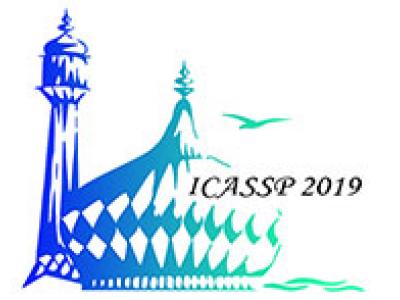
- Read more about Nuclei Segmentation in Histopathology Images
- Log in to post comments
Accurate and fast segmentation of nuclei in histopathological images plays a crucial role in cancer research for detection and grading, as well as personal treatment. Despite the important efforts, current algorithms are still suboptimal in terms of speed, adaptivity and generalizability. Popular Deep Convolutional Neural Networks (DCNNs) have recently been utilized for nuclei segmentation, outperforming \textit{traditional} approaches that exploit color and texture features in combination with shallow classifiers or segmentation algorithms.
- Categories:
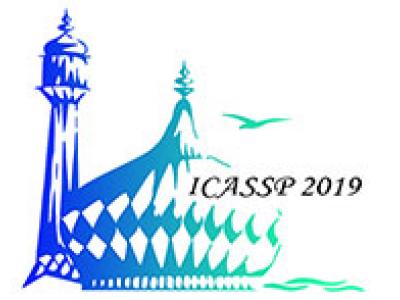
- Read more about A Pipeline for Lung Tumor Detection and Segmentation from CT Scans using Dilated Convolutional Neural Networks
- Log in to post comments
Lung cancer is the most prevalent cancer worldwide with about 230,000 new cases every year. Most cases go undiagnosed until it’s too late, especially in developing countries and remote areas. Early detection is key to beating cancer. Towards this end, the work presented here proposes an automated pipeline for lung tumor detection and segmentation from 3D lung CT scans from the NSCLC Radiomics Dataset. It also presents a new dilated hybrid-3D convolutional neural network architecture for tumor segmentation. First, a binary classifier chooses CT scan slices that may contain parts of a tumor.
- Categories:
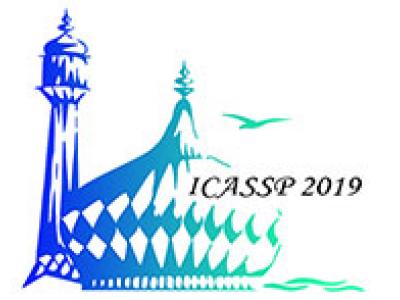
- Read more about Diving Deep onto Discriminative Ensemble of Histological Hashing & Class-Specific Manifold Learning for Multi-class Breast Carcinoma Taxonomy
- Log in to post comments
Histopathological images (HI) encrypt resolution dependent heterogeneous textures & diverse color distribution variability, manifesting in micro-structural surface tissue convolutions & inherently high coherency of cancerous cells posing significant challenges to breast cancer (BC) multi-classification.
- Categories:
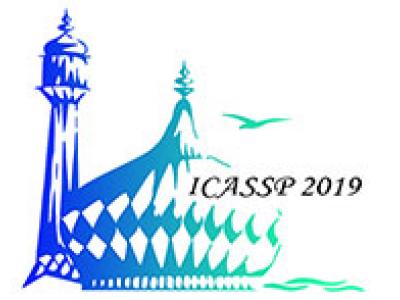
- Read more about UNMIXING DYNAMIC PET IMAGES: COMBINING SPATIAL HETEROGENEITY AND NON-GAUSSIAN NOISE
- Log in to post comments
- Categories:
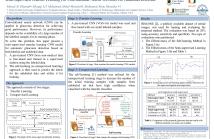
- Read more about Semi-Supervised Transfer Learning for Convolutional Neural Networks for Glaucoma Detection
- Log in to post comments
Convolutional neural network (CNN) can be applied in glaucoma detection for achieving good performance.
However, its performance depends on the availability of a large number of the labelled samples for its training phase.
To solve this problem, this paper present a semi-supervised transfer learning CNN model for automatic glaucoma detection based on both labeled and unlabeled data.
First, a pre-trained CNN from non-medical data is fine-tuned and trained in a supervised fashion using the labeled data.
- Categories:
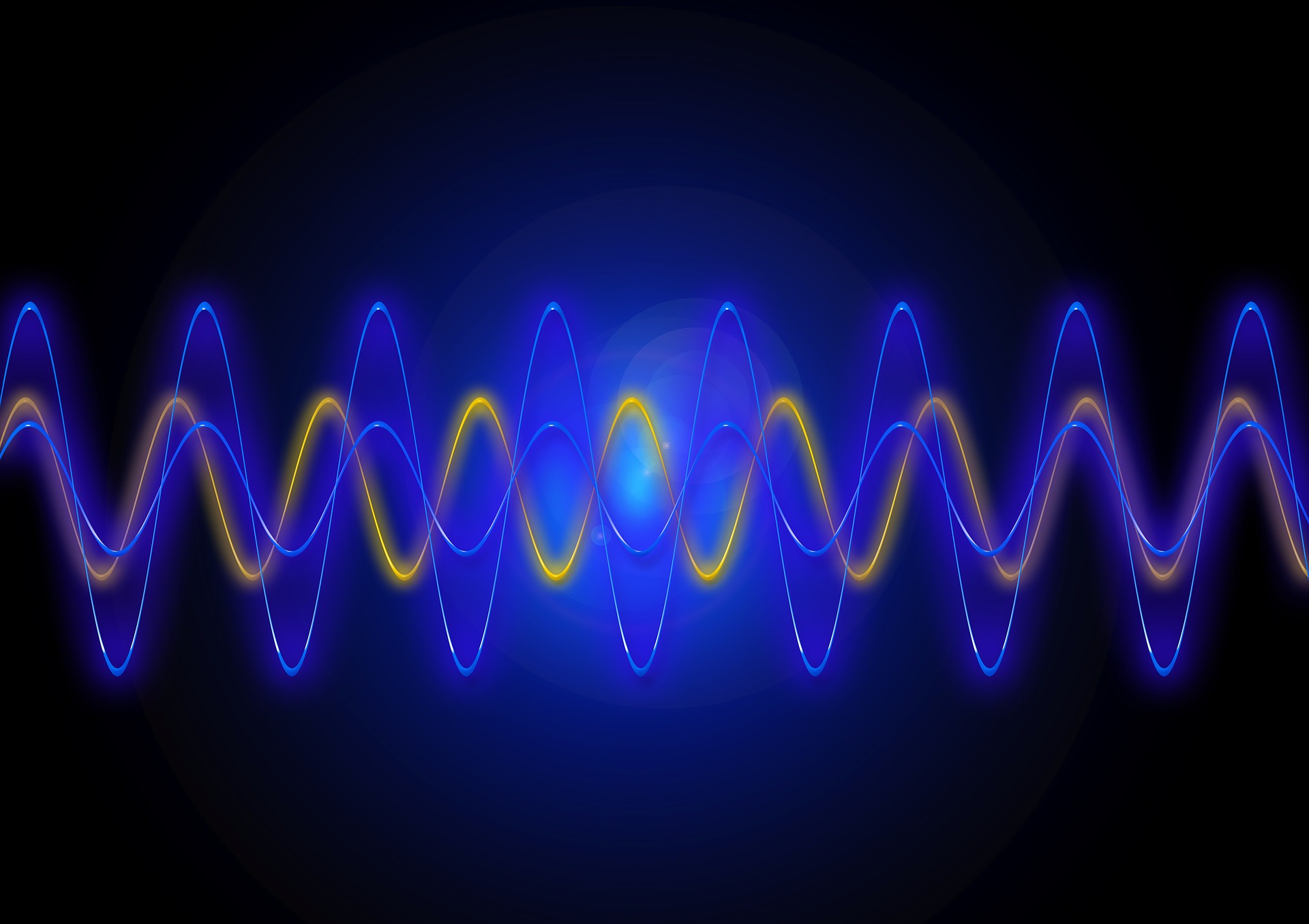
- Read more about AN EVENT-CONTRASTIVE CONNECTOME NETWORK FOR AUTOMATIC ASSESSMENTOF INDIVIDUAL FACE PROCESSING AND MEMORY ABILITY
- Log in to post comments
Human adapt their behaviors by continuously monitoring one another to function socially in our society. The ability to process face identity from memory is a crucial basic capability. In this work, we propose an event-contrastive connectome network (E-cCN) in representing brain’s functional connectivity with novel contrastive loss to handle layers of fMRI data variabilities exists under different controlled stimuli events to achieve improved automatic assessing of an individual’s face processing and memory ability.
- Categories:
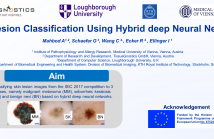
- Read more about SKIN LESION CLASSIFICATION USING HYBRID DEEP NEURAL NETWORKS
- Log in to post comments
Skin cancer is one of the major types of cancers with an increasing incidence over the past decades. Accurately diagnosing skin lesions to discriminate between benign and malignant skin lesions is crucial to ensure appropriate patient treatment. While there are many computerised methods for skin lesion classification, convolutional neural networks (CNNs) have been shown to be superior over classical methods.
- Categories:
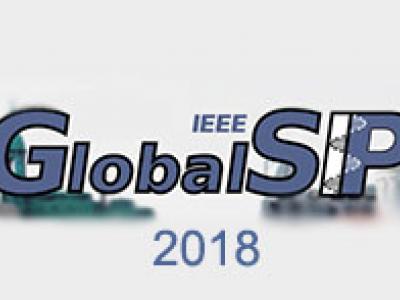
- Read more about POLARITY INVARIANT TRANSFORMATION FOR EEG MICROSTATES ANALYSIS
- Log in to post comments
Electroencephalography (EEG) has been widely used in human brain research. Several techniques in EEG relies on analyzing the topographical distribution of the data. One of the most common analysis is EEG microstate (EEG-ms). EEG-ms reflects the stable topographical representation of EEG signal lasting a few dozen milliseconds. EEG-ms were associated with resting state fMRI networks and were associated with mental processes and abnormalities.
- Categories:
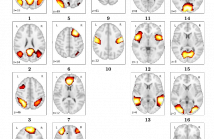
- Read more about HOW MANY FMRI SCANS ARE NECESSARY AND SUFFICIENT FOR RESTING BRAIN CONNECTIVITY ANALYSIS?
- Log in to post comments
Functional connectivity analysis by detecting neuronal coactivation in the brain can be efficiently done using Resting State Functional Magnetic Resonance Imaging (rs-fMRI) analysis. Most of the existing research in this area employ correlation-based group averaging strategies of spatial smoothing and temporal normalization of fMRI scans, whose reliability of results heavily depends on the voxel resolution of fMRI scan as well as scanning duration. Scanning period from 5 to 11 minutes has been chosen by most of the studies while estimating the connectivity of brain networks.
- Categories:
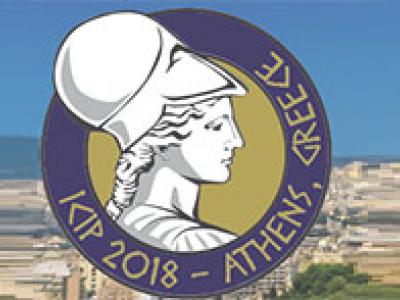
- Read more about SEGMENTATION OF LUNG TUMOR IN CONE BEAM CT IMAGES BASED ON LEVEL-SETS
- Log in to post comments
poster_v2.pdf

- Categories: