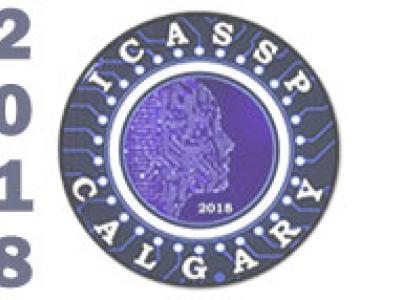
- Read more about Combining MatrixOn the SNR Variability in Noisy Compressed Sensing
- Log in to post comments
Compressed sensing (CS) is a sampling paradigm
that allows to simultaneously measure and compress signals that
are sparse or compressible in some domain. The choice of a
sensing matrix that carries out the measurement has a defining
impact on the system performance and it is often advocated to
draw its elements randomly. It has been noted that in the presence
of input (signal) noise, the application of the sensing matrix causes
SNR degradation due to the noise folding effect. In fact, it might
- Categories:
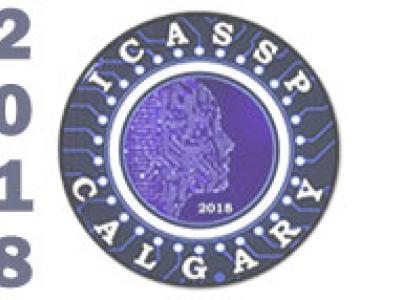
- Read more about Greedy Algorithm With Approximation Ratio For Sampling Noisy Graph Signals
- Log in to post comments
We study the optimal sampling set selection problem in sampling a noisy $k$-bandlimited graph signal. To minimize the effect of noise when trying to reconstruct a $k$-bandlimited graph signal from $m$ samples, the optimal sampling set selection problem has been shown to be equivalent to finding a $m \times k$ submatrix with the maximum smallest singular value, $\sigma_{\min}$ \cite{chen2015discrete}. As the problem is NP-hard, we present a greedy algorithm inspired by a similar submatrix selection problem known in computer science and to which we add a local search refinement.
- Categories:
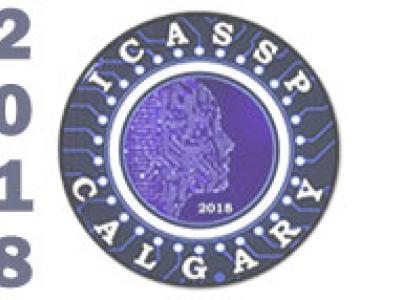
- Read more about SOLVING LINEAR INVERSE PROBLEMS USING GAN PRIORS: AN ALGORITHM WITH PROVABLE GUARANTEES
- Log in to post comments
In recent works, both sparsity-based methods as well as learning-based methods have proven to be successful in solving several challenging linear inverse problems. However, sparsity priors for natural signals and images suffer from poor discriminative capability, while learning-based methods seldom provide concrete theoretical guarantees. In this work, we advocate the idea of replacing hand-crafted priors, such as sparsity, with a Generative Adversarial Network (GAN) to solve linear inverse problems such as compressive sensing.
- Categories:
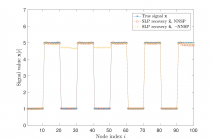
- Read more about The Network Nullspace Property for Compressed Sensing of Big Data over Networks
- Log in to post comments
- Categories:
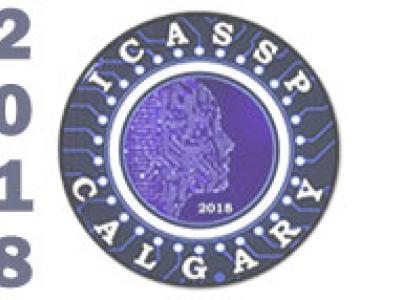
Self-reset analog-to-digital converters (ADCs) allow for digitization of a signal with a high dynamic range. The reset action is equivalent to a modulo operation performed on the signal. We consider the problem of recovering the original signal from the measured modulo-operated signal. In our formulation, we assume that the underlying signal is Lipschitz continuous. The modulo-operated signal can be expressed as the sum of the original signal and a piecewise-constant signal that captures the transitions. The reconstruction requires estimating the piecewise-constant signal.
- Categories:
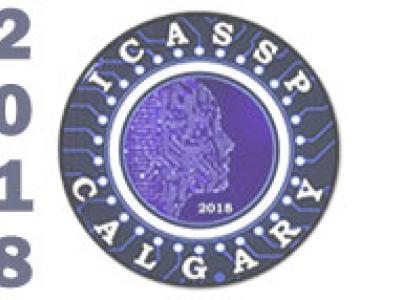
Self-reset analog-to-digital converters (ADCs) allow for digitization of a signal with a high dynamic range. The reset action is equivalent to a modulo operation performed on the signal. We consider the problem of recovering the original signal from the measured modulo-operated signal. In our formulation, we assume that the underlying signal is Lipschitz continuous. The modulo-operated signal can be expressed as the sum of the original signal and a piecewise-constant signal that captures the transitions. The reconstruction requires estimating the piecewise-constant signal.
- Categories:
- Read more about DESIGN OF SAMPLING SET FOR BANDLIMITED GRAPH SIGNAL ESTIMATION
- Log in to post comments
It is of particular interest to reconstruct or estimate bandlimited graph signals, which are smoothly varying signals defined over graphs, from partial noisy measurements. However, choosing an optimal subset of nodes to sample is NP-hard. We formularize the problem as the experimental design of a linear regression model if we allow multiple measurements on a single node. By relaxing it to a convex optimization problem, we get the proportion of sample for each node given the budget of total sample size. Then, we use a probabilistic quantization to get the number of each node to be sampled.
- Categories:
- Read more about Phase Retrieval Based Deconvolution Algorithm in Optical Systems
- Log in to post comments
In an optical imaging system, the retrieved image of an object is blurred by the point spread function (PSF) of the system,and cannot exactly represent the object. Deconvolution is an effective method to recover the object from the blurred image and improve the resolution of the optical system. But in real optical system, the detector only measures the intensity of the light, not the phase.
- Categories:
- Read more about REGULARIZED SELECTION: A NEW PARADIGM FOR INVERSE BASED REGULARIZED IMAGE RECONSTRUCTION TECHNIQUES
- Log in to post comments
- Categories: