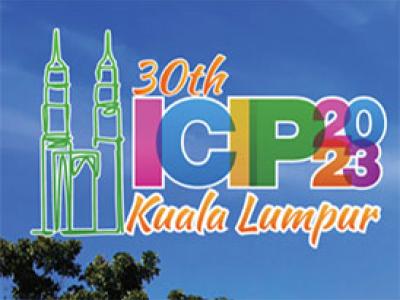
- Read more about Simple Self-Distillation Learning for Noisy Image Classification
- Log in to post comments
- Categories:
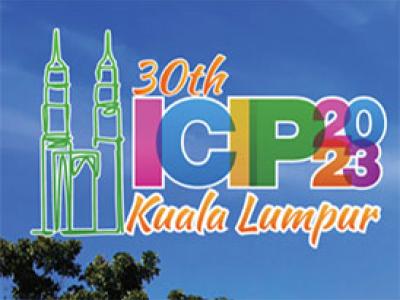
- Read more about Simple Self-Distillation Learning for Noisy Image Classification
- Log in to post comments
- Categories:
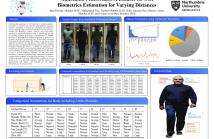
- Read more about Annotated Pedestrians: A Dataset for Soft Biometrics Estimation for Varying Distances
- Log in to post comments
Following the significance of soft biometrics to facilitate seamless recognition or retrieval, the need for multi-modality annotated datasets is increasing - to evaluate any standalone soft biometrics system. Although, large-size datasets like PETA were annotated to evaluate soft biometrics systems, however, they were mainly annotated for global soft biometrics such as gender and age and for clothing modality.
- Categories:
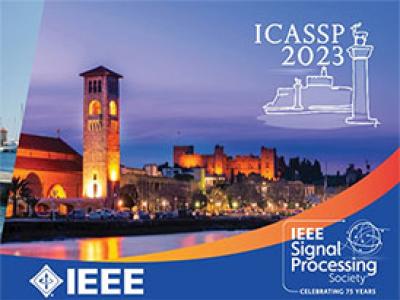
- Read more about Multi-dimensional Signal Recovery Using Low-rank Deconvolution
- Log in to post comments
In this work we present Low-rank Deconvolution, a powerful framework for low-level feature-map learning for efficient signal representation with application to signal recovery. Its formulation in multi-linear algebra inherits properties from convolutional sparse coding and low-rank approximation methods as in this setting signals are decomposed in a set of filters convolved with a set of low-rank tensors. We show its advantages by learning compressed video representations and solving image in-painting problems.
- Categories:
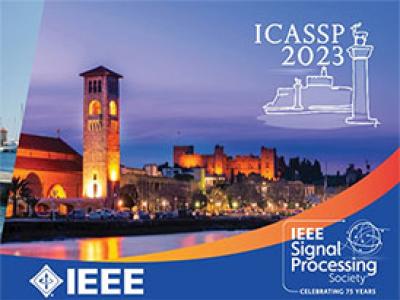
- Read more about MMCosine: Multi-Modal Cosine Loss Towards Balanced Audio-Visual Fine-Grained Learning
- Log in to post comments
Audio-visual learning helps to comprehensively understand the world by fusing practical information from multiple modalities. However, recent studies show that the imbalanced optimization of uni-modal encoders in a joint-learning model is a bottleneck to enhancing the model`s performance. We further find that the up-to-date imbalance-mitigating methods fail on some audio-visual fine-grained tasks, which have a higher demand for distinguishable feature distribution.
- Categories:
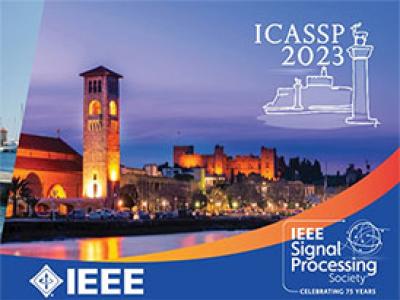
- Read more about Provably Convergent Plug & Play Linearized ADMM, applied to Deblurring Spatially Varying Kernels
- Log in to post comments
Plug & Play methods combine proximal algorithms with denoiser priors to solve inverse problems. These methods rely on the computability of the proximal operator of the data fidelity term. In this paper, we propose a Plug & Play framework based on linearized ADMM that allows us to bypass the computation of intractable proximal operators. We demonstrate the convergence of the algorithm and provide results on restoration tasks such as super-resolution and deblurring with non-uniform blur.
- Categories:
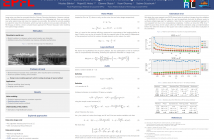
- Read more about PoGaIN: Poisson-Gaussian Image Noise Modeling from Paired Samples
- Log in to post comments
Image noise can often be accurately fitted to a Poisson-Gaussian distribution. However, estimating the distribution parameters from a noisy image only is a challenging task. Here, we study the case when paired noisy and noise-free samples are accessible. No method is currently available to exploit the noise-free information, which may help to achieve more accurate estimations. To fill this gap, we derive a novel, cumulant-based, approach for Poisson-Gaussian noise modeling from paired image samples.
- Categories:
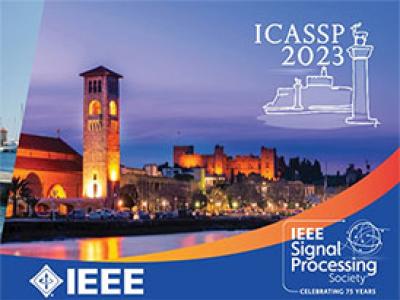
- Read more about Efficient Feature Extraction for Non-Maximum Suppression in Visual Person Detection
- Log in to post comments
poster.pdf

- Categories:
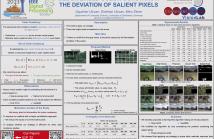
- Read more about Block-Based Color Constancy: The Deviation of Salient Pixels
- Log in to post comments
We recently proposed a color constancy method based on the observations that the human visual system might be "discounting the illuminant" by using space-average color and the highest luminance patches. Based on these observations, our algorithm relies on two assumptions: (i) there are several bright pixels in the scene, and (ii) the world is gray, on average. The main idea of the algorithm is to estimate the illuminant by finding the deviation of the brightest pixels from the gray value. During experiments, we observed that some pixels decrease the performance of the method.
- Categories: