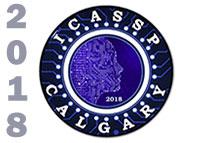
ICASSP is the world’s largest and most comprehensive technical conference focused on signal processing and its applications. The 2019 conference will feature world-class presentations by internationally renowned speakers, cutting-edge session topics and provide a fantastic opportunity to network with like-minded professionals from around the world. Visit website.
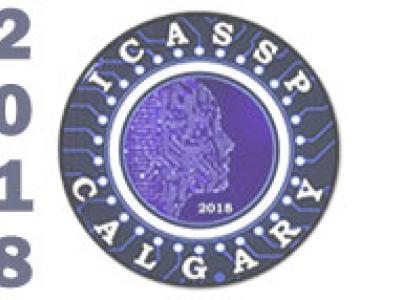
- Read more about Sound field reproduction with exterior cancellation using analytical weighting of harmonic coefficients
- Log in to post comments
A method for sound field reproduction with the suppression of exterior radiation is proposed, which makes it possible to synthesize a desired sound field in a reverberant environment without prior knowledge of the transfer functions of the multiple loudspeakers. The objective function used to achieve this is formulated as the weighted sum of the interior reproduction error and exterior radiation power. The optimal driving signals are derived by harmonic expansion of both the interior and exterior sound fields.
20180413.pdf

- Categories:
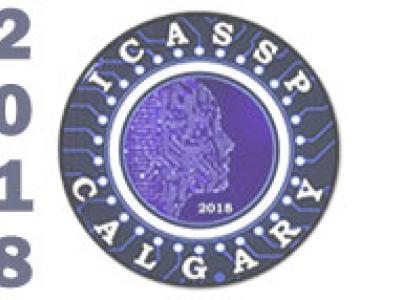
- Read more about POLYPHONIC MUSIC SEQUENCE TRANSDUCTION WITH METER-CONSTRAINED LSTM NETWORKS
- Log in to post comments
Automatic transcription of polyphonic music remains a challenging task in the field of Music Information Retrieval. In this paper, we propose a new method to post-process the output of a multi-pitch detection model using recurrent neural networks. In particular, we compare the use of a fixed sample rate against a meter-constrained time step on a piano performance audio dataset. The metric ground truth is estimated using automatic symbolic alignment, which we make available for further study.
- Categories:
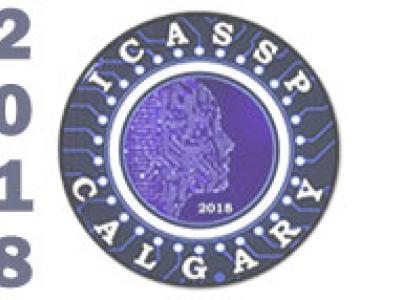
- Read more about MOTOR IMAGERY FOR EEG BIOMETRICS USING CONVOLUTIONAL NEURAL NETWORK
- Log in to post comments
This paper deals with electroencephalography (EEG)-based biometric identification, using a motor imagery task, specifically
performing imaginary arms and legs movements. Deep learning methods such as convolutional neural network (CNN) is used for automatic discriminative feature extraction and person identification. An extensive set of experimental tests, performed on a large database comprising EEG data collected from 40 subjects over two different sessions taken at a week distance, shows the existence of repeatable discriminative characteristics in individuals’ brain signals.
- Categories:
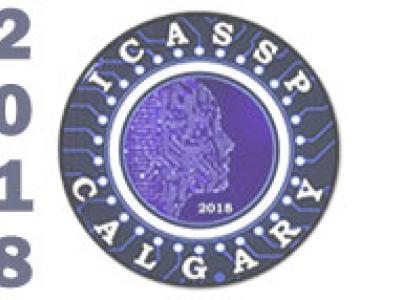
- Read more about AUDIO-VISUAL PERSON RECOGNITION IN MULTIMEDIA DATA FROM THE IARPA JANUS PROGRAM
- Log in to post comments
- Categories:
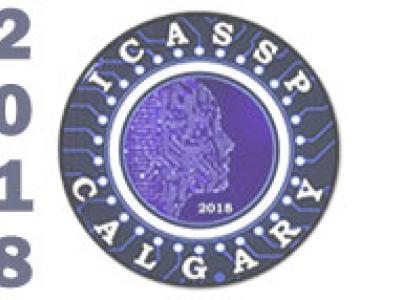
- Read more about 3-D CNN Models FOR FAR-FIELD MULTI-CHANNEL Speech Recognition
- Log in to post comments
- Categories:
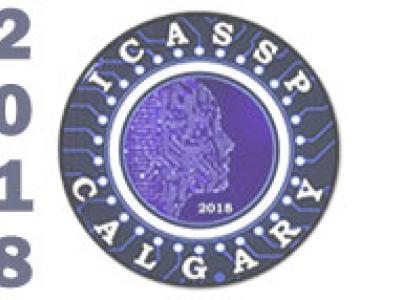
- Read more about Bayesian inference for multi-line spectra in linear sensor array
- Log in to post comments
- Categories:
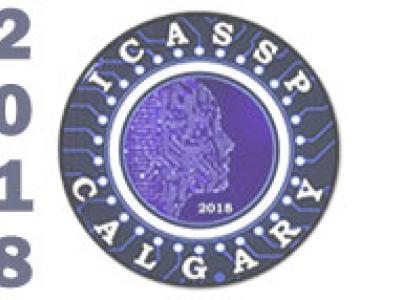
- Read more about Spectral feature mapping with mimic loss for robust speech recognition
- Log in to post comments
For the task of speech enhancement, local learning objectives are agnostic to phonetic structures helpful for speech recognition. We propose to add a global criterion to ensure de-noised speech is useful for downstream tasks like ASR. We first train a spectral classifier on clean speech to predict senone labels. Then, the spectral classifier is joined with our speech enhancer as a noisy speech recognizer. This model is taught to imitate the output of the spectral classifier alone on clean speech.
- Categories:
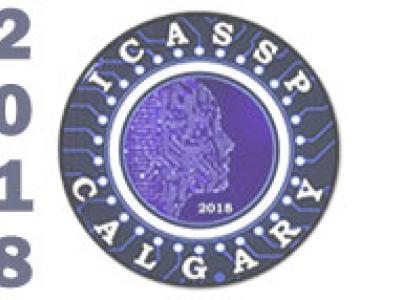
- Read more about RATE-OPTIMAL META LEARNING OF CLASSIFICATION ERROR
- Log in to post comments
Meta learning of optimal classifier error rates allows an experimenter to empirically estimate the intrinsic ability of any estimator to discriminate between two populations, circumventing the difficult problem of estimating the optimal Bayes classifier. To this end we propose a weighted nearest neighbor (WNN) graph estimator for a tight bound on the Bayes classification error; the Henze-Penrose (HP) divergence. Similar to recently proposed HP estimators [berisha2016], the proposed estimator is non-parametric and does not require density estimation.
- Categories:
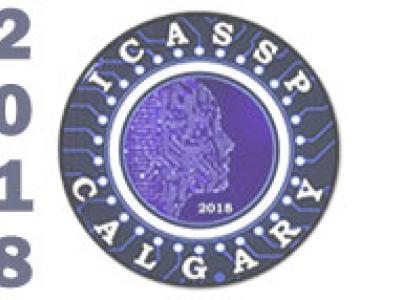
- Read more about Rate-optimal Meta Learning of Classification Error
- Log in to post comments
Meta learning of optimal classifier error rates allows an experimenter to empirically estimate the intrinsic ability of any estimator to discriminate between two populations, circumventing the difficult problem of estimating the optimal Bayes classifier. To this end we propose a weighted nearest neighbor (WNN) graph estimator for a tight bound on the Bayes classification error; the Henze-Penrose (HP) divergence. Similar to recently proposed HP estimators [berisha2016], the proposed estimator is non-parametric and does not require density estimation.
- Categories: