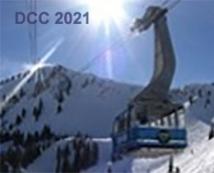
DCC 2021Virtual Conference - The Data Compression Conference (DCC) is an international forum for current work on data compression and related applications. Both theoretical and experimental work are of interest. Visit DCC 2021 website.
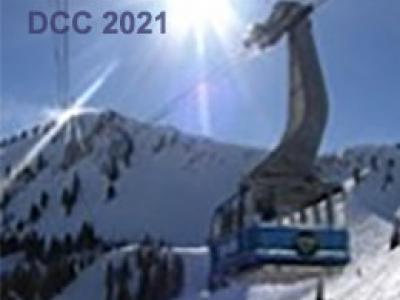
- Read more about Lossless Compression for Video Streams with Frequency Prediction and Macro Block Merging
- Log in to post comments
Cloud service has been emerging as a promising alternative to handle massive volumes of video sequences triggered by increasing demands on video service, especially surveillance and entertainment. Lossless compression of encoded video bitstreams can further eliminate the redundancies without altering the contents and facilitate the efficiency of cloud storage. In this paper, we propose a novel lossless compression scheme to further compress the video bitstreams generated by the state-of-the-art hybrid coding frameworks like H.264/AVC and HEVC.
- Categories:
- Read more about Modulated Variable-Rate Deep Video Compression
- Log in to post comments
In this work, we propose a variable-rate scheme for deep video compression, which can achieve continuously variable rate by a single model. The key idea is to use the R-D tradeoff parameter \(\lambda\) as the conditional parameter to control the bitrate. The scheme is developed on DVC, which jointly learns motion estimation, motion compression, motion compensation, and residual compression functions. In this framework, the motion and residual compression auto-encoders are critical for the rate adaptation because they generate the final bitstream directly.
- Categories:
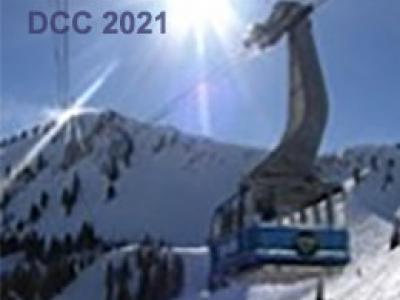
- Read more about Hybrid Intra-Prediction in Lossless Video Coding using Overfitted Neural Networks
- Log in to post comments
- Categories:
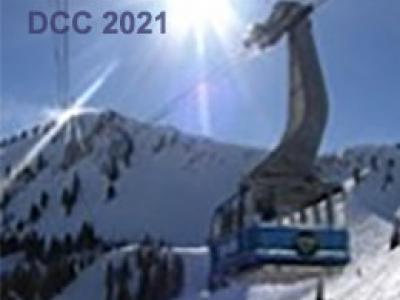
- Categories:
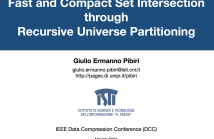
- Read more about Fast and Compact Set Intersection through Recursive Universe Partitioning
- 4 comments
- Log in to post comments
We present a data structure that encodes a sorted integer sequence in small space allowing, at the same time, fast intersection operations. The data layout is carefully designed to exploit word-level parallelism and SIMD instructions, hence providing good practical performance. The core algorithmic idea is that of recursive partitioning the universe of representation: a markedly different paradigm than the widespread strategy of partitioning the sequence based on its length.
- Categories:
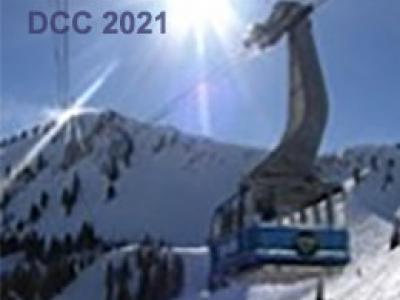
- Read more about Convolutional Neural Network for Image Compression with Application to JPEG Standard
- Log in to post comments
In this paper the authors present a novel structure of convolutional neural network for lossy image compression intended for use as a part of JPEG’s standard image compression stream. The network is trained on randomly selected images from high-quality image dataset of human faces and its effectiveness is verified experimentally using standard test images.
- Categories:
- Read more about HH-CompWordNet: Holistic Handwritten Word Recognition in the Compressed Domain
- Log in to post comments
Holistic word recognition in handwritten documents is an important research topic in the field of Document Image Analysis. For some applications, given strong language models, it can be more robust and computationally less expensive than character segmentation and recognition. This paper presents HH-CompWordNet, a novel approach to applying a Convolutional Neural Network (CNN) to directly to the DCT coefficients of the compressed domain word images.
- Categories:
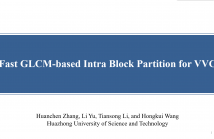
- Read more about Fast GLCM-based Intra Block Partition for VVC
- Log in to post comments
In the latest video coding standard, Versatile Video Coding (H.266/VVC), a new quadtree with nested multi-type tree (QTMT) coding block structure is proposed. QTMT significantly improves coding performance, but more complex block partitioning structure brings greater computational burden. To solve this problem, a fast intra block partition pattern pruning algorithm is proposed using gray level co-occurrence matrix (GLCM) to calculate texture direction information of coding units, terminating the horizontal or vertical split of the binary tree and the ternary tree in advance.
- Categories:
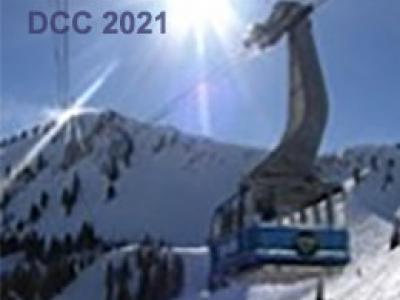
- Read more about On Random Editing in LZ-End
- 1 comment
- Log in to post comments
LZ-End is a variant of the LZ77 compression algorithm which allows random access to the compressed data. In this paper, we show how the random-access capability of LZ-End allows random edits to the compressed data, which is the first algorithm to randomly edit strings compressed by a Lempel-Ziv algorithm.
- Categories:
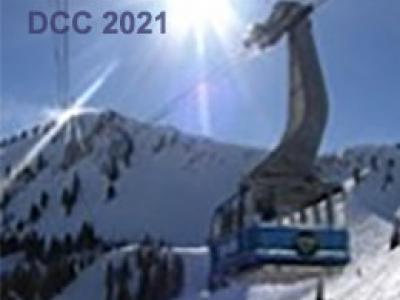
- Read more about Lossy Compression for Integrating Event Sensors
- Log in to post comments
- Categories: