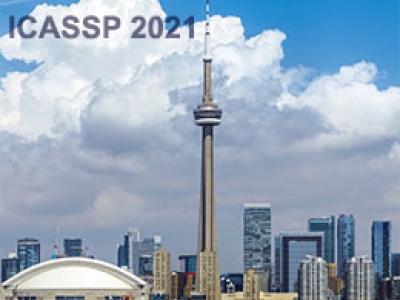
- Categories:
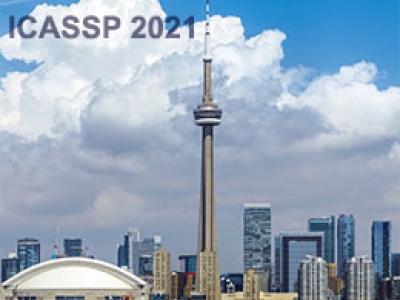
- Read more about Speech Acoustic Modelling from Raw Phase Spectrum
- Log in to post comments
Magnitude spectrum-based features are the most widely employed front-ends for acoustic modelling in automatic speech recognition (ASR) systems. In this paper, we investigate the possibility and efficacy of acoustic modelling using the raw short-time phase spectrum. In particular, we study the usefulness of the raw wrapped, unwrapped and minimum-phase phase spectra as well as the phase of the source and filter components for acoustic modelling.
Poster_RawPhase.pdf

Slides_RawPhase.pdf

- Categories:
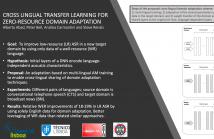
- Read more about CROSS LINGUAL TRANSFER LEARNING FOR ZERO-RESOURCE DOMAIN ADAPTATION
- Log in to post comments
We propose a method for zero-resource domain adaptation of DNN acoustic models, for use in low-resource situations where the only in-language training data available may be poorly matched to the intended target domain. Our method uses a multi-lingual model in which several DNN layers are shared between languages. This architecture enables domain adaptation transforms learned for one well-resourced language to be applied to an entirely different low- resource language.
- Categories:
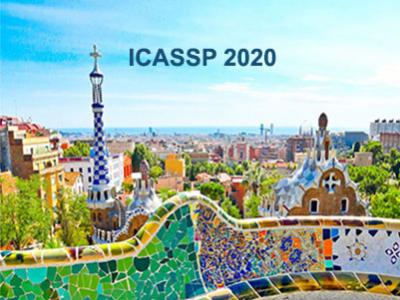
- Read more about DEEP NEURAL NETWORKS BASED AUTOMATIC SPEECH RECOGNITION FOR FOUR ETHIOPIAN LANGUAGES
- Log in to post comments
In this work, we present speech recognition systems for four Ethiopian languages: Amharic, Tigrigna, Oromo and Wolaytta. We have used comparable training corpora of about 20 to 29 hours speech and evaluation speech of about 1 hour for each of the languages. For Amharic and Tigrigna, lexical and language models of different vocabulary size have been developed. For Oromo and Wolaytta, the training lexicons have been used for decoding.
- Categories:
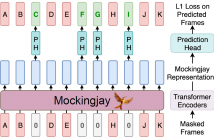
- Read more about Mockingjay: Unsupervised Speech Representation Learning with Deep Bidirectional Transformer Encoders
- Log in to post comments
We present Mockingjay as a new speech representation learning approach, where bidirectional Transformer encoders are pre-trained on a large amount of unlabeled speech. Previous speech representation methods learn through conditioning on past frames and predicting information about future frames. Whereas Mockingjay is designed to predict the current frame through jointly conditioning on both past and future contexts.
- Categories:
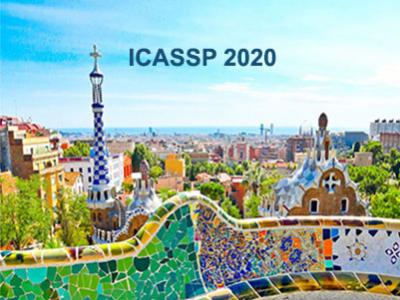
- Read more about TOWARDS FAST AND ACCURATE STREAMING END-TO-END ASR
- Log in to post comments
- Categories:
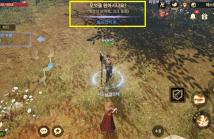
- Read more about Robust End-To-End Keyword Spotting And Voice Command Recognition For Mobile Game
- Log in to post comments
We present an effective method to solve a small-footprint keyword spotting (KWS) and voice command based user interface for mobile game. For KWS task, our goal is to design and implement a computationally very light deep neural network model into mobile device, in the same time to improve the accuracy in various noisy environments. We propose a simple yet effective convolutional neural network (CNN) with Google’s tensorflow-lite for android and Apple’s core ML for iOS deployment.
- Categories:
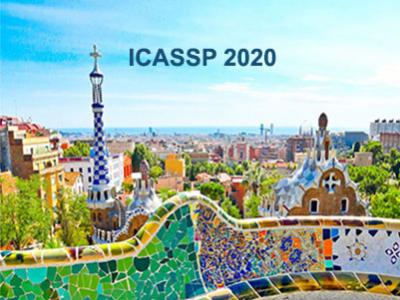
- Read more about A Streaming On-Device End-to-End Model Surpassing Server-Side Conventional Model Quality and Latency
- Log in to post comments
- Categories:
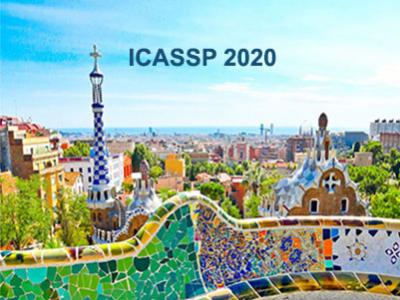
- Read more about An Attention-Based Joint Acoustic and Text On-Device End-to-End Model'
- Log in to post comments
- Categories: