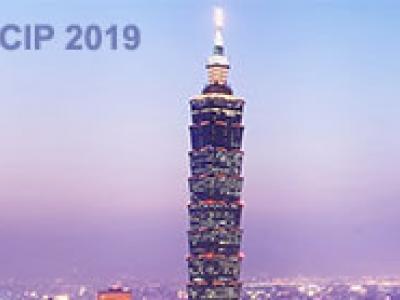
- Read more about REAL-TIME LIGHT FIELD DEPTH ESTIMATION VIA GPU-ACCELERATED MULTI-VIEW SEMI-GLOBAL MATCHING
- Log in to post comments
- Categories:
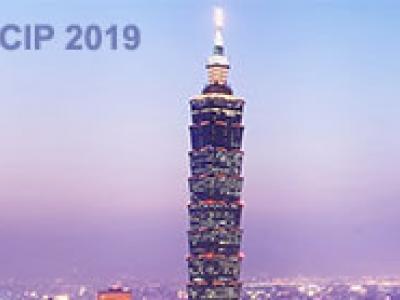
- Read more about CONTEXT-AWARE AUTOMATIC OCCLUSION REMOVAL
- Log in to post comments
Occlusion removal is an interesting application of image enhancement, for which, existing work suggests manually-annotated or domain-specific occlusion removal. No work tries to address automatic occlusion detection and removal as a context-aware generic problem. In this paper, we present a novel methodology to identify objects that do not relate to the image context as occlusions and remove them, reconstructing the space occupied coherently.
- Categories:
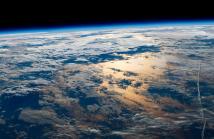
- Read more about CLOUDMASKGAN: A CONTENT-AWARE UNPAIRED IMAGE-TO-IMAGE TRANSLATION ALGORITHM FOR REMOTE SENSING IMAGERY
- Log in to post comments
Cloud segmentation is a vital task in applications that utilize satellite imagery. A common obstacle in using deep learning-based methods for this task is the insufficient number of images with their annotated ground truths. This work presents a content-aware unpaired image-to-image translation algorithm. It generates synthetic images with different land cover types from original images while preserving the locations and the intensity values of the cloud pixels. Therefore, no manual annotation of ground truth in these images is required.
- Categories:
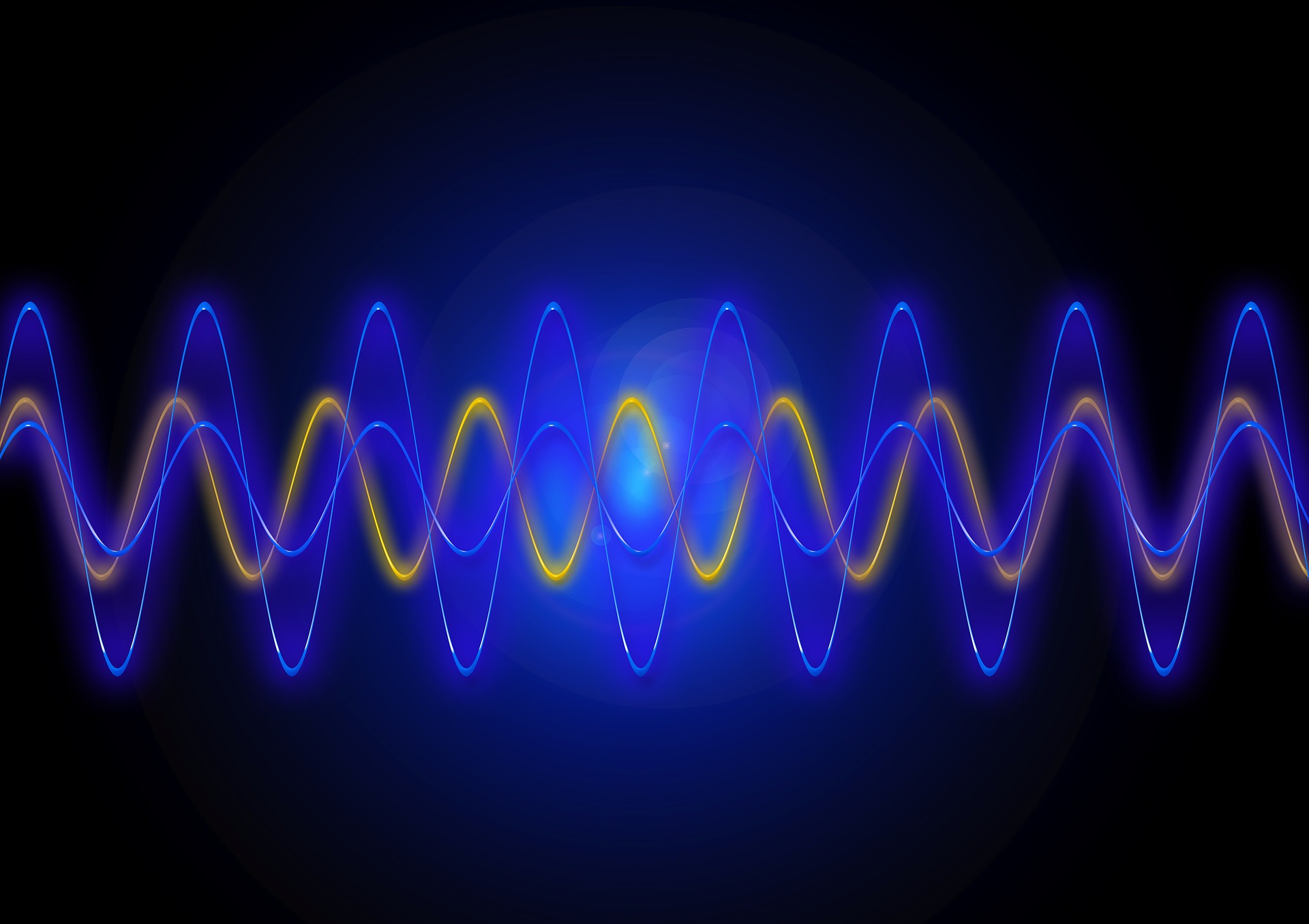
- Categories:
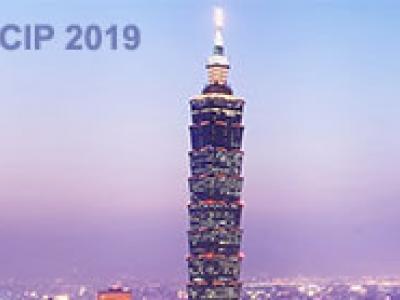
This paper presents a framework for accurately tracking objects of complex shapes with joint minimization of geometric and photometric parameters using a coarse 3D object model with the RGB-D cameras. Tracking with coarse 3D model is remarkably useful for industrial applications. A technique is proposed that uses a combination of point-to-plane distance minimization and photometric error minimization to track objects accurately. The concept of 'keyframes' are used in this system of object tracking for minimizing drift.
- Categories:
- Read more about A Metrological Measurement of Texture in Hyperspectral Images using Relocated Spectral Difference Occurrence Matrix
- Log in to post comments
A new hyperspectral texture descriptor, Relocated Spectral Difference Occurrence Matrix (rSDOM) is proposed. It assesses the distribution of spectral difference in a given neighborhood. For metrological purposes, rSDOM employs Kullback-Leibler pseudo-divergence (KLPD) for spectral difference calculation. It is generic and adapted for any spectral range and number of band. As validation, a texture classification scheme based on nearest neighbor classifier is applied on HyTexiLa dataset using rSDOM.
- Categories:
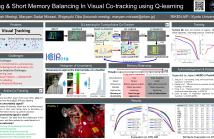
- Read more about Long & Short Memory Balancing In Visual Co-tracking using Q-learning
- Log in to post comments
Employing one or more additional classifiers to break the self-learning loop in tracing-by-detection has gained considerable attention. Most of such trackers merely utilize the redundancy to address the accumulating label error in the tracking loop, and suffer from high computational complexity as well as tracking challenges that may interrupt all classifiers (e.g. temporal occlusions). We propose the active co-tracking framework, in which the main classifier of the tracker labels samples of the video sequence, and only consults auxiliary classifier when it is uncertain.
- Categories:
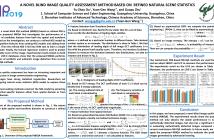
- Read more about A NOVEL BLIND IMAGE QUALITY ASSESSMENT METHOD BASED ON REFINED NATURAL SCENE STATISTICS
- Log in to post comments
Natural scene statistics (NSS) model has received considerable attention in the image quality assessment (IQA) community due to its high sensitivity to image distortion. However, most existing NSS-based IQA methods extract features either from spatial domain or from transform domain. There is little work to simultaneously consider the features from these two domains. In this paper, a novel blind IQA method (NBIQA) based on refined NSS is proposed. The proposed NBIQA first investigates the performance of a large number of candidate features from both the spatial and transform domains.
- Categories:
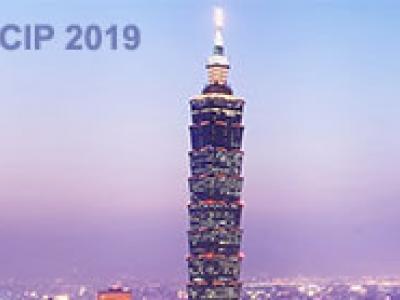
- Read more about Adaptive Hard Example Mining for Image Captioning
- Log in to post comments
- Categories:
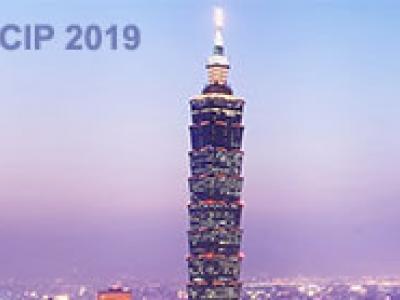
- Read more about AN END-TO-END MULTI-SCALE RESIDUAL RECONSTRUCTION NETWORK FOR IMAGE COMPRESSIVE SENSING
- Log in to post comments
Recently, deep-learning based reconstruction models have been proposed to improve recovery performance of compressive sensed image and overcome expensive time complexity drawbacks of iteration-based traditional algorithms. In this paper, we propose an end-to-end multi-scale residual convolutional neural network (CNN), dubbed MSRNet, to simulate image compressive sensing (CS) and inverse reconstruction process in real situation. In MSRNet, we apply three parallel channels with different convolution kernel sizes to exploit different-scale feature information.
- Categories: