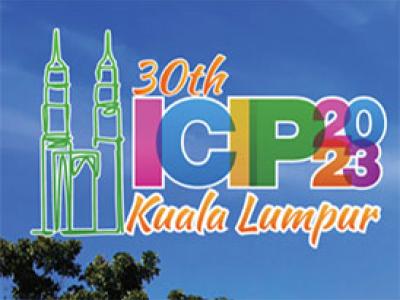
- Read more about DESIGNING STRONG BASELINES FOR TERNARY NEURAL NETWORK QUANTIZATION THROUGH SUPPORT AND MASS EQUALIZATION
- Log in to post comments
- Categories: