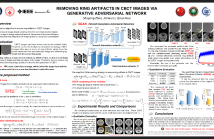
- Read more about REMOVING RING ARTIFACTS IN CBCT IMAGES VIA GENERATIVE ADVERSARIAL NETWORK
- Log in to post comments
Cone-beam computed tomography (CBCT) images often have some ring artifacts because of the inconsistent response of detector pixels. Removing ring artifacts in CBCT images without impairing the image quality is critical for the application of CBCT. In this paper, we explore this issue as an “adversarial problem” and propose a novel method to eliminate ring artifacts from CBCT images by using an imageto-image network based on Generative Adversarial Network (GAN).
- Categories:
- Read more about Computational Scratch Assay - A New Frontier for Image Analysis: Preliminary Study of Multi-Cellular Segmentation
- Log in to post comments
Quantitative scratch assay is significant in cell motility study for tissue repair, evolution of disease, drug treatment, and cancer metastasis. To overcome challenges in traditional manual operations in scratch assay, computational scratch assay is introduced, where image processing algorithms are exploited for cell motility quantification. In this new research realm, dedicated analysis tools are under-developed, which provides many opportunities for researchers expert on signal processing.
- Categories:
- Read more about SEGMENTATION OF CORONARY ARTERIES FROM X-RAY ANGIOGRAPHY SEQUENCES DURING CONTRAST FLUID PROPAGATION BY IMAGE REGISTRATION
- 1 comment
- Log in to post comments
Gaining information about heart and coronary arteries such as estimating the flow velocity and coronary flow reserve (CFR) by only using 2D + time X-ray angiography sequence is of great interest due to its availability. We propose to segment the coronary arteries from 2D+time X-ray angiography sequences during contrast fluid propagation, by using a multi-step method based on unsharp masking followed by an iterative process of segmenting and non-rigid registration, until the alignment from the registration process is satisfactory.
Abstract.pdf

- Categories:
- Read more about A Joint Multi-scale Convolutional Network for Fully Automatic Segmentation of the Left Ventricle
- Log in to post comments
Left ventricle (LV) segmentation is crucial for quantitative analysis of the cardiac contractile function. In this paper, we propose a joint multi-scale convolutional neural network to fully automatically segment the LV. Our method adopts two kinds of multi-scale features of cardiac magnetic resonance (CMR) images, including multi-scale features directly extracted from CMR images with different scales and multi-scale features constructed by intermediate layers of standard CNN architecture.
- Categories:
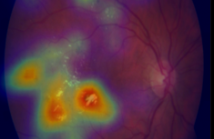
- Read more about WEAKLY-SUPERVISED LOCALIZATION OF DIABETIC RETINOPATHY LESIONS IN RETINAL FUNDUS IMAGES
- Log in to post comments
Convolutional neural networks (CNNs) show impressive performance for image classification and detection, extending heavily to the medical image domain. Nevertheless, medical experts are skeptical in these predictions as the nonlinear multilayer structure resulting in a classification outcome is not directly graspable. Recently, approaches have been shown which help the user to understand the discriminative regions within an image which are decisive for the CNN to conclude to a certain class.
- Categories:
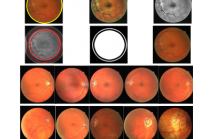
- Read more about CIRCLET BASED FRAMEWORK FOR OPTIC DISK DETECTION
- Log in to post comments
Optic Disc (OD) detection in retinal fundus images is a cru-cial stage for the automation of a screening system in diabetic ophthalmology. Most researches for automatic localization of OD benefit the regions of vessels. In this paper, we present a fast and novel method based on the Circlet Transform to detect OD in digital retinal fundus images that doesn’t utilize the location of the vessels. First, each R, G and B band is enhanced using CLAHE method. Then, the enhanced image in RGB color space is converted to L*a*b one.
- Categories:
- Read more about DETECTION OF MICROANEURYSM USING LOCAL RANK TRANSFORM IN COLOR FUNDUS IMAGES
- Log in to post comments
Accurate detection of microaneurysm (MA) plays a very important role in early diagnosis of diabetic retinopathy. This paper presents a novel method based on the variation of local intensity for microaneurysms detection in retinal images. In contribution, proposed method use local rank transform effectively
- Categories:
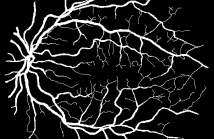
- Read more about Patch-based Fully Convolutional Neural Network With Skip Connections For Retinal Blood Vessel Segmentation
- Log in to post comments
Automated segmentation of retinal blood vessels plays an important role in the computer aided diagnosis of retinal diseases. The paper presents a new formulation of patch-based fully Convolutional Neural Networks (CNNs) that allows accurate segmentation of the retinal blood vessels. A major modification in this retinal blood vessel segmentation task is to improve and speed-up the patch-based fully CNN training by local entropy sampling and a skip CNN architecture with class-balancing loss.
icip2017.pdf

- Categories:
- Read more about Mass Segmentation in Mammograms: a Cross-Sensor comparison of deep and tailored features
- Log in to post comments
Through the years, several CAD systems have been developed to help radiologists in the hard task of detecting signs
of cancer in mammograms. In these CAD systems, mass segmentation plays a central role in the decision process. In the
literature, mass segmentation has been typically evaluated in a intra-sensor scenario, where the methodology is designed and
evaluated in similar data. However, in practice, acquisition systems and PACS from multiple vendors abound and current
- Categories:
Segmenting microvascular structures is an important requirement in understanding angioadaptation by which vascular networks remodel their morphological structures. Accurate segmentation for separating microvasculature structures is important in quantifying remodeling process. In this work, we utilize a deep convolutional neural network (CNN) framework for obtaining robust segmentations of microvasculature from epifluorescence microscopy imagery of mice dura mater.
- Categories: