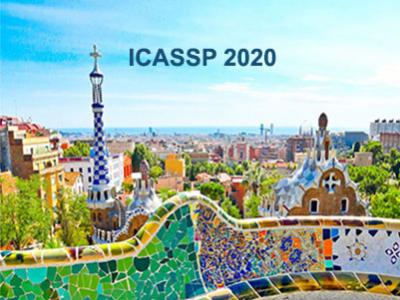
- Read more about A segmentation based deep learning framework for multimodal retinal image registration
- Log in to post comments
Multimodal image registration plays an important role in diagnosing and treating ophthalmologic diseases. In this paper, a deep learning framework for multimodal retinal image registration is proposed. The framework consists of a segmentation network, feature detection and description network, and an outlier rejection network, which focuses only on the globally coarse alignment step using the perspective transformation.
- Categories:
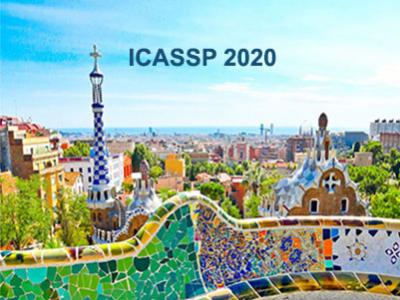
- Read more about A MULTI-SCALED RECEPTIVE FIELD LEARNING APPROACH FOR MEDICAL IMAGE SEGMENTATION
- 1 comment
- Log in to post comments
Biomedical image segmentation has been widely studied, and lots of methods have been proposed. Among these methods, attention U-Net has achieved a promising performance. However, it has drawbacks of extracting the multi-scaled receptive field features at the high-level feature maps, resulting in the degeneration when dealing with the lesions with apparent scale variations. To solve this problem, this paper integrates an atrous spatial pyramid pooling (ASPP) module in the contracting path of attention U-Net.
- Categories:
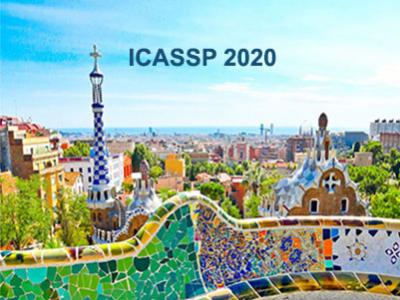
- Read more about Lightweight V-Net for Liver Segmentation
- Log in to post comments
The V-Net based 3D fully convolutional neural networks have been widely used in liver volumetric data segmentation. However, due to the large number of parameters of these networks, 3D FCNs suffer from high computational cost and GPU memory usage. To address these issues, we design a lightweight V-Net (LV-Net) for liver segmentation in this paper. The proposed network makes two contributions. The first is that we design an inverted residual bottleneck block (IRB block) and a 3D average pooling block and apply them to the proposed LV-Net.
- Categories:
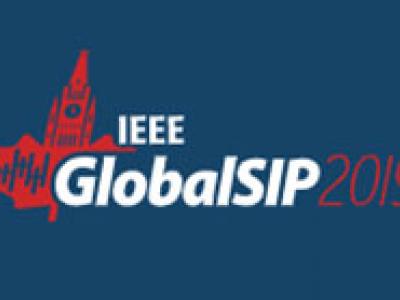
- Read more about Deep Learning Based Mass Detection in Mammograms
- Log in to post comments
Mammogram is the primary imaging technique for breast cancer screening, the leading type of cancer in women worldwide. While the clinical effectiveness of mammogram has been well demonstrated, the mammographic characteristics of breast masses are quite complex. As a result, radiologists certified for reading mammography are lacking, which limits the accessibility of mammography for more population. In this paper, we propose a Computer Aided Detection (CADe) method to automatically detect masses in mammography.
- Categories:
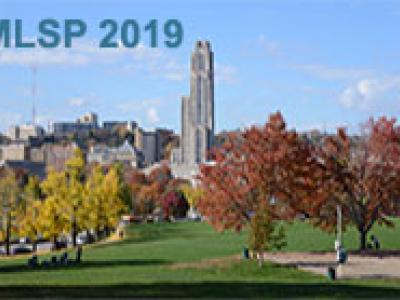
- Read more about A Multimodal Dense U-Net for Accelerating Multiple Sclerosis MRI
- Log in to post comments
The clinical analysis of magnetic resonance (MR) can be accelerated through the undersampling in the k-space (Fourier domain). Deep learning techniques have been recently received considerable interest for accelerating MR imaging (MRI). In this paper, a deep learning method for accelerating MRI is presented, which is able to reconstruct undersampled MR images obtained by reducing the k-space data in the direction of the phase encoding.
MLSP2019_poster_v4.pdf

- Categories:
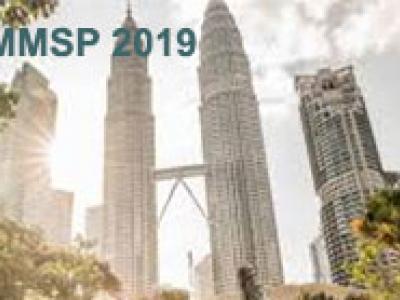
- Read more about Multi-Label Classification for Automatic Human Blastocyst Grading with Severely Imbalanced Data
- Log in to post comments
Quality scores assigned to blastocyst inner cell mass (ICM), trophectoderm (TE), and zona pellucida (ZP) are critical markers for predicting implantation potential of a human blastocyst in IVF treatment. Deep Convolutional Neural Networks (CNNs) have shown success in various image classification tasks, including classification of blastocysts into two quality categories. However, the problem of multi-label multi-class classification for blastocyst grading remains unsolved.
- Categories:
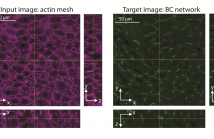
- Read more about PREDICTION OF MULTIPLE 3D TISSUE STRUCTURES BASED ON SINGLE-MARKER IMAGES USING CONVOLUTIONAL NEURAL NETWORKS
- Log in to post comments
A quantitative understanding of complex biological systems such as tissues requires reconstructing the structure of the different components of the system. Fluorescence microscopy provides the means to visualize simultaneously several tissue components. However, it can be time consuming and is limited by the number of fluorescent markers that can be used. In this study, we describe a toolbox of algorithms based on convolutional neural networks for the prediction of 3D tissue structures by learning features embedded within single-marker images.
- Categories: