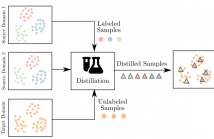
- Read more about Multi-Source Domain Adaptation meets Dataset Distillation through Dataset Dictionary Learning
- Log in to post comments
In this paper, we consider the intersection of two problems in machine learning: Multi-Source Domain Adaptation (MSDA) and Dataset Distillation (DD). On the one hand, the first considers adapting multiple heterogeneous labeled source domains to an unlabeled target domain. On the other hand, the second attacks the problem of synthesizing a small summary containing all the information about the datasets. We thus consider a new problem called MSDA-DD.
- Categories:
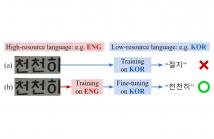
- Read more about CROSS-LINGUAL LEARNING IN MULTILINGUAL SCENE TEXT RECOGNITION
- Log in to post comments
In this paper, we investigate cross-lingual learning (CLL) for multilingual scene text recognition (STR). CLL transfers knowledge from one language to another. We aim to find the condition that exploits knowledge from high-resource languages for improving performance in low-resource languages. To do so, we first examine if two general insights about CLL discussed in previous works are applied to multilingual STR: (1) Joint learning with high- and low-resource languages may reduce performance on low-resource languages, and (2) CLL works best between typologically similar languages.
- Categories:
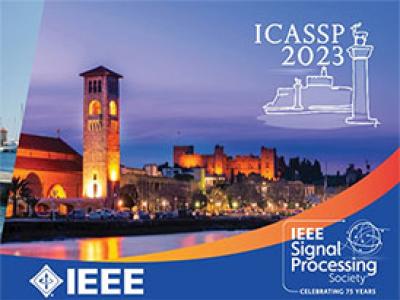
- Read more about Towards Realizing the Value of Labeled Target Samples: a Two-Stage Approach for Semi-Supervised Domain Adaptation
- Log in to post comments
Semi-Supervised Domain Adaptation (SSDA) is a recently emerging research topic that extends from the widely-investigated Unsupervised Domain Adaptation (UDA) by further having a few target samples labeled, i.e., the model is trained with labeled source samples, unlabeled target samples as well as a few labeled} target samples.
- Categories:
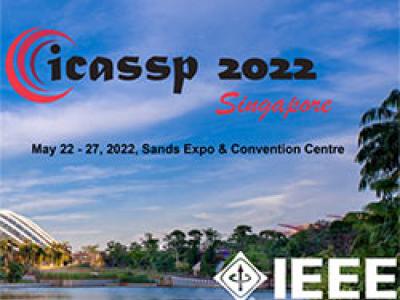
- Read more about SERAB: A MULTI-LINGUAL BENCHMARK FOR SPEECH EMOTION RECOGNITION
- Log in to post comments
The Speech Emotion Recognition Adaptation Benchmark (SERAB) is a new framework to evaluate the performance and generalization capacity of different approaches for utterance-level SER. The benchmark is composed of nine datasets for SER in six languages. We used the proposed framework to evaluate a selection of standard hand-crafted feature sets and state-of-the-art DNN representations. The results highlight that using only a subset of the data included in SERAB can result in biased evaluation, while compliance with the proposed protocol can circumvent this issue.
- Categories:
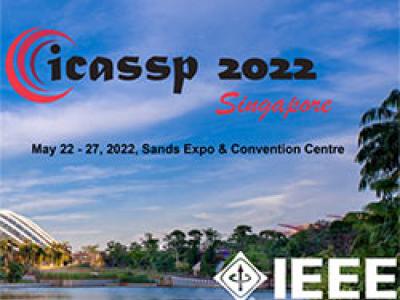
- Read more about Category-Adaptive Domain Adaptation for Semantic Segmentation
- Log in to post comments
poster.pdf

- Categories:
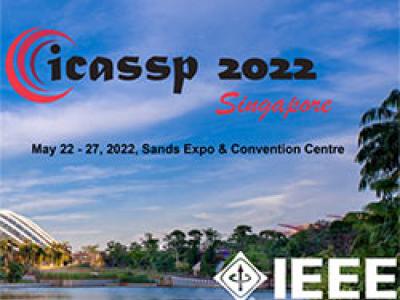
- Read more about EXPLORING TRANSFERABILITY MEASURES AND DOMAIN SELECTION IN CROSS-DOMAIN SLOT FILLING
- Log in to post comments
As an essential task for natural language understanding, slot filling aims to identify the contiguous spans of specific slots in an utterance. In real-world applications, the labeling costs of utterances may be expensive, and transfer learning techniques have been developed to ease this problem. However, cross-domain slot filling could significantly suffer from negative transfer due to non-targeted or zero-shot slots.
- Categories:
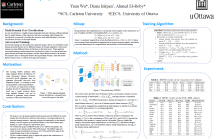
- Read more about Mixup Regularized Adversarial Networks for Multi-Domain Text Classification
- Log in to post comments
Using the shared-private paradigm and adversarial training
can significantly improve the performance of multi-domain
text classification (MDTC) models. However, there are two
issues for the existing methods: First, instances from the multiple
domains are not sufficient for domain-invariant feature
extraction. Second, aligning on the marginal distributions
may lead to a fatal mismatch. In this paper, we propose mixup
regularized adversarial networks (MRANs) to address these
two issues. More specifically, the domain and category mixup
Poster.pdf

- Categories: