- Image/Video Storage, Retrieval
- Image/Video Processing
- Image/Video Coding
- Image Scanning, Display, and Printing
- Image Formation
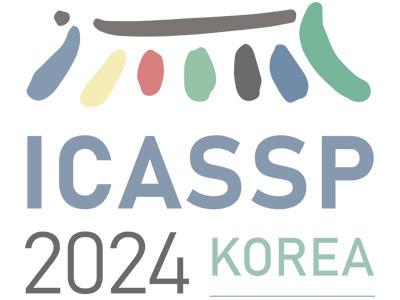
- Read more about LEARNING SPATIO-TEMPORAL RELATIONS WITH MULTI-SCALE INTEGRATED PERCEPTION FOR VIDEO ANOMALY DETECTION
- Log in to post comments
In weakly supervised video anomaly detection, it has been verified that anomalies can be biased by background noise. Previous works attempted to focus on local regions to exclude irrelevant information. However, the abnormal events in different scenes vary in size, and current methods struggle to consider local events of different scales concurrently. To this end, we propose a multi-scale integrated perception
ye_poster.pdf

- Categories:
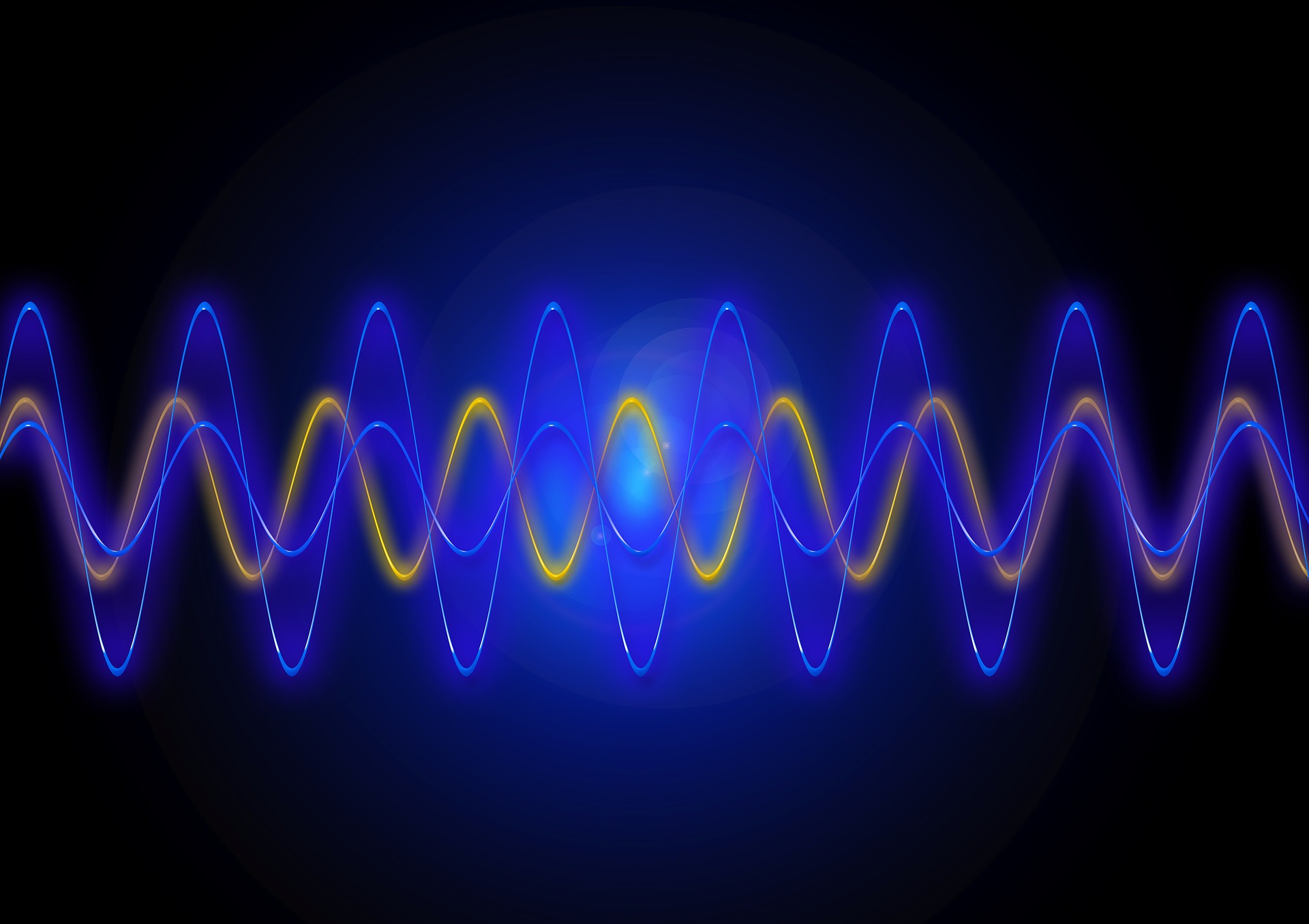
- Read more about DCL-NET DUAL CONTRASTIVE LEARNING NETWORK FOR SEMI-SUPERVISED MULTI-ORGAN SEGMENTATION
- Log in to post comments
Semi-supervised learning (SSL) is a sound measure to relieve the strict demand of abundant annotated datasets, especially for challenging multi-organ segmentation (MoS). However, most existing SSL methods predict pixels in a single image independently, ignoring the relations among images and categories. In this paper, we propose a two-stage Dual Contrastive Learning Network (DCL-Net) for semi-supervised MoS, which utilizes global and local contrastive learning to strengthen the relations among images and classes.
- Categories:
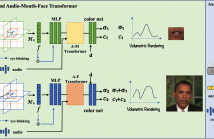
- Read more about DT-NeRF: Decomposed Triplane-Hash Neural Radiance Fields for High-Fidelity Talking Portrait Synthesis
- Log in to post comments
In this paper, we present the decomposed triplane-hash neural radiance fields (DT-NeRF), a framework that significantly improves the photorealistic rendering of talking faces and achieves state-of-the-art results on key evaluation datasets. Our architecture decomposes the facial region into two specialized triplanes: one specialized for representing the mouth, and the other for the broader facial features. We introduce audio features as residual terms and integrate them as query vectors into our model through an audio-mouthface transformer.
- Categories:
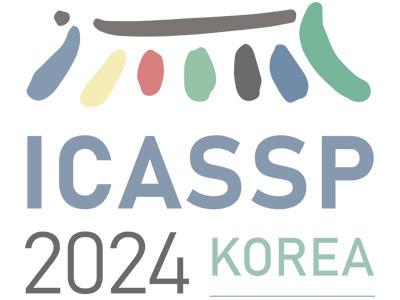
- Read more about Enhancing Adversarial Transferability in Object Detection with Bidirectional Feature Distortion
- Log in to post comments
Previous works have shown that perturbing internal-layer features can significantly enhance the transferability of black-box attacks in classifiers. However, these methods have not achieved satisfactory performance when applied to detectors due to the inherent differences in features between detectors and classifiers. In this paper, we introduce a concise and practical untargeted adversarial attack in a label-free manner, which leverages only the feature extracted from the backbone model.
- Categories:
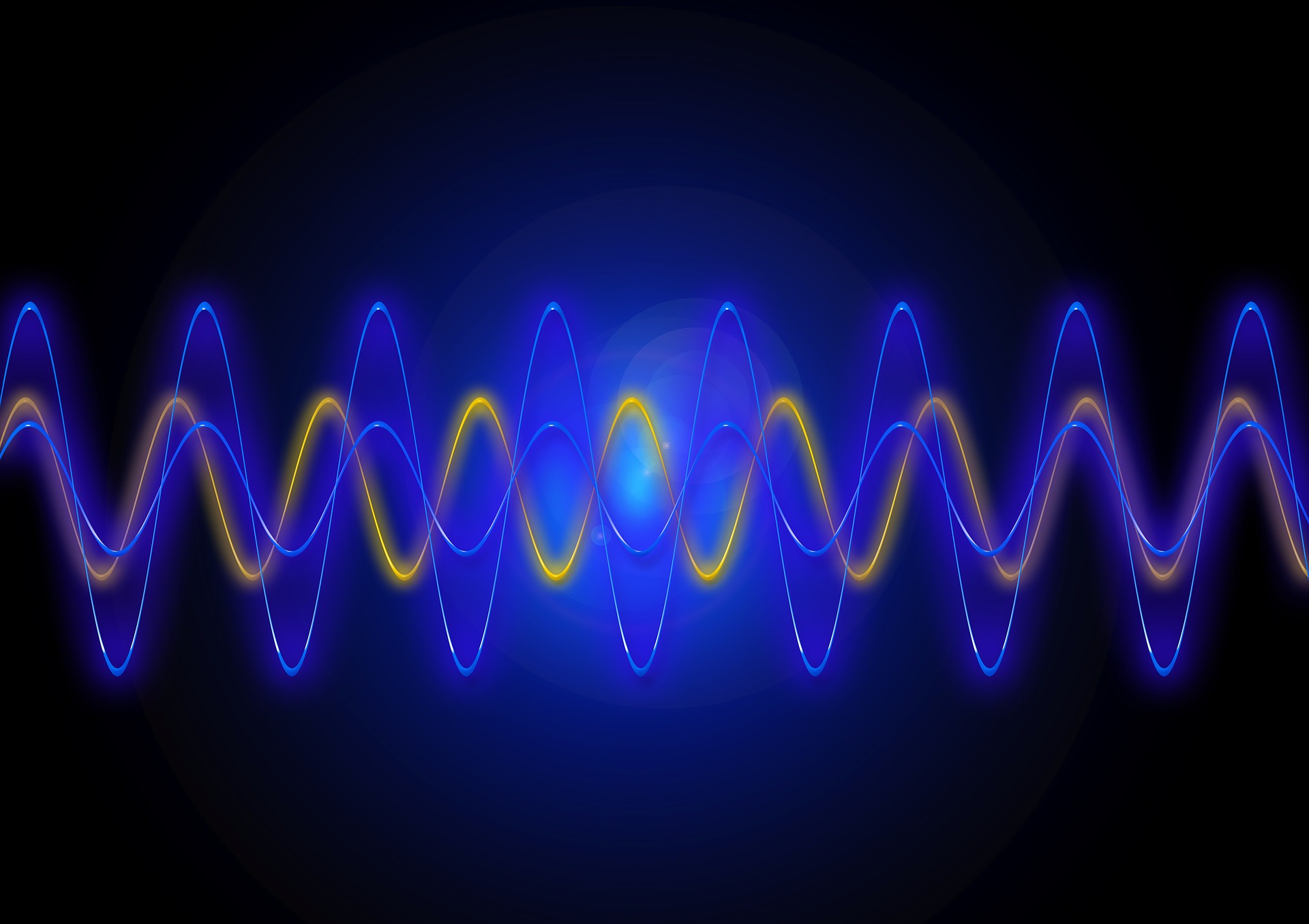
- Read more about MODALITY-DEPENDENT SENTIMENTS EXPLORING FOR MULTI-MODAL SENTIMENT CLASSIFICATION
- Log in to post comments
Recognizing human feelings from image and text is a core
challenge of multi-modal data analysis, often applied in personalized advertising. Previous works aim at exploring the
shared features, which are the matched contents between
images and texts. However, the modality-dependent sentiment information (private features) in each modality is usually ignored by cross-modal interactions, the real sentiment
is often reflected in one modality. In this paper, we propose a Modality-Dependent Sentiment Exploring framework
- Categories:
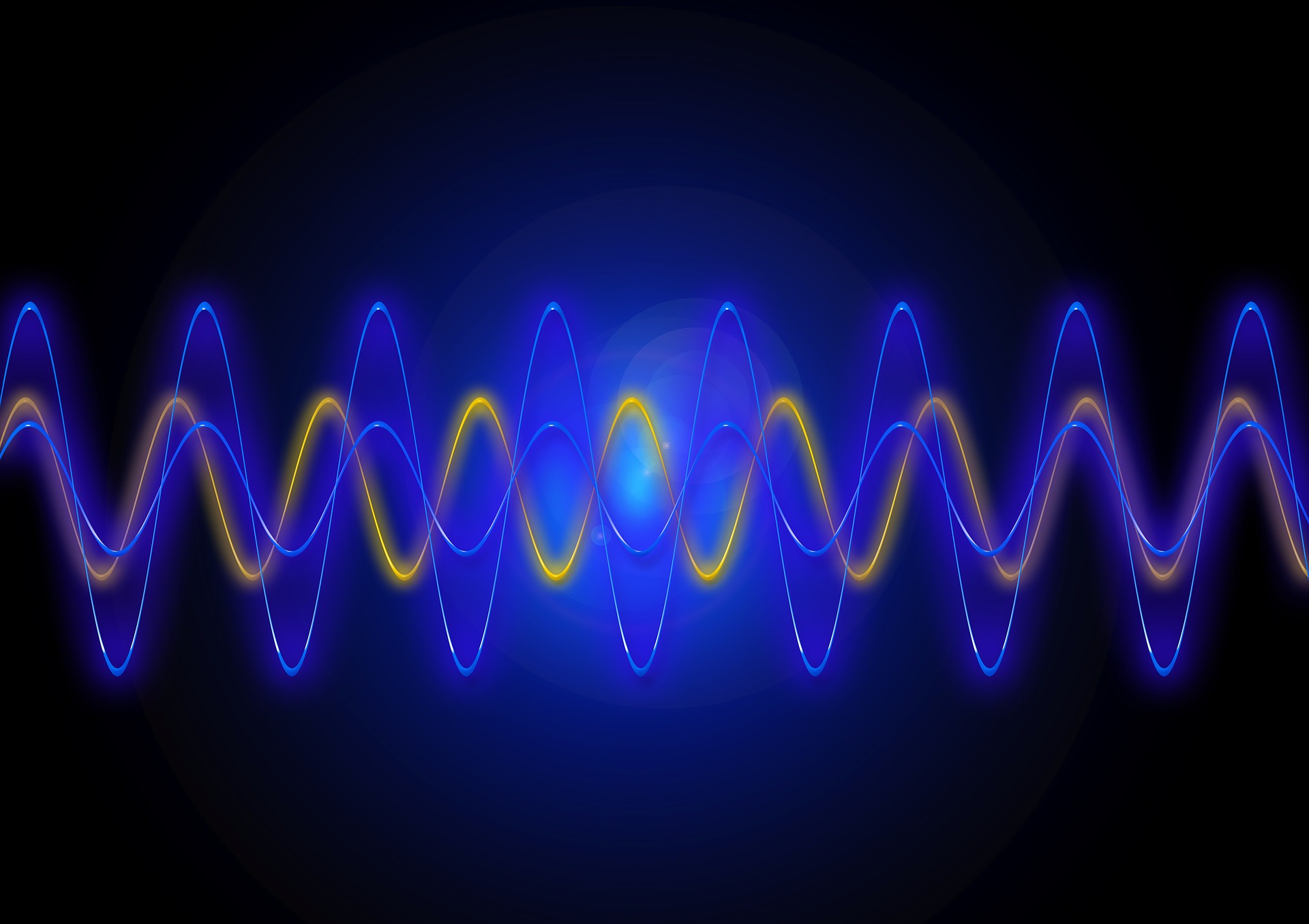
- Read more about SDRNET: SALIENCY-GUIDED DYNAMIC RESTORATION NETWORK FOR RAIN AND HAZE REMOVAL IN NIGHTTIME IMAGES
- Log in to post comments
Due to the different physical imaging models, most haze or rain removal methods for daytime images are not suitable for nighttime images. Fog effect produced by the accumu-lation of rain also brings great challenges to the restoration of lowlight nighttime images. To deal well with the multi-ple noise interference in this complex situation, we propose a saliency-guided dynamic restoration network (SDRNet) that can remove rain and haze in nighttime scenes. First, a saliency-guided detail enhancement preprocessing method is designed to get images with clearer details as the auxilia-ry input.
- Categories:
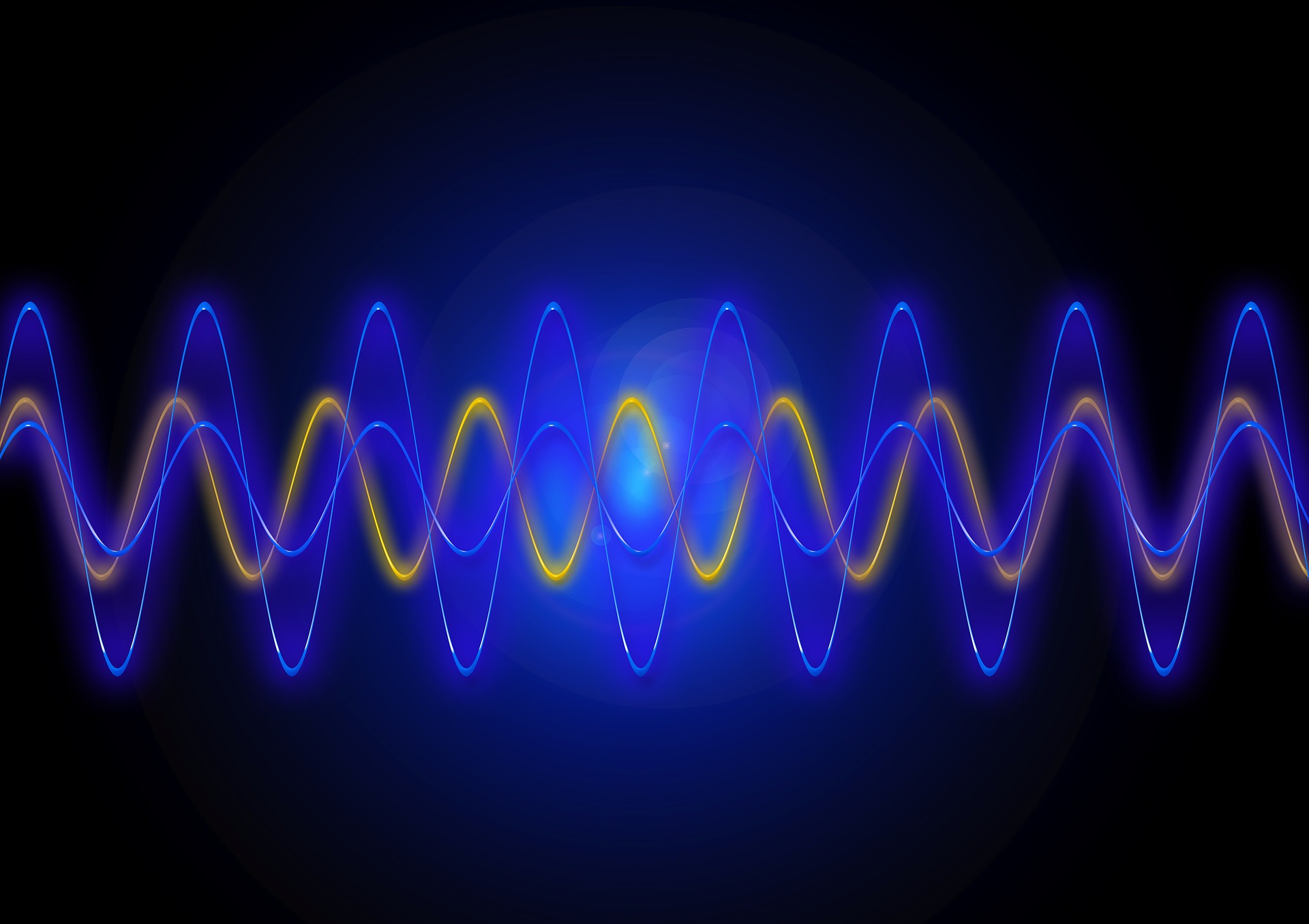
- Read more about MEMORY-AUGMENTED DUAL-DOMAIN UNFOLDING NETWORK FOR MRI RECONSTRUCTION
- Log in to post comments
The compressed sensing MRI aims to recover high-fidelity images from undersampled k-space data, which enables MRI acceleration and meanwhile mitigates problems caused by prolonged acquisition time, such as physiological motion artifacts, patient discomfort, and delayed medical care. In this regard, the deep unfolding network (DUN) has emerged as the predominant solution due to the benefits of better interpretability and model capacity. However, existing algorithms remain inadequate for two principal reasons.
- Categories:
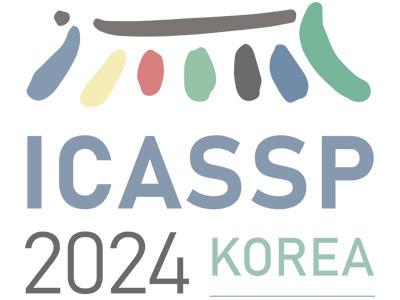
- Read more about TokenMotion: Motion-Guided Vision Transformer For Video Camouflaged Object Detection Via Learnable Token Selection
- Log in to post comments
The area of Video Camouflaged Object Detection (VCOD) presents unique challenges in the field of computer vision due to texture similarities between target objects and their surroundings, as well as irregular motion patterns caused by both objects and camera movement. In this paper, we introduce TokenMotion (TMNet), which employs a transformer-based model to enhance VCOD by extracting motion-guided features using a learnable token selection. Evaluated on the challenging MoCA-Mask dataset, TMNet achieves state-of-the-art performance in VCOD.
- Categories:
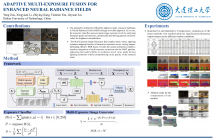
- Read more about Adaptive Multi-Exposure Fusion for Enhanced Neural Radiance Fields
- Log in to post comments
Neural Radiance Fields (NeRF) have revolutionized 3D scene modeling and rendering. However, their performance dips when handling images with diverse exposure levels, mainly due to the intricate luminance dynamics. Addressing this, we present an innovative method that proficiently models and renders images across a spectrum of exposure conditions. Our approach utilizes an unsupervised classifier-generator structure for HDR fusion, significantly enhancing NeRF's ability to comprehend and adjust to light variations, leading to the generation of images with appropriate brightness.
- Categories:
- Read more about Gravitated Latent Space Loss Generated by Metric Tensor for High-Dynamic Range Imaging
- Log in to post comments
High Dynamic Range (HDR) imaging seeks to enhance image quality by combining multiple Low Dynamic Range (LDR) images captured at varying exposure levels. Traditional deep learning approaches often employ reconstruction loss, but this method can lead to ambiguities in feature space during training. To address this issue, we present a new loss function, termed Gravitated Latent Space (GLS) loss, that leverages a metric tensor to introduce a form of virtual gravity within the latent space. This feature helps the model in overcoming saddle points more effectively.
- Categories: