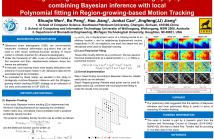
- Read more about Augmenting 3D Ultrasound Strain Elastography by combining Bayesian inference with local Polynomial fitting in Region-growing-based Motion Tracking
- Log in to post comments
Accurately tracking large tissue motion over a sequence of ultrasound images is critically important to several clinical applications including, but not limited to, elastography, flow imaging, and ultrasound-guided motion compensation. However, tracking in vivo large tissue deformation in 3D is a challenging problem and requires further developments. In this study, we explore a novel tracking strategy that combines Bayesian inference with local polynomial fitting.
ICIP2021.pdf

- Categories:
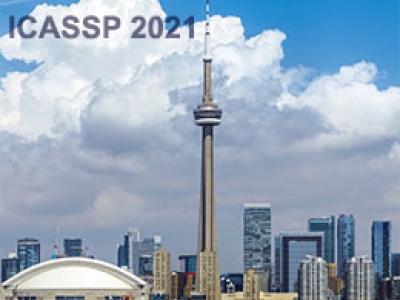
- Read more about Ultrasound elasticity imaging using physics-based models and learning-based plug-and-play priors
- Log in to post comments
Existing physical model-based imaging methods for ultrasound elasticity reconstruction utilize fixed variational regularizers that may not be appropriate for the application of interest or may not capture complex spatial prior information about the underlying tissues. On the other hand, end-to-end learning-based methods count solely on the training data, not taking advantage of the governing physical laws of the imaging system.
ICASSP_slides.pdf

ICASSP_poster.pdf

- Categories:
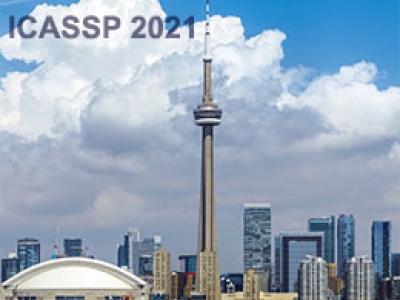
There is growing interest in the use of deep neural network
(DNN) based image denoising to reduce patient’s X-ray
dosage in medical computed tomography (CT). An effective
denoiser must remove noise while maintaining the texture
and detail. Commonly used mean squared error (MSE) loss
functions in the DNN training weight errors due to bias and
variance equally. However, the error due to bias is often more
egregious since it results in loss of image texture and detail.
In this paper, we present a novel approach to designing a loss
- Categories:
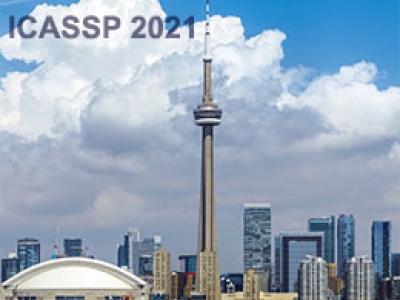
In this paper, we present a novel Image Fusion Model
(IFM) for ECG heart-beat classification to overcome the
weaknesses of existing machine learning techniques that rely
either on manual feature extraction or direct utilization of 1D
raw ECG signal. At the input of IFM, we first convert the
heart-beats of ECG into three different images using Gramian
Angular Field (GAF), Recurrence Plot (RP) and Markov
Transition Field (MTF) and then fuse these images to create
- Categories:
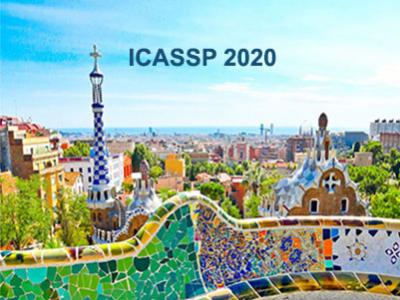
- Read more about Combining cGAN and MIL for Hotspot Segmentation in Bone Scintigraphy
- Log in to post comments
- Categories:
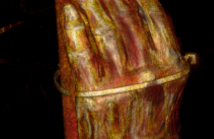
- Read more about Signal Sensing and Reconstruction Paradigms for a Novel Multi-source Static Computed Tomography System
- Log in to post comments
Conventional Computed Tomography (CT) systems use a single X-ray source and an arc of detectors mounted on a rotating gantry to acquire a set of projection data. Novel CT systems are now being pioneered in which a complete ring of distributed X-ray sources and detectors are electronically turned on and off, without any mechanical motion, to acquire a set of projections for tomographic reconstruction. This paper discusses new sensing and reconstruction paradigms enabled by this new CT architecture.
- Categories:
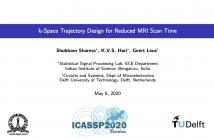
The development of compressed sensing (CS) techniques for magnetic resonance imaging (MRI) is enabling a speedup of MRI scanning. To increase the incoherence in the sampling, a random selection of points on the k-space is deployed and a continuous trajectory is obtained by solving a traveling salesman problem (TSP) through these points. A feasible trajectory satisfying the gradient constraints is then obtained by parameterizing it using state-of-the-art methods. In this paper, a constrained convex optimization based method to obtain feasible trajectories is proposed.
- Categories:
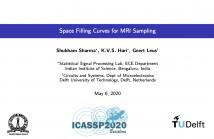
- Read more about SPACE FILLING CURVES FOR MRI SAMPLING
- Log in to post comments
A novel class of k-space trajectories for magnetic resonance imaging (MRI) sampling using space-filling curves (SFCs) is presented here. More specifically, Peano, Hilbert and Sierpinski curves are used. We propose 1-shot and 4-shot variable density SFCs by utilizing the space coverage provided by SFCs in different iterations. The proposed trajectories are compared with state-of-the-art echo-planar imaging (EPI) trajectories for 128 × 128 and 256 × 256 phantom and brain images.
- Categories:
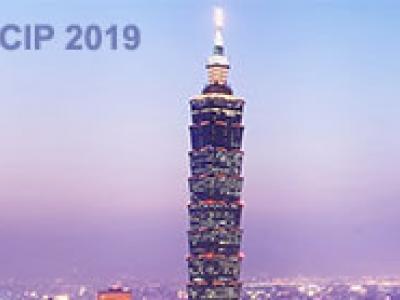
- Read more about Generation of head models for brain stimulation using deep convolution networks
- Log in to post comments
Transcranial magnetic stimulation (TMS) is a non-invasive clinical technique used for treatment of several neurological diseases such as depression, Alzheimer’s disease and Parkinson’s disease. However, it is always challenging to accurately adjust the electric field on different specific brain regions due to the requirement of several stimulation parameters’ optimizations.
- Categories:
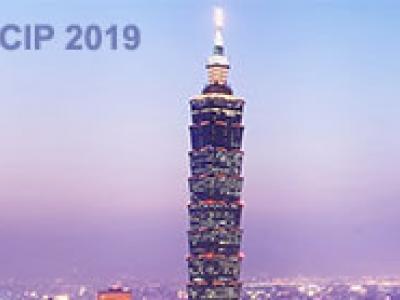
- Read more about COMPRESSED SENSING MRI WITH JOINT IMAGE-LEVEL AND PATCH-LEVEL PRIORS
- Log in to post comments
- Categories: