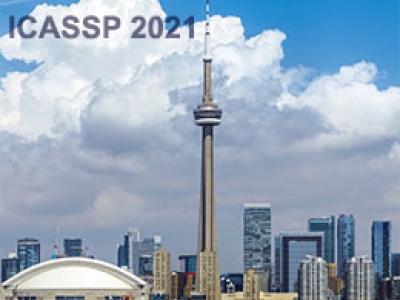
- Read more about Slides: Transcription Is All You Need: Learning To Separate Musical Mixtures With Score As Supervision
- Log in to post comments
Most music source separation systems require large collections of isolated sources for training, which can be difficult to obtain. In this work, we use musical scores, which are comparatively easy to obtain, as a weak label for training a source separation system. In contrast with previous score-informed separation approaches, our system does not require isolated sources, and score is used only as a training target, not required for inference.
- Categories:
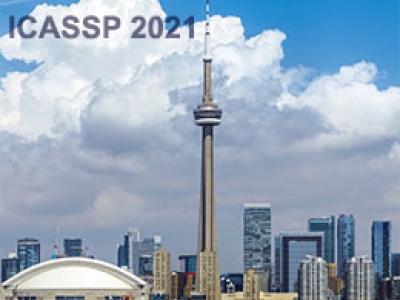
- Read more about Poster: Transcription Is All You Need: Learning To Separate Musical Mixtures With Score As Supervision
- Log in to post comments
Most music source separation systems require large collections of isolated sources for training, which can be difficult to obtain. In this work, we use musical scores, which are comparatively easy to obtain, as a weak label for training a source separation system. In contrast with previous score-informed separation approaches, our system does not require isolated sources, and score is used only as a training target, not required for inference.
- Categories:
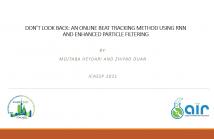
- Read more about DON’T LOOK BACK: AN ONLINE BEAT TRACKING METHOD USING RNN AND ENHANCED PARTICLE FILTERING
- Log in to post comments
Online beat tracking (OBT) has always been a challenging task. Due to the inaccessibility of future data and the need to make inference in real-time. We propose Don’t Look back! (DLB), a novel approach optimized for efficiency when performing OBT. DLB feeds the activations of a unidirectional RNN into an enhanced Monte-Carlo localization model to infer beat positions. Most preexisting OBT methods either apply some offline approaches to a moving window containing past data to make predictions about future beat positions or must be primed with past data at startup to initialize.
- Categories:
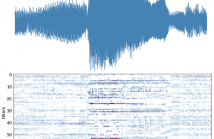
- Read more about Deep Convolutional and Recurrent Networks for Polyphonic Instrument Classification from Monophonic Raw Audio Waveforms
- Log in to post comments
Sound Event Detection and Audio Classification tasks are traditionally addressed through time-frequency representations of audio signals such as spectrograms. However, the emergence of deep neural networks as efficient feature extractors has enabled the direct use of audio signals for classification purposes. In this paper, we attempt to recognize musical instruments in polyphonic audio by only feeding their raw waveforms into deep learning models.
- Categories:
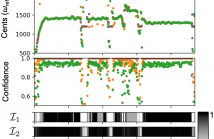
- Read more about RELIABILITY ASSESSMENT OF SINGING VOICE F0-ESTIMATES USING MULTIPLE ALGORITHMS
- Log in to post comments
Over the last decades, various conceptually different approaches for fundamental frequency (F0) estimation in monophonic audio recordings have been developed. The algorithms’ performances vary depending on the acoustical and musical properties of the input audio signal. A common strategy to assess the reliability (correctness) of an estimated F0-trajectory is to evaluate against an annotated reference. However, such annotations may not be available for a particular audio collection and are typically laborintensive to generate.
- Categories:
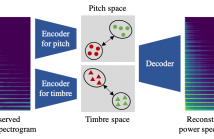
- Read more about Pitch-Timbre Disentanglement of Musical Instrument Sounds Based on VAE-Based Metric Learning
- Log in to post comments
This paper describes a representation learning method for disentangling an arbitrary musical instrument sound into latent pitch and timbre representations. Although such pitch-timbre disentanglement has been achieved with a variational autoencoder (VAE), especially for a predefined set of musical instruments, the latent pitch and timbre representations are outspread, making them hard to interpret.
- Categories:
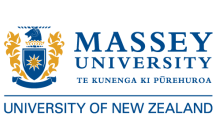
- Read more about DEEPF0: END-TO-END FUNDAMENTAL FREQUENCY ESTIMATION FOR MUSIC AND SPEECH SIGNALS
- Log in to post comments
We propose a novel pitch estimation technique called DeepF0, which leverages the available annotated data to directly learns from the raw audio in a data-driven manner. F0 estimation is important in various speech processing and music information retrieval applications. Existing deep learning models for pitch estimations have relatively limited learning capabilities due to their shallow receptive field. The proposed model addresses this issue by extending the receptive field of a network by introducing the dilated convolutional blocks into the network.
- Categories:
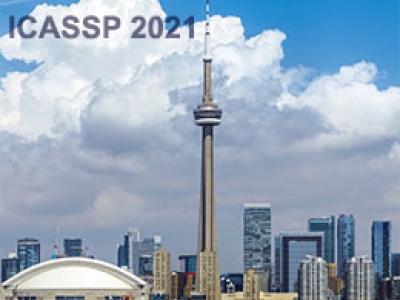
- Read more about Melon Playlist Dataset: A Public Dataset For Audio-based Playlist Generation And Music Tagging
- Log in to post comments
One of the main limitations in the field of audio signal processing is the lack of large public datasets with audio representations and high-quality annotations due to restrictions of copyrighted commercial music. We present Melon Playlist Dataset, a public dataset of mel-spectrograms for 649,091 tracks and 148,826 associated playlists annotated by 30,652 different tags. All the data is gathered from Melon, a popular Korean streaming service. The dataset is suitable for music information retrieval tasks, in particular, auto-tagging and automatic playlist continuation.
icassp2021.pdf

- Categories:
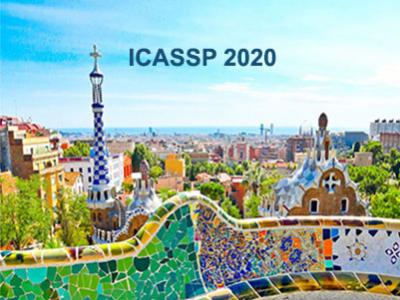
- Read more about Learning to rank music tracks using triplet loss
- Log in to post comments
Most music streaming services rely on automatic recommendation algorithms to exploit their large music catalogs. These algorithms aim at retrieving a ranked list of music tracks based on their similarity with a target music track. In this work, we propose a method for direct recommendation based on the audio content without explicitly tagging the music tracks. To that aim, we propose several strategies to perform triplet mining from ranked lists. We train a Convolutional Neural Network to learn the similarity via triplet loss.
icassp.pdf

- Categories:
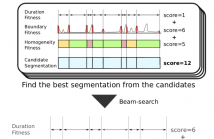
- Read more about MUSIC BOUNDARY DETECTION BASED ON A HYBRID DEEP MODEL OF NOVELTY, HOMOGENEITY, REPETITION AND DURATION
- Log in to post comments
Current state-of-the-art music boundary detection methods use local features for boundary detection, but such an approach fails to explicitly incorporate the statistical properties of the detected segments. This paper presents a music boundary detection method that simultaneously considers a fitness measure based on the boundary posterior probability, the likelihood of the segmentation duration sequence, and the acoustic consistency within a segment.
- Categories: