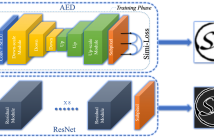
- Read more about PRED: A PARALLEL NETWORK FOR HANDLING MULTIPLE DEGRADATIONS VIA SINGLE MODEL IN SINGLE IMAGE SUPER-RESOLUTION
- Log in to post comments
Existing SISR (single image super-resolution) methods mostly assume that a low-resolution (LR) image is bicubicly downsampled from its high-resolution (HR) counterpart, which inevitably give rise to poor performance when the degradation is out of assumption. To address this issue, we propose a framework PRED (parallel residual and encoder-decoder network) with an innovative training strategy to enhance the robustness to multiple degradations. Consequently, the network can handle spatially variant degradations, which significantly improves the practicability of the proposed method.
poster.pptx

PRED_paper.pdf

- Categories:
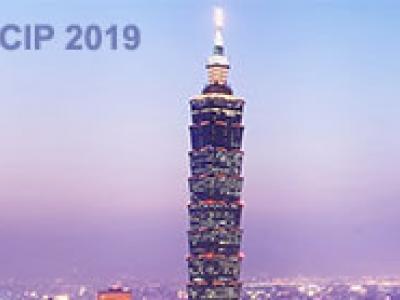
- Read more about FEATURE EXTRACTION AND TRACKING OF CNN SEGMENTATIONS FOR IMPROVED ROAD DETECTION FROM SATELLITE IMAGERY
- Log in to post comments
Road detection in high-resolution satellite images is an important and popular research topic in the field of image processing. In this paper, we propose a novel road extraction and tracking method based on road segmentation results from a convolutional network, providing improved road detection. The proposed method incorporates our previously proposed connected-tube marked point process (MPP) model and a post-tracking algorithm. We present experimental results on the Massachusetts roads dataset to show the performance of our method on road detection in remotely-sensed images.
- Categories:
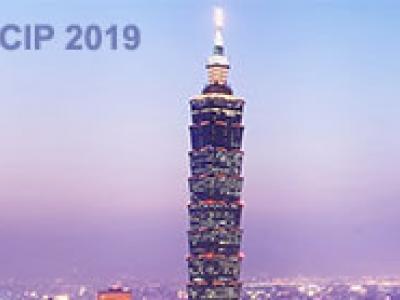
- Read more about FAST AND LIGHTWEIGHT IMAGE SUPER-RESOLUTION BASED ON DENSE RESIDUALS TWO-CHANNEL NETWORK
- Log in to post comments
The existing advanced super-resolution methods with deepening or widening network demand high computational resources and memory consumption. It is difficult to directly apply them in practice. Therefore, we propose a fast and lightweight two-channel end-to-end network with fewer parameters and low computational complexity in this paper. The shallow channel mainly restores the general outline of the image, while the deep channel mainly learns the high- frequency texture information. And the deep channel combines the dense block and residual connection.
- Categories:
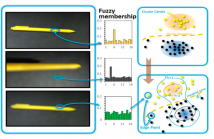
- Read more about BLIND IMAGE BLUR ASSESSMENT BASED ON MARKOV-CONSTRAINED FCM AND BLUR ENTROPY
- Log in to post comments
The image blur assessment is of various practical use such as feedback of microscope dynamic focusing and assessment of the quality of pictures in social media. However, the prob- lem of providing a fast and sensitive assessment toward im- age blur is not easy to deal with. In this paper, we provide a new effective way to evaluate the blur level of the image.
FCM poster.pdf

- Categories:
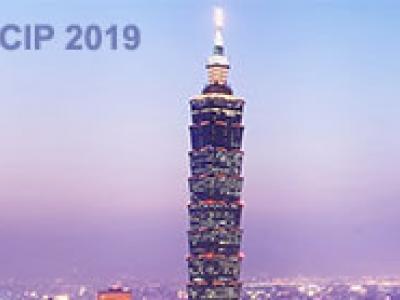
- Read more about DEPTH FROM SPECTRAL DEFOCUS BLUR
- Log in to post comments
This paper proposes a method for depth estimation from a single multispectral image by using a lens property known as chromatic aberration.
posterFin.pdf

- Categories:
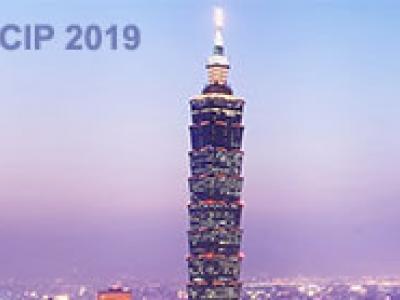
- Read more about AGE ESTIMATION USING TRAINABLE GABOR WAVELET LAYERS IN A CONVOLUTIONAL NEURAL NETWORK
- Log in to post comments
- Categories:
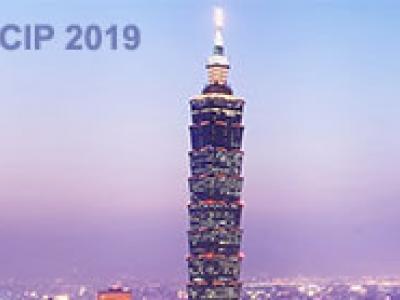
- Read more about DISCRIMINATIVE FEATURES FOR INCREMENTAL LEARNING CLASSIFIER
- 2 comments
- Log in to post comments
An important problem in artificial intelligence is to develop an ef-
ficient system that can adapt to new knowledge in an incremen-
tal manner without forgetting previously learned knowledge. Al-
though Convolutional Neural Networks (CNNs) are good at learn-
ing strong classifier and discriminative features, CNNs can not per-
form well in incremental classifier learning due to the catastrophic
forgetting problem in the retraining process. In this paper, we pro-
pose a novel yet extremely simple approach to enhance the discrim-
- Categories:
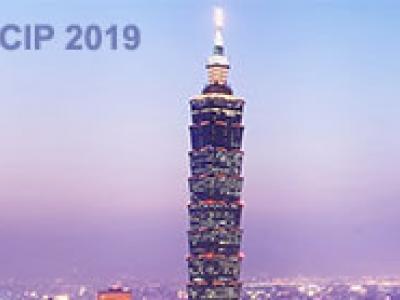
- Read more about GRAPH BASED NON-UNIFORM SAMPLING AND RECONSTRUCTION OF DEPTH MAPS
- Log in to post comments
High-quality depth sensing is highly demanded in intelligent computer vision, 3DTV, and many other related fields. However, prevalent time-of-fly (ToF) depth sensors are of low resolution as the number of pixel-level demodulators is limited. Moreover, the rectangular sampling does not consider the signal characteristics of depth maps. Being a departure of previous resolution enhancement on rectangular sampling, this paper investigates the non-uniform sampling of depth maps, and the high-resolution depth reconstruction from limited non-uniformly distributed samples.
ICIP.ppt

- Categories:
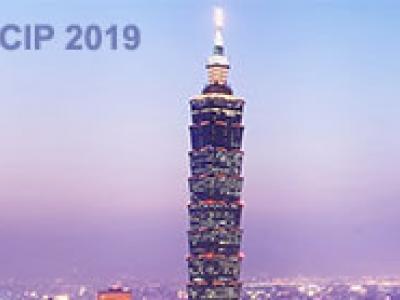
- Read more about Collaborative Method for Incremental Learning on Classification and Generation
- Log in to post comments
- Categories:
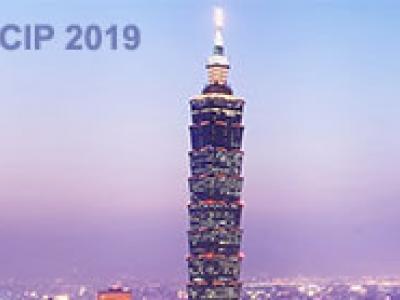
- Read more about Evolutionary Camera Pose Estimation of a Multi-Camera Setup for Computed Tomography
- Log in to post comments
- Categories: