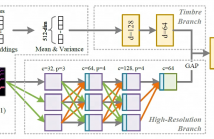
- Read more about TG-Critic: A Timbre-Guided Model for Reference-Independent Singing Evaluation
- Log in to post comments
Automatic singing evaluation independent of reference melody is a challenging task due to its subjective and multi-dimensional nature. As an essential attribute of singing voices, vocal timbre has a non-negligible effect and influence on human perception of singing quality. However, no research has been done to include timbre information explicitly in singing evaluation models. In this paper, a data-driven model TG-Critic is proposed to introduce timbre embeddings as one of the model inputs to guide the evaluation of singing quality.
- Categories:
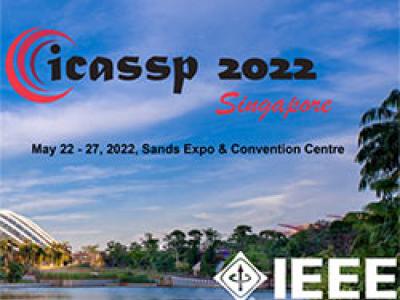
- Read more about Longshen Ou ICASSP 2022 Exploring Transformer's Potential on Automatic Piano Transcription
- Log in to post comments
- Categories:
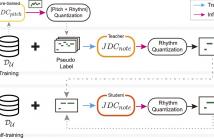
- Read more about PSEUDO-LABEL TRANSFER FROM FRAME-LEVEL TO NOTE-LEVEL IN A TEACHER-STUDENT FRAMEWORK FOR SINGING TRANSCRIPTION FROM POLYPHONIC MUSIC
- Log in to post comments
Lack of large-scale note-level labeled data is the major obstacle to singing transcription from polyphonic music. We address the issue by using pseudo labels from vocal pitch estimation models given unlabeled data. The proposed method first converts the frame-level pseudo labels to note-level through pitch and rhythm quantization steps. Then, it further improves the label quality through self-training in a teacher-student framework.
- Categories:
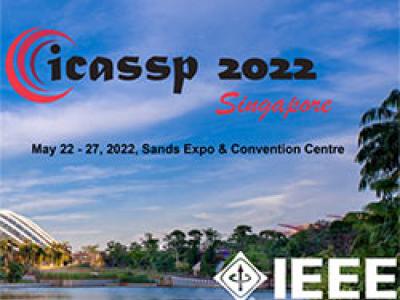
- Read more about SCORE DIFFICULTY ANALYSIS FOR PIANO PERFORMANCE EDUCATION BASED ON FINGERING
- Log in to post comments
In this paper, we introduce score difficulty classification as a subtask of music information retrieval (MIR), which may be used in music education technologies, for personalised curriculum generation, and score retrieval. We introduce a novel dataset for our task, Mikrokosmos-difficulty, containing 147 piano pieces in symbolic representation and the corresponding difficulty labels derived by its composer Béla Bartók and the publishers. As part of our methodology, we propose piano technique feature representations based on different piano fingering algorithms.
- Categories:
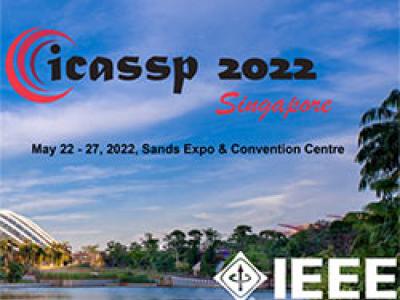
- Read more about A Few-sample Strategy for Guitar Tablature Transcription Based on Inharmonicity Analysis and Playability Constraints
- Log in to post comments
The prominent strategical approaches regarding the problem of guitar tablature transcription rely either on fingering patterns encoding or on the extraction of string-related audio features. The current work combines the two aforementioned strategies in an explicit manner by employing two discrete components for string-fret classification. It extends older few-sample modeling strategies by introducing various adaptation schemes for the first stage of audio processing, taking advantage of the inharmonic characteristics of guitar sound.
- Categories:
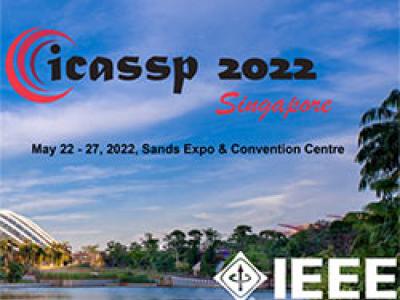
- Read more about Genre-conditioned Acoustic Models for Automatic Lyrics Transcription of Polyphonic Music
- Log in to post comments
Lyrics transcription of polyphonic music is challenging not only because the singing vocals are corrupted by the background music, but also because the background music and the singing style vary across music genres, such as pop, metal, and hip hop, which affects lyrics intelligibility of the song in different ways. In this work, we propose to transcribe the lyrics of polyphonic music using a novel genre-conditioned network.
- Categories:
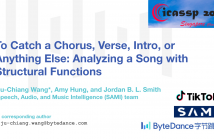
- Read more about TO CATCH A CHORUS, VERSE, INTRO, OR ANYTHING ELSE: ANALYZING A SONG WITH STRUCTURAL FUNCTIONS
- Log in to post comments
Conventional music structure analysis algorithms aim to divide a song into segments and to group them with abstract labels (e.g., ‘A’, ‘B’, and ‘C’). However, explicitly identifying the function of each segment (e.g., ‘verse’ or ‘chorus’) is rarely attempted, but has many applications. We introduce a multi-task deep learning framework to model these structural semantic labels directly from audio by estimating "verseness," "chorusness," and so forth, as a function of time.
- Categories:
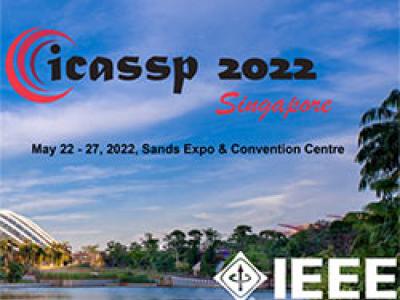
- Read more about The Mirrornet : Learning Audio Synthesizer Controls Inspired by Sensorimotor Interaction
- Log in to post comments
Experiments to understand the sensorimotor neural interactions in the human cortical speech system support the existence of a bidirectional flow of interactions between the auditory and motor regions. Their key function is to enable the brain to ‘learn’ how to control the vocal tract for speech production. This idea is the impetus for the recently proposed "MirrorNet", a constrained autoencoder architecture.
- Categories:
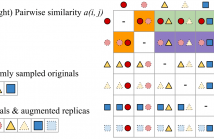
- Read more about Neural Audio Fingerprint for High-specific Audio Retrieval based on Contrastive Learning
- Log in to post comments
Most of existing audio fingerprinting systems have limitations to be used for high-specific audio retrieval at scale. In this work, we generate a low-dimensional representation from a short unit segment of audio, and couple this fingerprint with a fast maximum inner-product search. To this end, we present a contrastive learning framework that derives from the segment-level search objective. Each update in training uses a batch consisting of a set of pseudo labels, randomly selected original samples, and their augmented replicas.
- Categories:
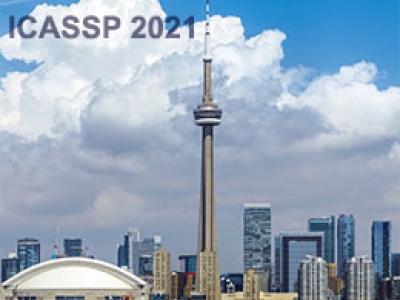
The identification of structural differences between a music performance and the score is a challenging yet integral step of audio-to-score alignment, an important subtask of music signal processing. We present a novel method to detect such differences between the score and performance for a given piece of music using progressively dilated convolutional neural networks. Our method incorporates varying dilation rates at different layers to capture both short-term and long-term context, and can be employed successfully in the presence of limited annotated data.
- Categories: