- Communication and Sensing aspects of Sensor Networks, Wireless and Ad-Hoc Networks
- Communication Systems and Applications
- MIMO Communications and Signal Processing
- Signal Transmission and Reception
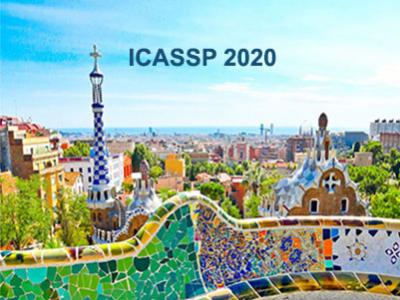
- Read more about Joint Sparse Recovery using Deep Unfolding With Application to Massive Random Access
- Log in to post comments
- Categories:
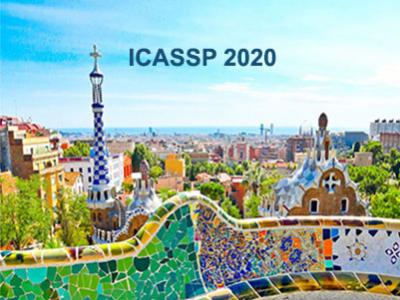
- Read more about Proximal Multitask Learning Over Distributed Networks with Jointly Sparse Structure
- Log in to post comments
- Categories:
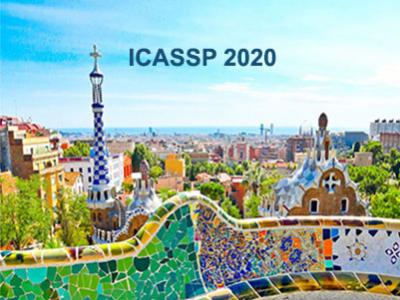
- Read more about Proximal Multitask Learning Over Distributed Networks with Jointly Sparse Structure
- Log in to post comments
- Categories:
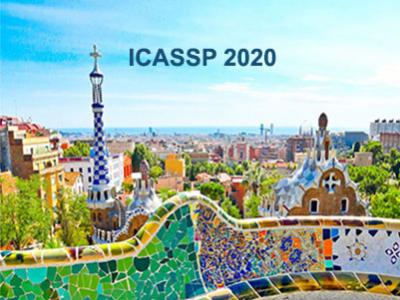
- Read more about A LEARNING APPROACH TO COOPERATIVE COMMUNICATION SYSTEM DESIGN
- Log in to post comments
The cooperative relay network is a type of multi-terminal communication system. We present in this paper a Neural Network (NN)-based autoencoder (AE) approach to optimize its design. This approach implements a classical three-node cooperative system as one AE model, and uses a two-stage scheme to train this model and minimize the designed losses. We demonstrate that this approach shows performance close to the best baseline in decode-and-forward (DF), and outperforms the best baseline in amplify-and-forward (AF), over a wide range of signal-to-noise-ratio (SNR) values.
- Categories:
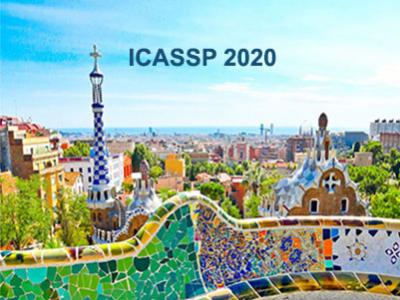
- Read more about Robust Hybrid Beamforming for Satellite-Terrestrial Integrated Networks
- 1 comment
- Log in to post comments
- Categories:
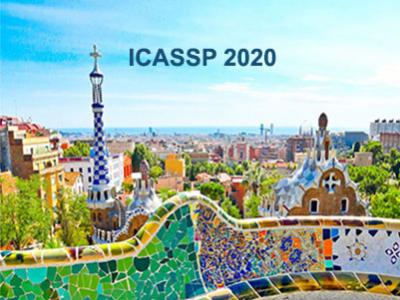
The problem of maximizing the information flow through a sensor network tasked with an inference objective at the fusion center is considered. The sensor nodes take observations, compress, and send them to the fusion center through a network of relays. The network imposes capacity constraints on the rate of transmission in each connection and flow conservation constraints. It is shown that this rate-constrained inference problem can be cast as a Network Utility Maximization problem by suitably defining the utility functions for each sensor, and can be solved using existing techniques.
- Categories:
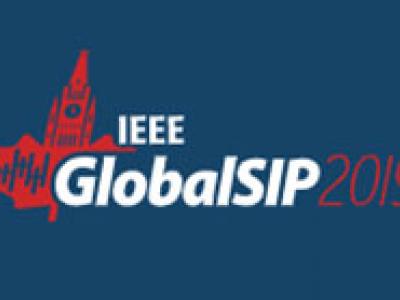
- Read more about Beam Alignment-Based mmWave Spectrum Sensing in Cognitive Vehicular Networks
- Log in to post comments
Millimeter wave (mmWave) communication is a promising technology to alleviate the shortage of spectrum resources in vehicular networks. To use mmWave spectrum resources more efficiently, in this paper we propose a novel beam alignment-based vehicular mmWave spectrum sensing model and algorithm. We first establish the spectrum sensing model on the basis of characteristics of mmWave signals and then derive the test statistics.
- Categories:
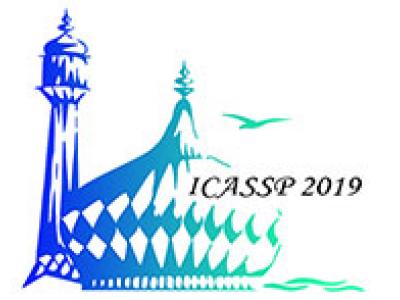
- Read more about Provably Accelerated Randomized Gossip Algorithms
- Log in to post comments
In this work we present novel provably accelerated gossip algorithms for solving the average consensus problem. The proposed protocols are inspired from the recently developed accelerated variants of the randomized Kaczmarz method - a popular method for solving linear systems. In each gossip iteration all nodes of the network update their values but only a pair of them exchange their private information. Numerical experiments on popular wireless sensor networks showing the benefits of our protocols are also presented.
- Categories:
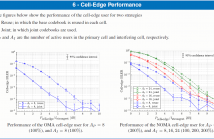
- Read more about Joint Codebook Design for Multi-Cell NOMA
- Log in to post comments
For spreading-based multiple access, whether orthogonal (OMA) or non-orthogonal (NOMA), the spreading sequences (signatures) are selected from a predefined codebook. When operating in a cellular system, intercell interference will be inherently present between close base stations that share the same resources. If the codebook is reused across the different cells, then intercell interference can cause a full collision of the interfering users in the code-domain, thus deteriorating their performance, especially those at the cell-edge.
- Categories:
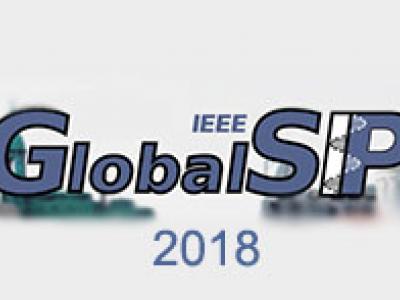
- Read more about Polar Feature Based Deep Architectures for Automatic Modulation Classification Considering Channel Fading
- Log in to post comments
To develop intelligent receivers, automatic modulation classification (AMC) plays an important role for better spectrum utilization. The emerging deep learning (DL) technique has received much attention in AMC due to its superior performance in classifying data with deep structure. In this work, a novel polar-based deep learning architecture with channel compensation network (CCN) is proposed. Our test results show that learning features from polar domain (r-θ) can improve recognition accuracy by 5% and reduce training overhead by 48%.
- Categories: