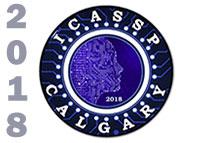
ICASSP is the world’s largest and most comprehensive technical conference focused on signal processing and its applications. The 2019 conference will feature world-class presentations by internationally renowned speakers, cutting-edge session topics and provide a fantastic opportunity to network with like-minded professionals from around the world. Visit website.
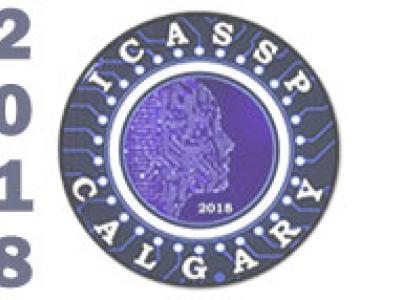
- Categories:
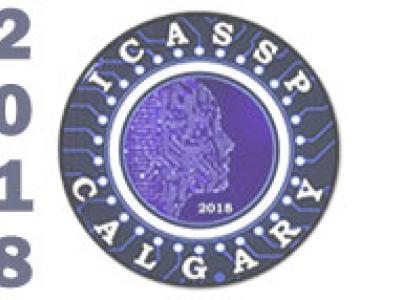
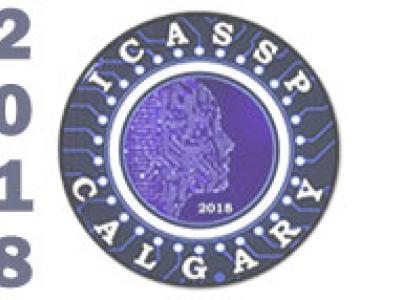
- Read more about BirdVox-full-night: a dataset and website for avian flight call detection.
- Log in to post comments
This article addresses the automatic detection of vocal, nocturnally migrating birds from a network of acoustic sensors.
Thus far, owing to the lack of annotated continuous recordings, existing methods had been benchmarked in a binary classification setting (presence vs. absence).
Instead, with the aim of comparing them in event detection, we release BirdVox-full-night, a dataset of 62 hours of audio comprising 35402 flight calls of nocturnally migrating birds, as recorded from 6 sensors.
- Categories:
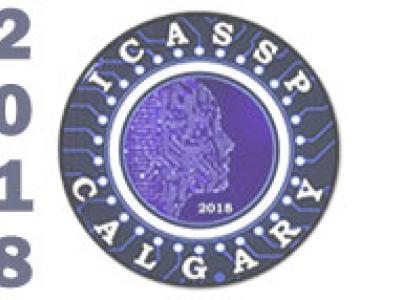
- Read more about Recognition of Faces and Facial Attributes using Accumulative Local Sparse Representations
- Log in to post comments
This paper addresses the problem of automated recognition of faces and facial attributes by proposing a new general approach called Accumulative Local Sparse Representation (ALSR). In the learning stage, we build a general dictionary of patches that are extracted from face images in a dense manner on a grid. In the testing stage, patches of the query image are sparsely represented using a \em local dictionary. This dictionary contains similar atoms of the general dictionary that are spatially in the same neighborhood.
- Categories:
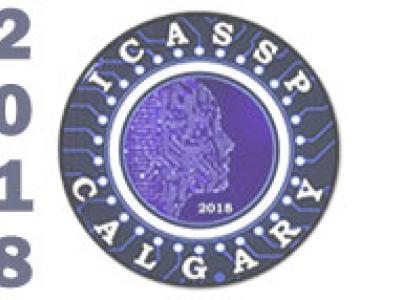
- Read more about A Greedy Pursuit Algorithm For Separating Signals From Nonlinear Compressive Observations
- Log in to post comments
In this paper we study the unmixing problem which aims
to separate a set of structured signals from their superposition.
In this paper, we consider the scenario in which
the mixture is observed via nonlinear compressive measurements.
We present a fast, robust, greedy algorithm called
Unmixing Matching Pursuit (UnmixMP) to solve this problem.
We prove rigorously that the algorithm can recover the
constituents from their noisy nonlinear compressive measurements
with arbitrarily small error. We compare our algorithm
- Categories:
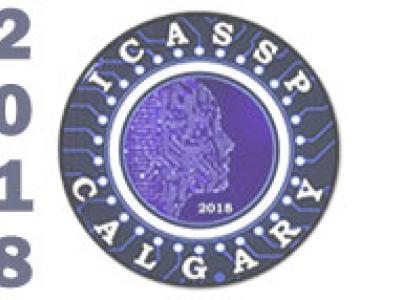
- Read more about Generative Adversarial Source Separation
- Log in to post comments
- Categories:
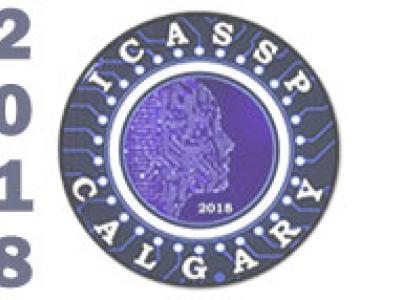
- Read more about REALIZING DIRECTIONAL SOUND SOURCE IN FDTD METHOD BY ESTIMATING INITIAL VALUE
- Log in to post comments
Wave-based acoustic simulation methods are studied actively for predicting acoustical phenomena. Finite-difference timedomain (FDTD) method is one of the most popular methods owing to its straightforwardness of calculating an impulse response. In an FDTD simulation, an omnidirectional sound source is usually adopted, which is not realistic because the real sound sources often have specific directivities. However, there is very little research on imposing a directional sound source into FDTD methods.
- Categories:
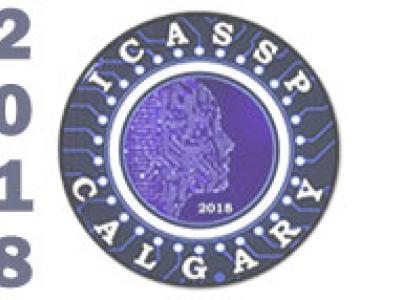
- Read more about Maximal Figure-of-Merit Embedding for Multi-label Audio Classification
- Log in to post comments
- Categories:
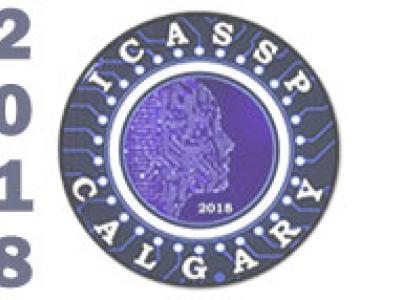
- Read more about Vocal melody extraction using patch-based CNN
- Log in to post comments
A patch-based convolutional neural network (CNN) model presented in this paper for vocal melody extraction in polyphonic music is inspired from object detection in image processing. The input of the model is a novel time-frequency representation which enhances the pitch contours and suppresses the harmonic components of a signal. This succinct data representation and the patch-based CNN model enable an efficient training process with limited labeled data. Experiments on various datasets show excellent speed and competitive accuracy comparing to other deep learning approaches.
- Categories:
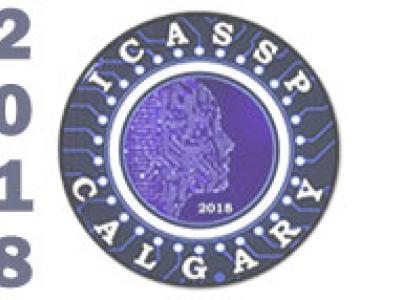
- Read more about On the use of WaveNet as a Statistical Vocoder
- Log in to post comments
- Categories: