- Image/Video Storage, Retrieval
- Image/Video Processing
- Image/Video Coding
- Image Scanning, Display, and Printing
- Image Formation
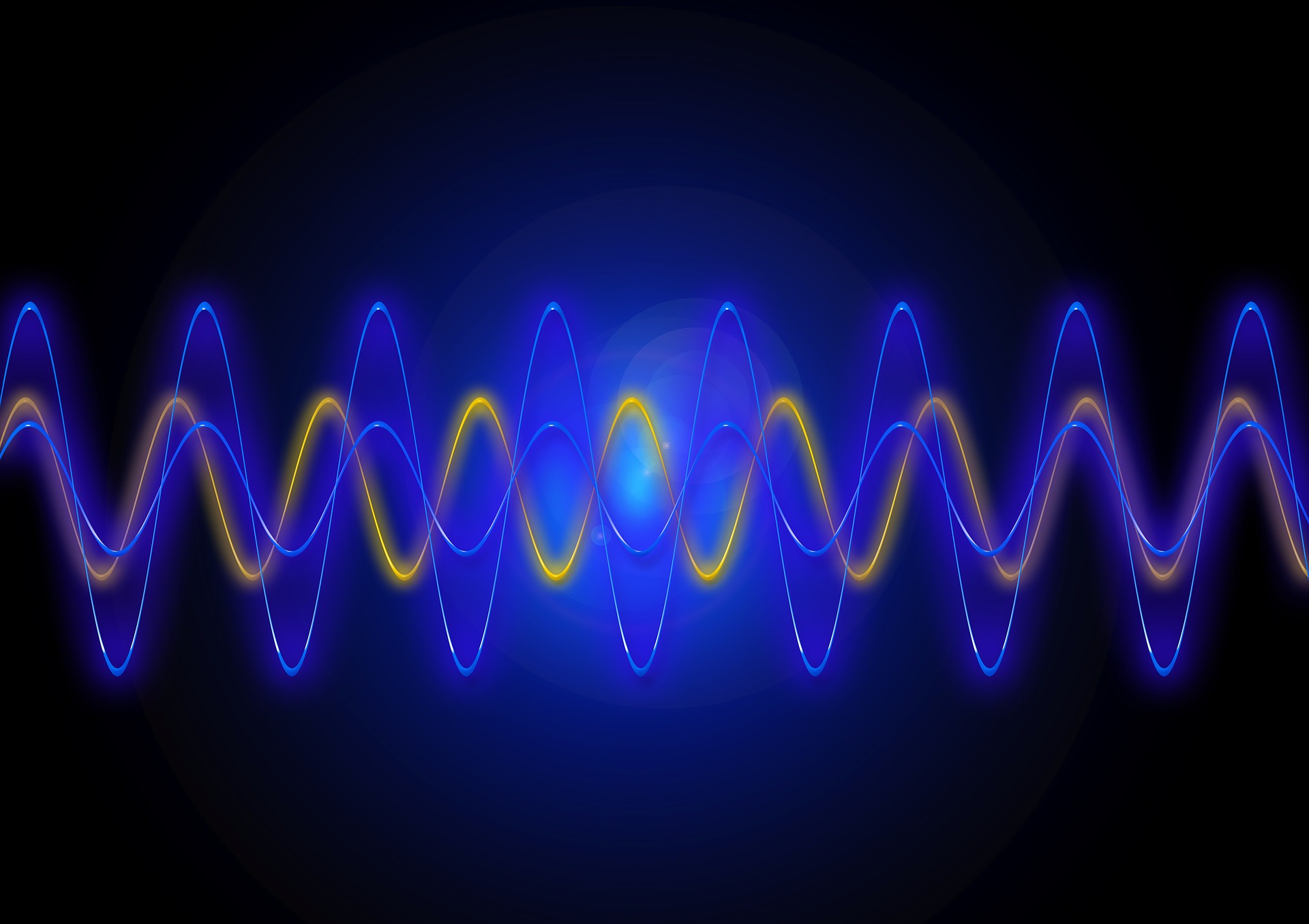
- Read more about Supplement Material for SCIGS: 3D GAUSSIANS SPLATTING FROM A SNAPSHOT COMPRESSIVE IMAGE
- Log in to post comments
In this supplementary material for "SCIGS: 3D GAUSSIANS SPLATTING FROM A SNAPSHOT COMPRESSIVE IMAGE", the results of comparative experiments on all the datasets from dynamic scenes and static scenes are shown. The experiment compares our SCIGS against current state-of-the-art SCI decoding methods and state-of-the-art SCI image-based reconstruction method. An additional experiment is conducted to assess the impact of various mask overlapping rates during the SCI image modulated.
- Categories:
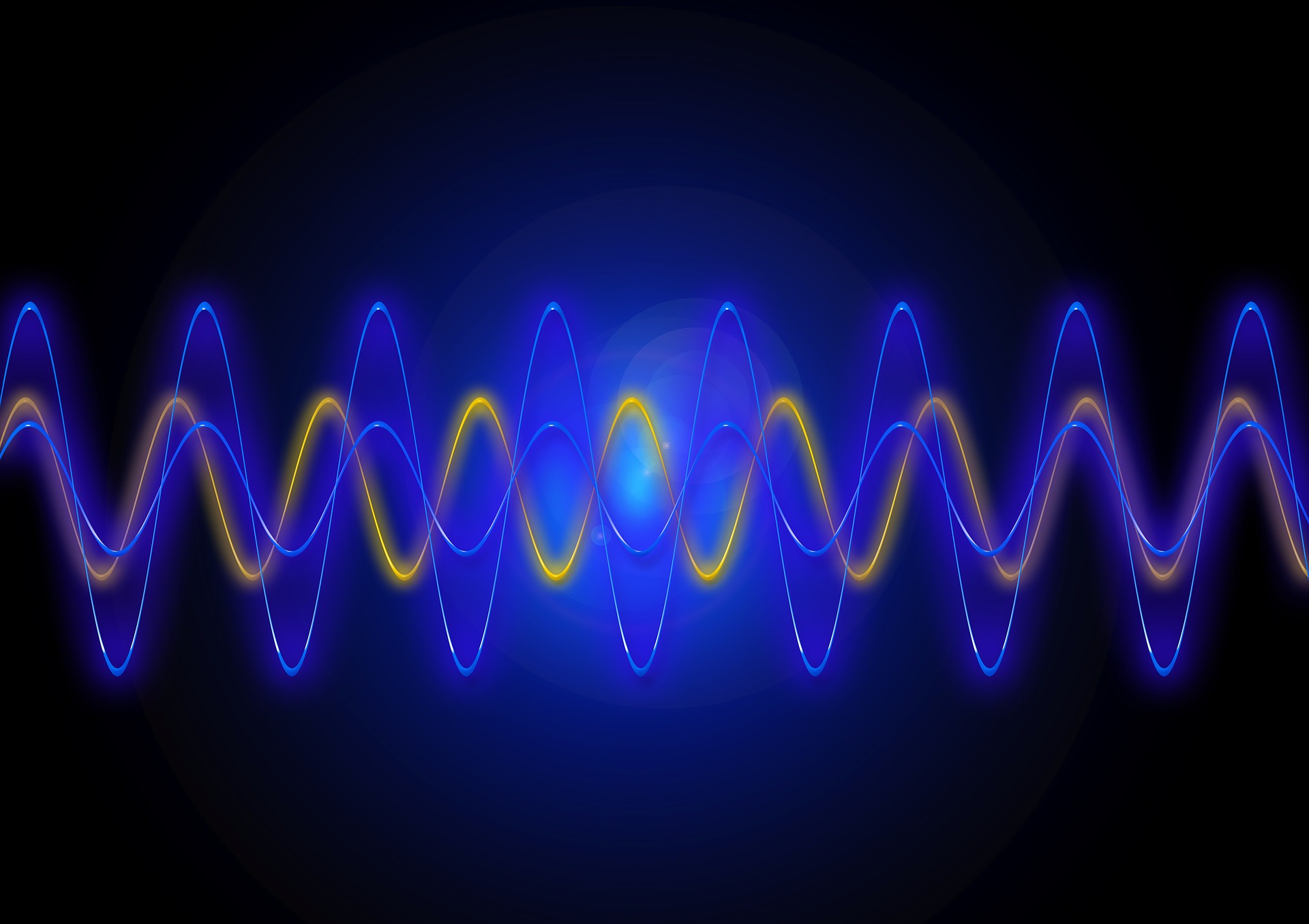
- Read more about Supplementary Materials for "WEAKLY SUPERVISED DEFECT LOCALIZATION WITH RESIDUAL FEATURES"
- Log in to post comments
Supplementary Materials for "WEAKLY SUPERVISED DEFECT LOCALIZATION WITH RESIDUAL FEATURES"
- Categories:
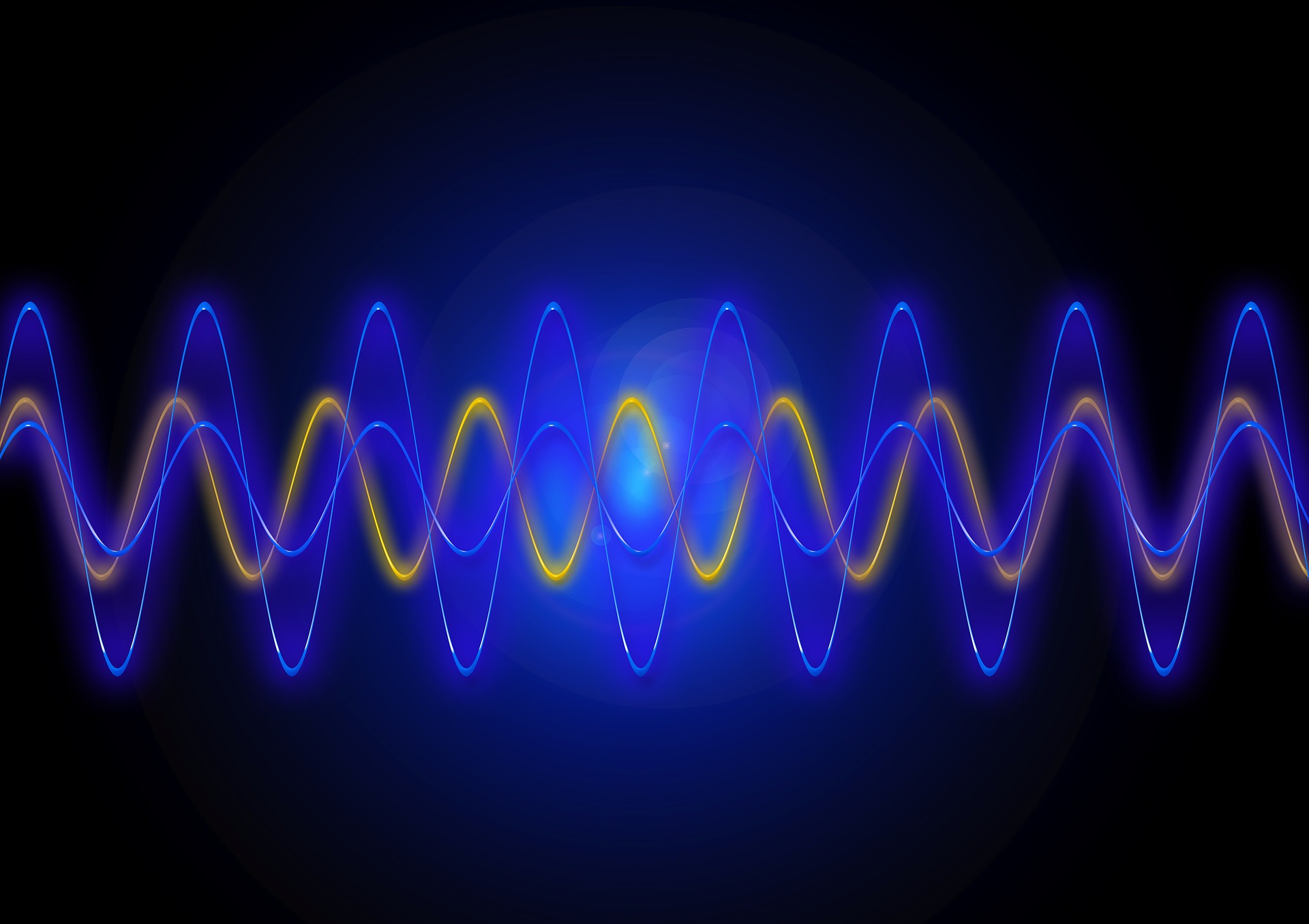
- Read more about Supplementary Material for DARTs: Deformable Animation Ready Templates for Clothing Humans
- Log in to post comments
Accurate 3D modeling of humans and high-fidelity garments is crucial in computer vision and graphics, impacting gaming, virtual, and augmented reality applications.
- Categories:
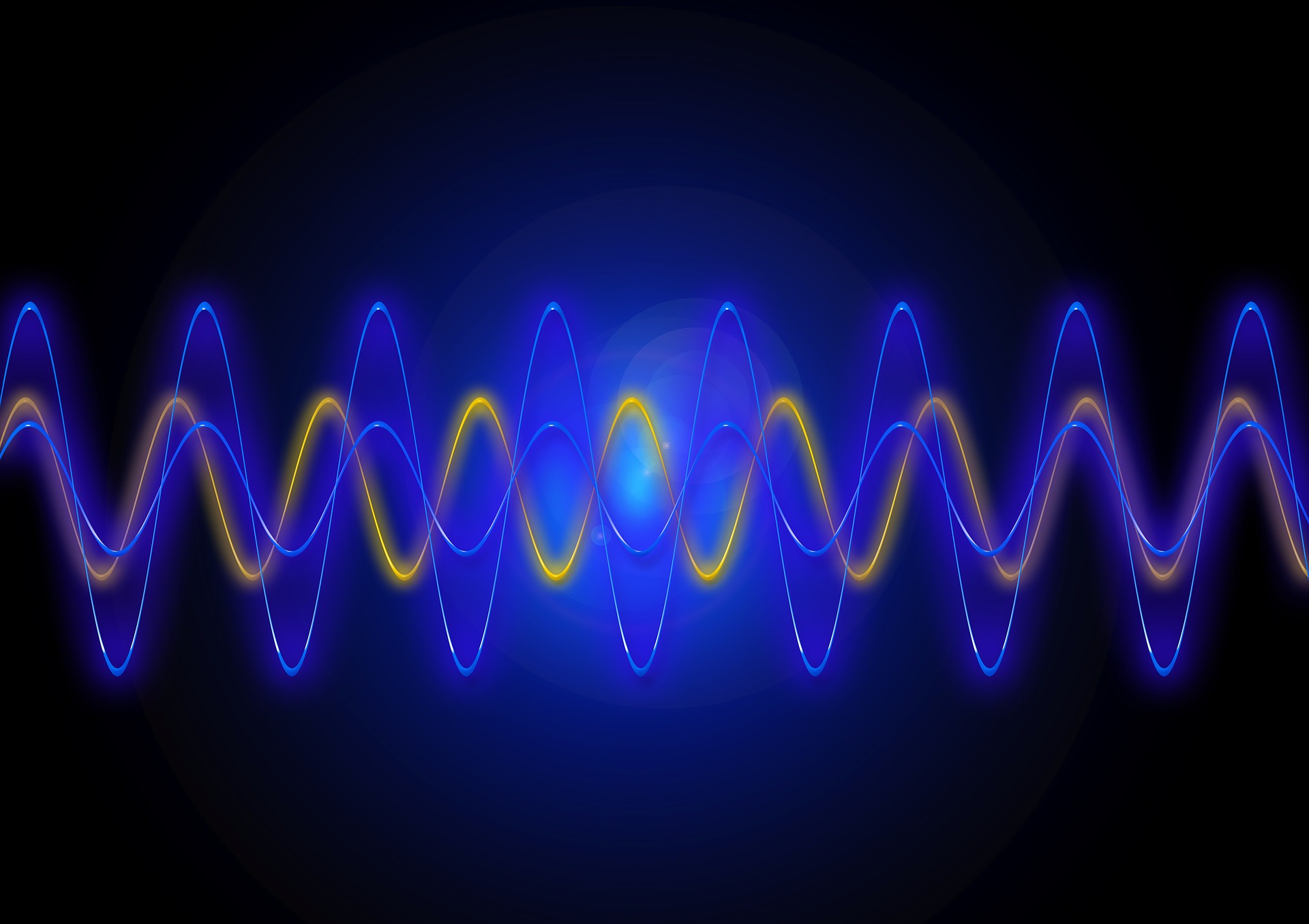
- Read more about HSBS: COMPREHENSIVE BOOSTING OF FACIAL EXPRESSION RECOGNITION VIA HIERARCHICAL SEMANTIC AND BATCH-WISE SIMILARITY
- Log in to post comments
Facial Expression Recognition (FER) has achieved significant success in recent years due to the rise of deep learning. Meanwhile, latent semantic information is crucial for recognizing facial expressions with subtle differences. Inspired by inconsistencies in learning intensity across different layers of deep learning networks — where shallow-layer features lack generalization and task relevance compared to deep-layer features — we propose a novel Hierarchical Semantic Transfer (HST) method.
- Categories:
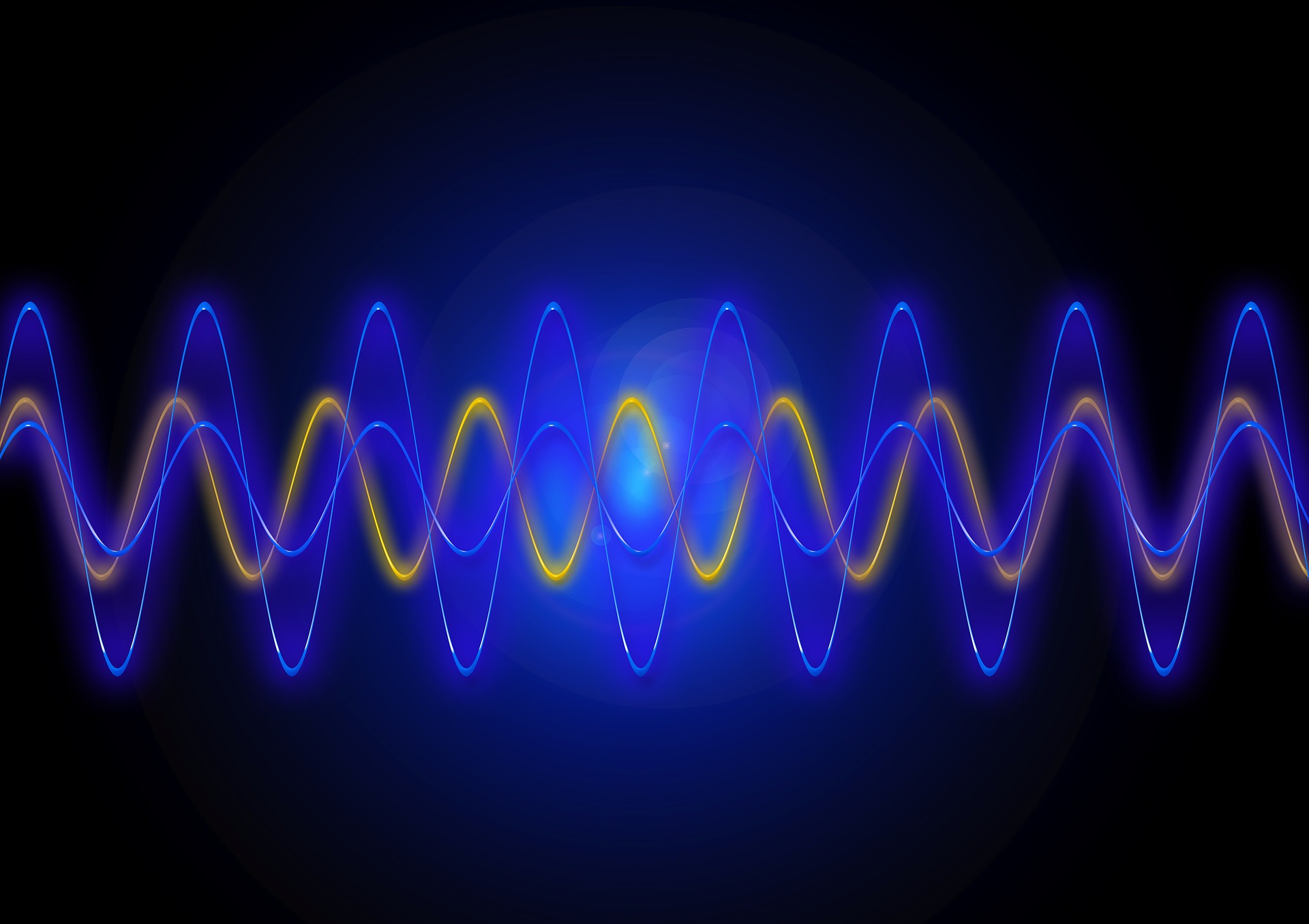
- Read more about HSBS: COMPREHENSIVE BOOSTING OF FACIAL EXPRESSION RECOGNITION VIA HIERARCHICAL SEMANTIC AND BATCH-WISE SIMILARITY
- Log in to post comments
Facial Expression Recognition (FER) has achieved significant success in recent years due to the rise of deep learning. Meanwhile, latent semantic information is crucial for recognizing facial expressions with subtle differences. Inspired by inconsistencies in learning intensity across different layers of deep learning networks — where shallow-layer features lack generalization and task relevance compared to deep-layer features — we propose a novel Hierarchical Semantic Transfer (HST) method.
- Categories:
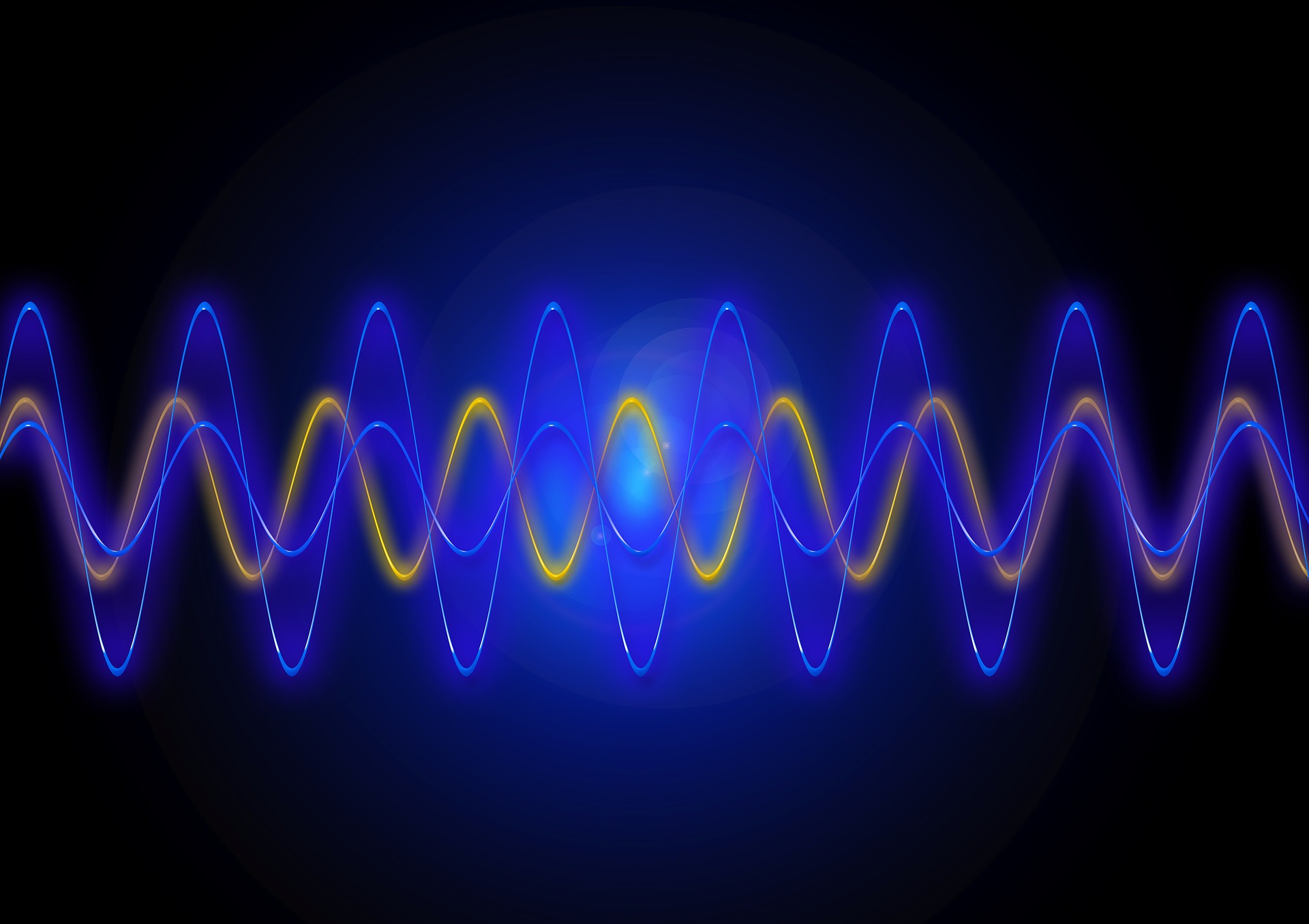
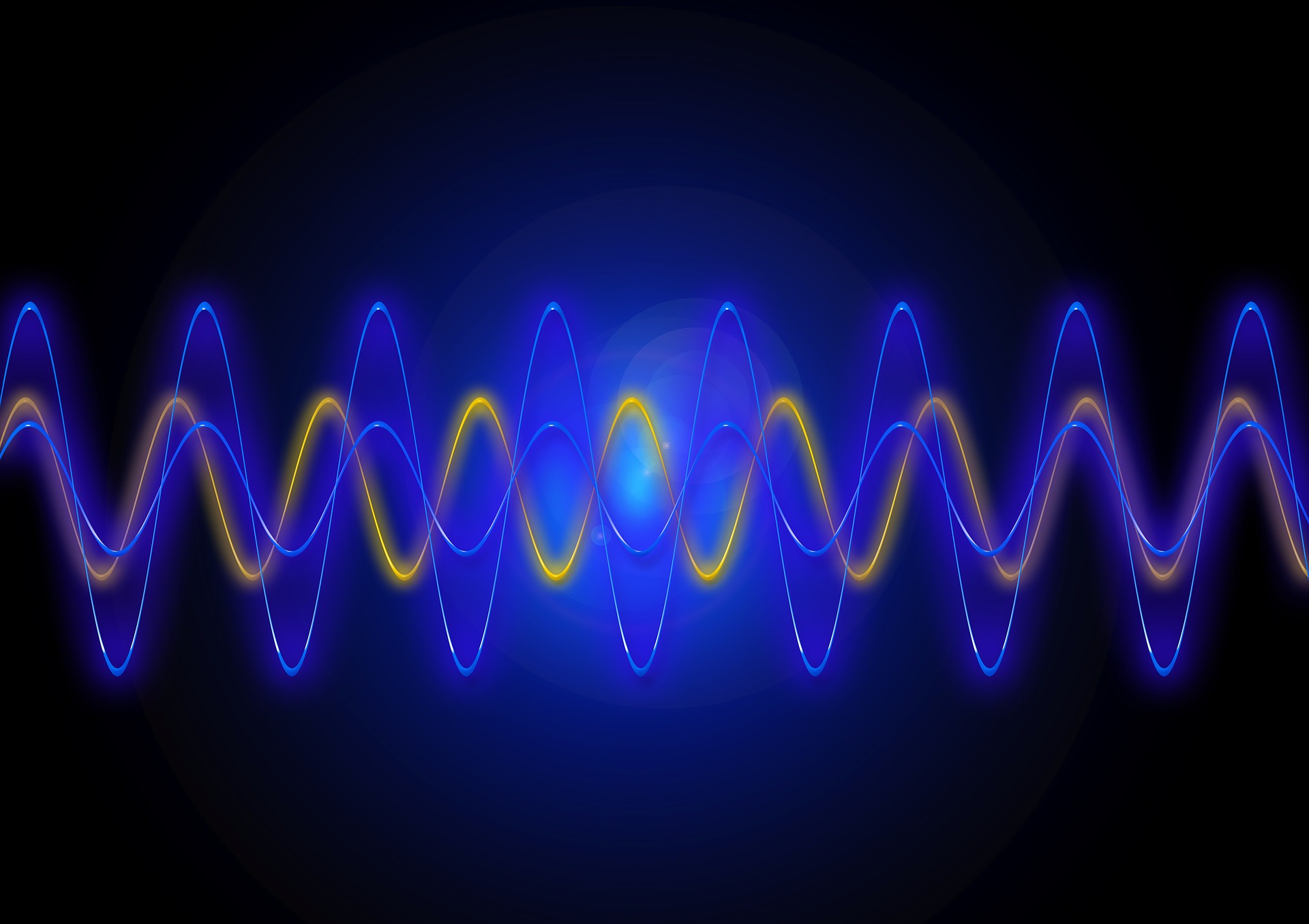
- Read more about Retrieval-Augmented Natural Language Reasoning for Explainable Visual Question Answering
- Log in to post comments
Visual Question Answering with Natural Language Explanation (VQA-NLE) task is challenging due to its high demand for reasoning-based inference. Recent VQA-NLE studies focus on enhancing model networks to amplify the model’s reasoning capability but this approach is resource consuming and unstable. In this work, we introduce a new VQA-NLE model, ReRe (Retrieval-augmented natural language Reasoning), using leverage retrieval information from the memory to aid in generating accurate answers and persuasive explanations without relying on complex networks and extra datasets.
- Categories:
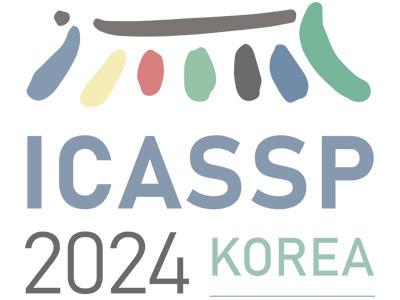
- Read more about FEATURE-CONSTRAINED AND ATTENTION-CONDITIONED DISTILLATION LEARNING FOR VISUAL ANOMALY DETECTION
- Log in to post comments
Visual anomaly detection in computer vision is an essential one-class classification and segmentation problem. The student-teacher (S-T) approach has proven effective in addressing this challenge. However, previous studies based on S-T underutilize the feature representations learned by the teacher network, which restricts anomaly detection performance.
- Categories:
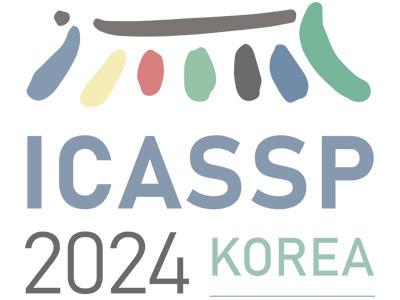
- Read more about MULTI-MODALITY ACTION RECOGNITION BASED ON DUAL FEATURE SHIFT IN VEHICLE CABIN MONITORING
- Log in to post comments
Driver Action Recognition (DAR) is crucial in vehicle cabin monitoring systems. In real-world applications, it is common for vehicle cabins to be equipped with cameras featuring different modalities. However, multi-modality fusion strategies for the DAR task within car cabins have rarely been studied. In this paper, we propose a novel yet efficient multi-modality driver action recognition method based on dual feature shift, named DFS. DFS first integrates complementary features across modalities by performing modality feature interaction.
- Categories:
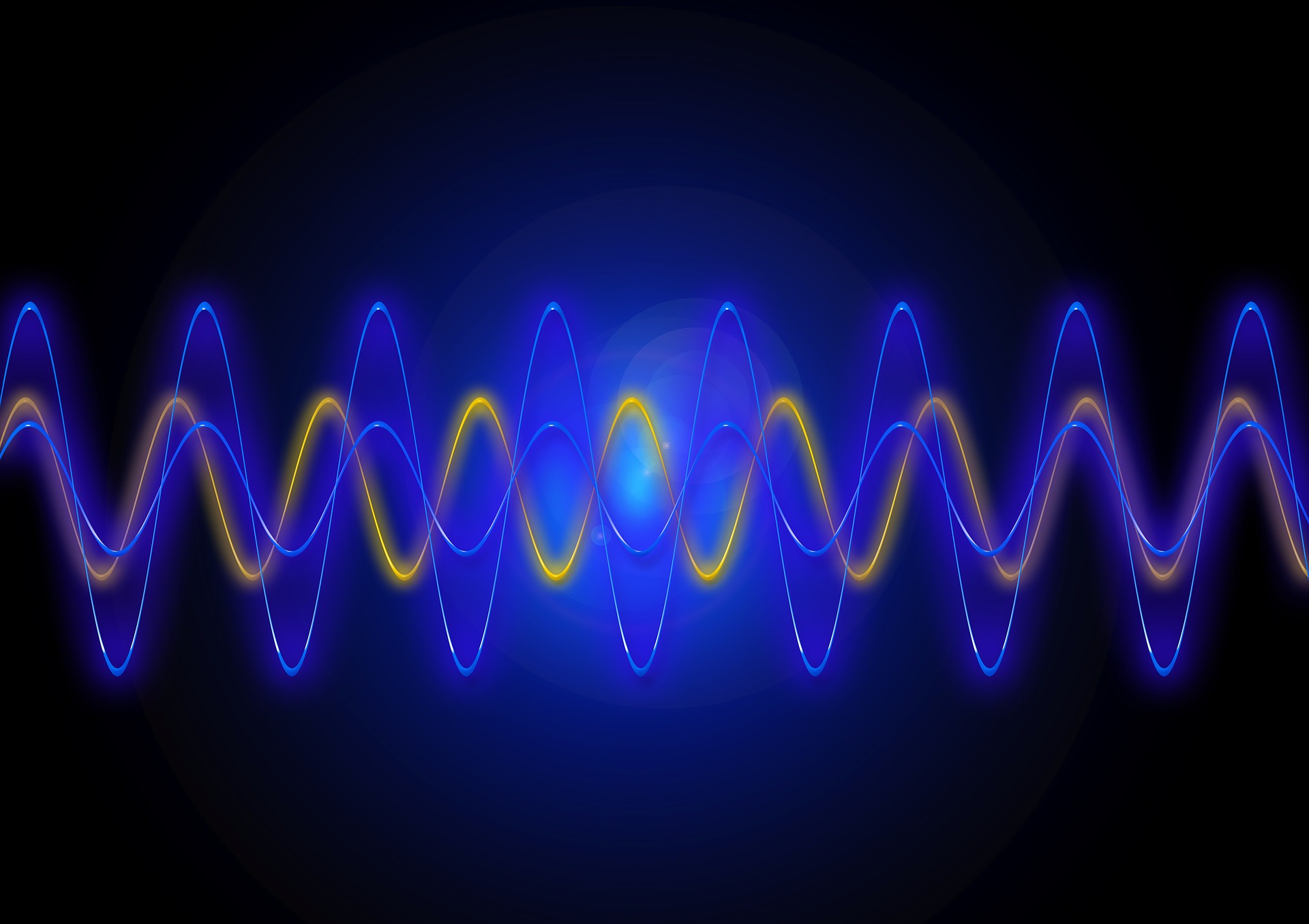
- Read more about MULTILINGUAL AUDIO-VISUAL SPEECH RECOGNITION WITH HYBRID CTC/RNN-T FAST CONFORMER
- Log in to post comments
Humans are adept at leveraging visual cues from lip movements for recognizing speech in adverse listening conditions. Audio-Visual Speech Recognition (AVSR) models follow similar approach to achieve robust speech recognition in noisy conditions. In this work, we present a multilingual AVSR model incorporating several enhancements to improve performance and audio noise robustness. Notably, we adapt the recently proposed Fast Conformer model to process both audio and visual modalities using a novel hybrid CTC/RNN-T architecture.
- Categories: