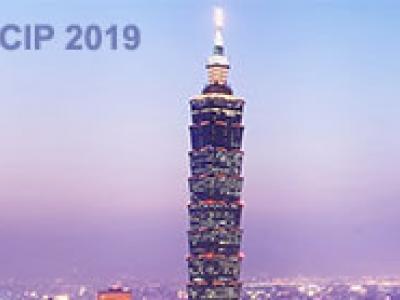
- Read more about BiRA-Net: Bilinear Attention Net for Diabetic Retinopathy Grading
- Log in to post comments
Diabetic retinopathy (DR) is a common retinal disease that leads to blindness. For diagnosis purposes, DR image grading aims to provide automatic DR grade classification, which is not addressed in conventional research methods of binary DR image classification. Small objects in the eye images, like lesions and microaneurysms, are essential to DR grading in medical imaging, but they could easily be influenced by other objects.
- Categories:
- Read more about DEEP U-NET REGRESSION AND HAND-CRAFTED FEATURE FUSION FOR ACCURATE BLOOD VESSEL SEGMENTATION
- Log in to post comments
Automated curvilinear image segmentation is a crucial step to characterize and quantify the morphology of blood vessels across scale. We propose a dual pipeline RF_OFB+U-NET that fuses U-Net deep learning features with a low level image feature filter bank using the random forests classifier for vessel segmentation. We modify the U-Net CNN architecture to provide a foreground vessel regression likelihood map that is used to segment both arteriole and venule blood vessels in mice dura mater tissues.
- Categories:
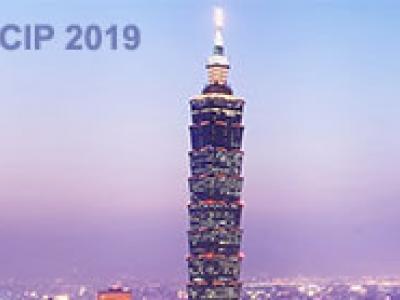
- Read more about A dual attention dilated residual network for liver lesion classification and localization on CT images
- Log in to post comments
Automatic liver lesion classification on computed
tomography images is of great importance to early cancer
diagnosis and remains a challenging task. State-of-the-art
liver lesion classification algorithms are currently based on
manually selected regions of interest (ROIs) or automatically
detected ROIs. However, liver lesions usually vary in size
and shape, which makes the ROI selection process laborintensive
and also poses an obstacle to automatic lesion
detection. In this paper, we propose a dual-attention dilated
- Categories:
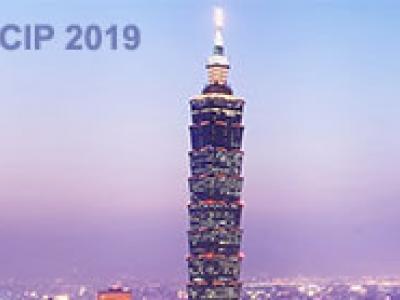
- Read more about A dual attention dilated residual network for liver lesion classification and localization on CT images
- Log in to post comments
Automatic liver lesion classification on computed
tomography images is of great importance to early cancer
diagnosis and remains a challenging task. State-of-the-art
liver lesion classification algorithms are currently based on
manually selected regions of interest (ROIs) or automatically
detected ROIs. However, liver lesions usually vary in size
and shape, which makes the ROI selection process laborintensive
and also poses an obstacle to automatic lesion
detection. In this paper, we propose a dual-attention dilated
- Categories:
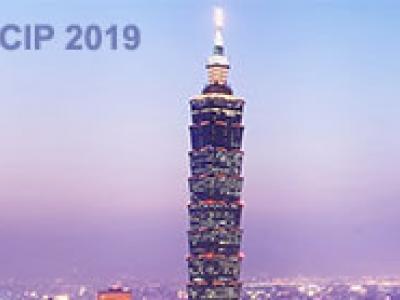
- Read more about A dual attention dilated residual network for liver lesion classification and localization on CT images
- Log in to post comments
Automatic liver lesion classification on computed
tomography images is of great importance to early cancer
diagnosis and remains a challenging task. State-of-the-art
liver lesion classification algorithms are currently based on
manually selected regions of interest (ROIs) or automatically
detected ROIs. However, liver lesions usually vary in size
and shape, which makes the ROI selection process laborintensive
and also poses an obstacle to automatic lesion
detection. In this paper, we propose a dual-attention dilated
- Categories:
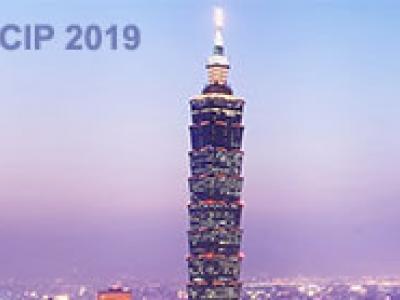
- Read more about ACCURATE SEGMENTATION OF SYNAPTIC CLEFT WITH CONTOUR GROWING CONCATENATED WITH A CONVNET
- Log in to post comments
- Categories:
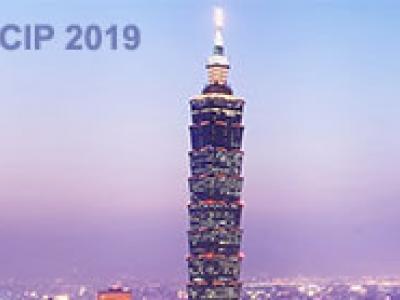
- Read more about Two-stage Unsupervised Learning Method for Affine and Deformable Registration
- Log in to post comments
Conventional medical image registration relies on time-consuming iterative optimization. We propose a two-stage unsupervised learning method for 3D medical image registration. In the first stage, we learn a global image-wise affine map by a deep network. In the second stage, we learn a local voxel-wise deformation vector field by an encoder-decoder architecture. The final registered image is acquired by applying the local deformation field to the moved image of the first stage.
- Categories:
- Read more about TENSOR-FACTORIZATION-BASED 3D SINGLE IMAGE SUPER-RESOLUTION WITH SEMI-BLIND POINT SPREAD FUNCTION ESTIMATION
- Log in to post comments
A volumetric non-blind single image super-resolution technique using tensor factorization has been recently introduced by our group. That method allowed a 2-order-of-magnitude faster high-resolution image reconstruction with equivalent image quality compared to state-of-the-art algorithms. In this work a joint alternating recovery of the high-resolution image and of the unknown point spread function parameters is proposed. The method is evaluated on dental computed tomography images.
- Categories:
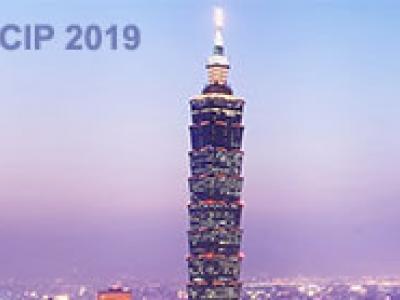
- Read more about Low Dose Abdominal CT Image Reconstruction: An Unsupervised Learning based Approach
- Log in to post comments
In medical practice, the X-ray Computed tomography-based scans expose a high radiation dose and lead to the risk of prostate or abdomen cancers. On the other hand, the low-dose CT scan can reduce radiation exposure to the patient. But the reduced radiation dose degrades image quality for human perception, and adversely affects the radiologist’s diagnosis and prognosis. In this paper, we introduce a GAN based auto-encoder network to de-noise the CT images.
- Categories:
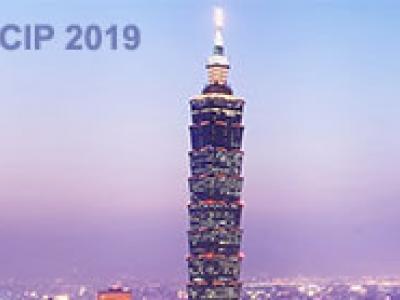
- Read more about COMPRESSED SENSING MRI WITH JOINT IMAGE-LEVEL AND PATCH-LEVEL PRIORS
- Log in to post comments
- Categories: