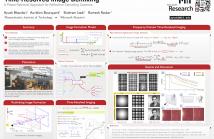
- Read more about TIME-RESOLVED IMAGE DEMIXING
- Log in to post comments
When multiple light paths combine at a given location on an image sensor, an image mixture is created. Demixing or recovering the original constituent components in such cases is a highly ill--posed problem. A number of elegant solutions have thus been developed in the literature, relying on measurement diversity such as polarization, shift, motion, or scene features.
- Categories:
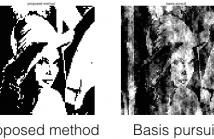
This is the IEEE SPL presentation of the author's paper:
M. Nagahara, "Discrete Signal Reconstruction by Sum of Absolute Values," in IEEE Signal Processing Letters, vol. 22, no. 10, pp. 1575-1579, Oct. 2015.
doi: 10.1109/LSP.2015.2414932
URL: http://ieeexplore.ieee.org/stamp/stamp.jsp?tp=&arnumber=7064695&isnumber...
icassp2016.pdf

- Categories:
- Read more about Sparse Phase Retrieval with Near Minimal Measurements: A Structured Sampling Based Approach
- Log in to post comments
- Categories:
- Read more about Bandlimited Field Reconstruction from Samples obtained on a Discrete Grid with Unknown Random Locations
- Log in to post comments
Sampling spatial fields using sensors which are location unaware is an exciting topic. Due to symmetry and shift invariance of bandlimited fields, it is known that uniformly distributed location-unaware sensors cannot infer the field. This work studies asymmetric (nonuniform) distributions on location-unaware sensors that will enable bandlimited field inference. In this first exposition, to facilitate analysis, location-unaware sensors are restricted to a discrete grid. Oversampling is used to overcome the lack of location information.
- Categories:
- Read more about SPARSITY-BASED RECONSTRUCTION METHOD FOR SIGNALS WITH FINITE RATE OF INNOVATION
- Log in to post comments
In the last decade, it was shown that it is possible to reconstruct signals with finite rate of innovation (FRI signals) from the samples of their filtered versions. However, when noise is present, the present reconstruction algorithms tend to be low accuracy. In this work, a new sparsity-based reconstruction method for FRI signals is put forward. The streams of Diracs and exponential reproducing kernel are considered. Firstly, the analog time axis is quantified and aligned to grids.
- Categories:
- Read more about INDEPENDENT VERSUS REPEATED MEASUREMENTS: A PERFORMANCE QUANTIFICATION VIA STATE EVOLUTION
- Log in to post comments
The paper quantifies and compares the exact asymptotic performance of multiple measurement vector (MMV) and distributed sensing (DS) models. Both models assume multiple measurement instances y_k = A_kx_k + w_k; k = 1,2,...,K. The difference is that MMV involves identical measurement matrices whereas DS allows different matrices for different measurement instances. It has been recognized that DS works better than MMV empirically. However, the quantification of the performance difference is not available in the literature.
ICASSP2016.pdf

- Categories:
- Read more about 1-Bit Compressed Sensing Of Positive Semi-Definite Matrices Via Rank-1 Measurement Matrices
- Log in to post comments
In this paper, we investigate the problem of recovering positive semi-definite (PSD) matrix from 1-bit sensing. The measurement matrix is rank-1 and constructed by the outer product of a pair of vectors, whose entries are independent and identically distributed (i.i.d.) Gaussian variables. The recovery problem is solved in closed form through a convex programming. Our analysis reveals that the solution is biased in general. However, in case of error-free measurement, we find that for rank-r PSD matrix with bounded condition number, the bias decreases with an order of O(1/r).
- Categories:
- Read more about Sparse Phase Retrieval Using Partial Nested Fourier Samplers
- Log in to post comments
- Categories:
- Read more about Fast Sparse Recovery via Non-Convex Optimization
- Log in to post comments
slides.pdf

- Categories:
- Categories: