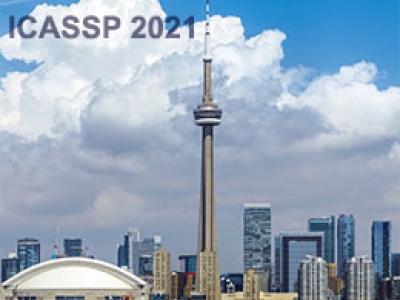
- Read more about Hierarchical Attention Fusion for Geo-Localization
- Log in to post comments
slides.pdf

poster.pdf

- Categories:
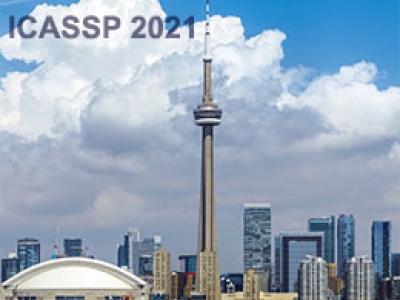
- Read more about MULTI-DIRECTIONAL CONVOLUTION NETWORKS WITH SPATIAL-TEMPORAL FEATURE PYRAMID MODULE FOR ACTION RECOGNITION
- Log in to post comments
Recent attempts show that factorizing 3D convolutional filters into separate spatial and temporal components brings impressive improvement in action recognition. However, traditional temporal convolution operating along the temporal dimension will aggregate unrelated features, since the feature maps of fast-moving objects have shifted spatial positions. In this paper, we propose a novel and effective Multi-Directional convolution (MDConv), which extracts features along different spatial-temporal orientations.
poster-4.pdf

- Categories:
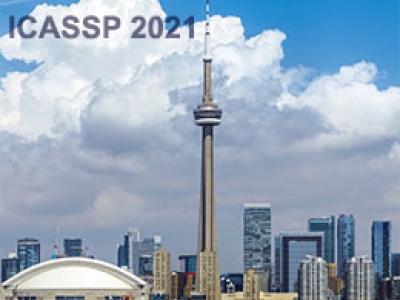
- Read more about Attention-Embedded Decomposed Network with Unpaired CT Images Prior for Metal Artifact Reduction
- Log in to post comments
Recently, unsupervised learning is proposed to avoid the performance degrading caused by synthesized paired computed tomography (CT) images. However, existing unsupervised methods for metal artifact reduction (MAR) only use features in image space, which is not enough to restore regions heavily corrupted by metal artifacts. Besides, they lack the distinction and selection for effective features. To address these issues, we propose an attention-embedded decomposed network to reduce metal artifacts in both image space and sinogram space with unpaired images.
poster2.pdf

- Categories:
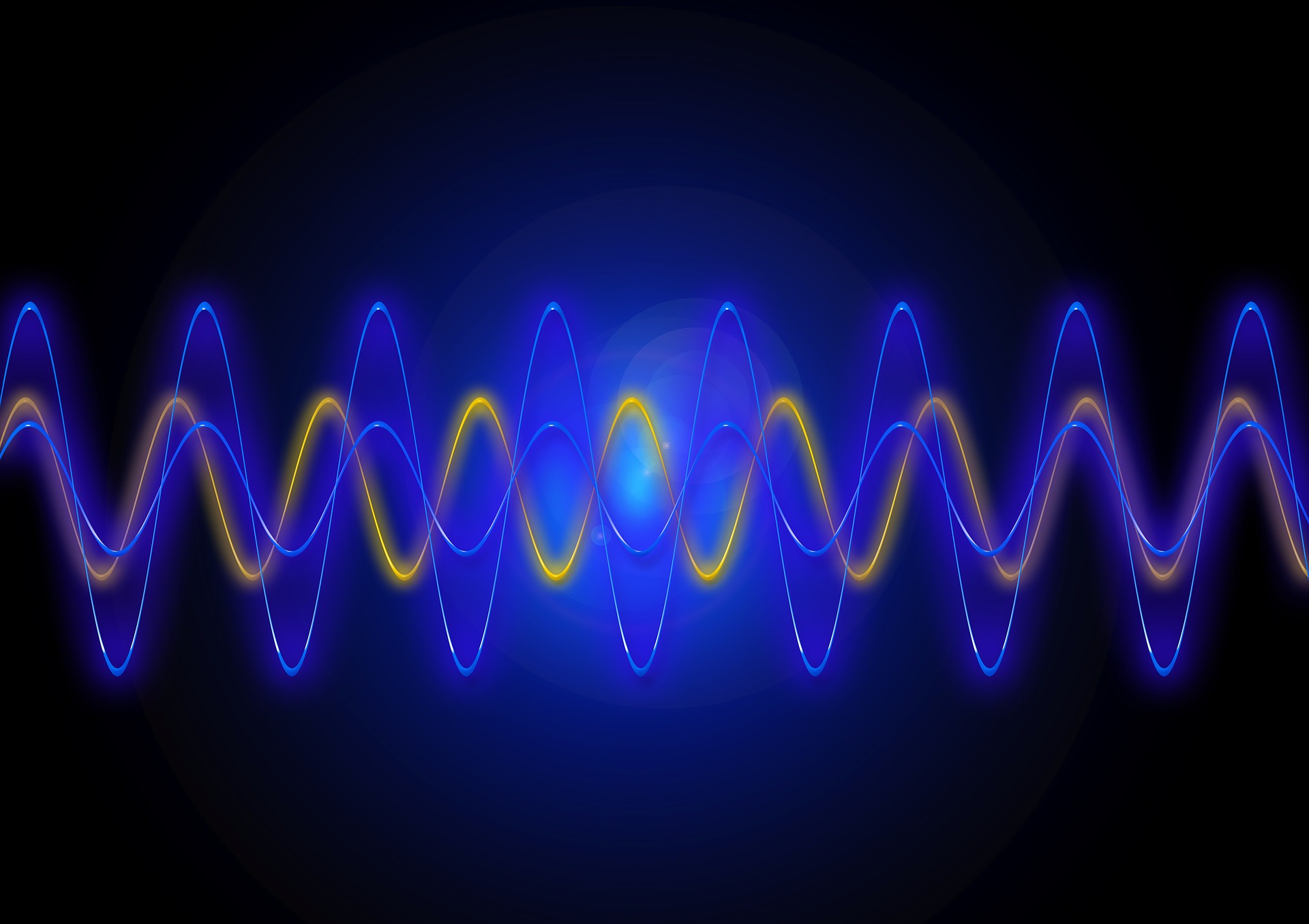
- Read more about ROBUST BINARY LOSS FOR MULTI-CATEGORY CLASSIFICATION WITH LABEL NOISE
- Log in to post comments
- Categories:
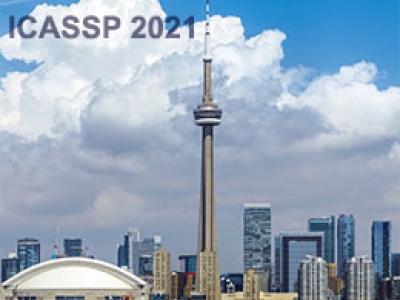
Unmanned aerial vehicles (UAV) often rely on GPS for navigation. GPS signals, however, are very low in power and easily jammed or otherwise disrupted. This paper presents a method for determining the navigation errors present at the beginning of a GPS-denied period utilizing data from a synthetic aperture radar (SAR) system. This is accomplished by comparing an online-generated SAR image with a reference image obtained a priori.
- Categories:
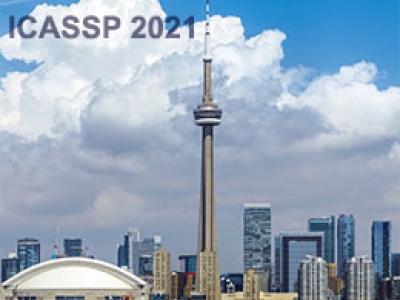
Unmanned aerial vehicles (UAV) often rely on GPS for navigation. GPS signals, however, are very low in power and easily jammed or otherwise disrupted. This paper presents a method for determining the navigation errors present at the beginning of a GPS-denied period utilizing data from a synthetic aperture radar (SAR) system. This is accomplished by comparing an online-generated SAR image with a reference image obtained a priori.
- Categories:
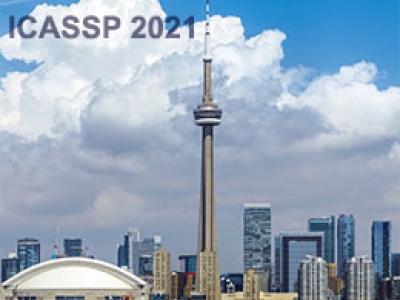
Few-shot image classification aims to classify unseen classes with limited labelled samples. Recent works benefit from the meta-learning process with episodic tasks and can fast adapt to class from training to testing. Due to the limited number of samples for each task, the initial embedding network for meta-learning becomes an essential component and can largely affect the performance in practice. To this end, most of the existing methods highly rely on the efficient embedding network.
- Categories:
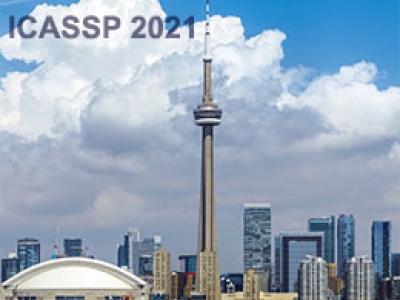
- Read more about OAS-Net: Occlusion Aware Sampling Network for Accurate Optical Flow
- Log in to post comments
OASNet_poster.pdf

- Categories:
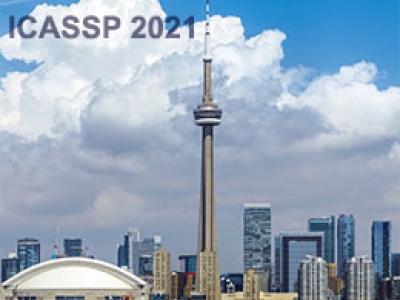
- Read more about Suremap: Predicting Uncertainty in Cnn-Based Image Reconstructions Using Stein’s Unbiased Risk Estimate
- Log in to post comments
Convolutional neural networks (CNN) have emerged as a powerful tool for solving computational imaging reconstruction problems. However, CNNs are generally difficult-to-understand black-boxes. Accordingly, it is challenging to know when they will work and, more importantly, when they will fail.
- Categories: