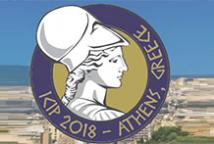
The International Conference on Image Processing (ICIP), sponsored by the IEEE Signal Processing Society, is the premier forum for the presentation of technological advances and research results in the fields of theoretical, experimental, and applied image and video processing. ICIP has been held annually since 1994, brings together leading engineers and scientists in image and video processing from around the world. Visit website.
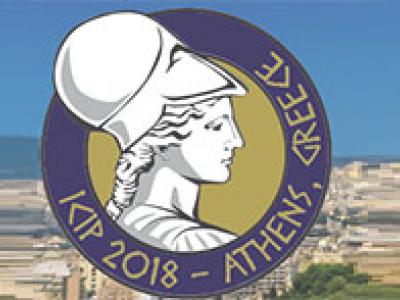
Detection of cell nuclei in microscopic images is a challenging research topic, because of limitations in cellular image quality and diversity of nuclear morphology, i.e. varying nuclei shapes, sizes, and overlaps between multiple cell nuclei. This has been a topic of enduring interest with promising recent success shown by deep learning methods. These methods train for example convolutional neural networks (CNNs) with a training set of input images and known, labeled nuclei locations. Many of these methods are supplemented by spatial or morphological processing.
- Categories:
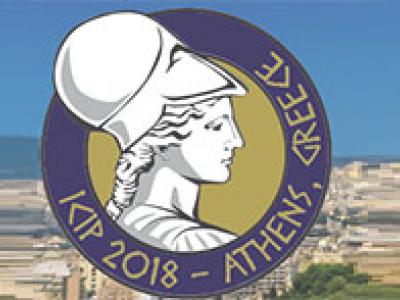
- Read more about RECOVERING TEXTURE OF DENOISED IMAGE VIA ITS STATISTICAL ANALYSIS
- Log in to post comments
ICIPposter.pdf

- Categories:
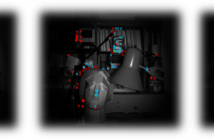
- Read more about MULTIPLE LAYERS OF CONTRASTED IMAGES FOR ROBUST FEATURE-BASED VISUAL TRACKING
- Log in to post comments
Feature-based SLAM (Simultaneous Localization and Mapping) techniques rely on low-level contrast information extracted from images to detect and track keypoints. This process is known to be sensitive to changes in illumination of the environment that can lead to tracking failures. This paper proposes a multi-layered image representation (MLI) that computes and stores different contrast-enhanced versions of an original image. Keypoint detection is performed on each layer, yielding better robustness to light changes.
- Categories:
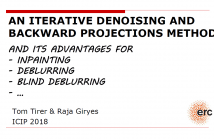
Inverse problems appear in many applications, such as image deblurring and inpainting. The common approach to address them is to design a specific algorithm for each problem. The Plug-and-Play (P&P) framework, which has been recently introduced, allows solving general inverse problems by leveraging the impressive capabilities of existing denoising algorithms. While this fresh strategy has found many applications, a burdensome parameter tuning is often required in order to obtain high-quality results.
- Categories:
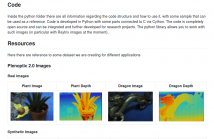
- Read more about Plenoptic Toolbox 2.0 - Benchmarking of Depth Estimation Methods for MLA-Based Focused Plenoptic Cameras
- Log in to post comments
MLA-based focused plenoptic cameras, also called type 2.0 cameras, have advantages over type 1.0 plenoptic cameras, because of their better inherent spatial image resolution and their compromise between depth of focus and angular resolution. However, they are more difficult to process since they require a depth estimation first to compute the all-in-focus image from the raw MLA image data. Current toolboxes for plenoptic cameras only support the type 1.0 cameras (like Lytro) and cannot handle type 2.0 cameras (like Raytrix).
- Categories:
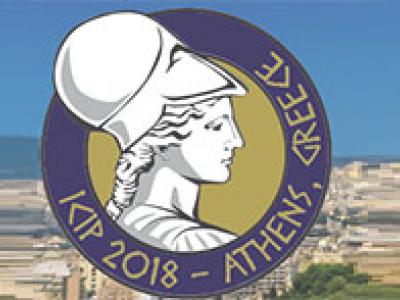
- Read more about DEEP MR BRAIN IMAGE SUPER-RESOLUTION USING STRUCTURAL PRIORS
- Log in to post comments
- Categories:
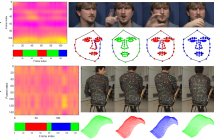
- Read more about DEFORMABLE MOTION 3D RECONSTRUCTION BY UNION OF REGULARIZED SUBSPACES
- Log in to post comments
This paper presents an approach to jointly retrieve camera pose, time-varying 3D shape, and automatic clustering based on motion primitives, from incomplete 2D trajectories in a monocular video. We introduce the concept of order-varying temporal regularization in order to exploit video data, that can be indistinctly applied to the 3D shape evolution as well as to the similarities between images. This results in a union of regularized subspaces which effectively encodes the 3D shape deformation.
- Categories:
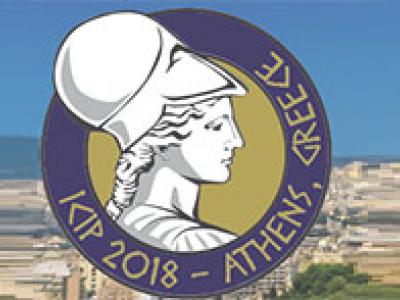
Image deblurring is one of the standard problems in image processing.
Recently, this area of research is dominated by blind deblurring, where neither the sharp image nor the blur are known.
The majority of works, however, target scenarios where the captured scene is static and the blur is caused by camera motion, i.e. the whole image is blurred.
In this work we address a similar yet different scenario: an object moves in front of a static background.
Such object is blurred due to motion while the background is sharp and partially occluded by the object.
poster.pdf

- Categories:
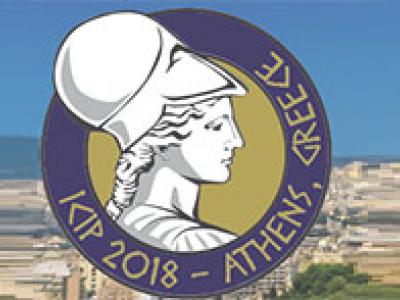
- Read more about Anisotropic Partial Differential Equation based Video Saliency Detection
- Log in to post comments
In this paper, we propose a novel video saliency detection method using the Partial Differential Equations (PDEs). We first form a static adaptive anisotropic PDE model from the unpredicted frames of the video using a detection map and a saliency seeds set of most attractive image elements. At the same time, we also extract motion features from the predicted frames of the video to generate motion saliency map. Then, we combine these two maps to obtain the final saliency map (video).
Poster.pdf

- Categories:
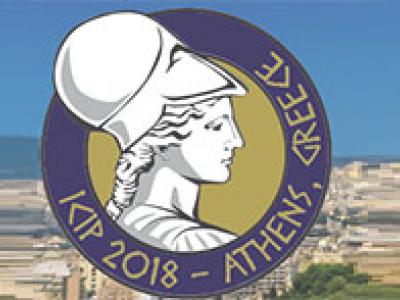
- Read more about AN ADVANCED VISIBILITY RESTORATION TECHNIQUE FOR UNDERWATER IMAGES
- Log in to post comments
- Categories: